Don’t Expect AI Magic. Your Data Still Matters Most
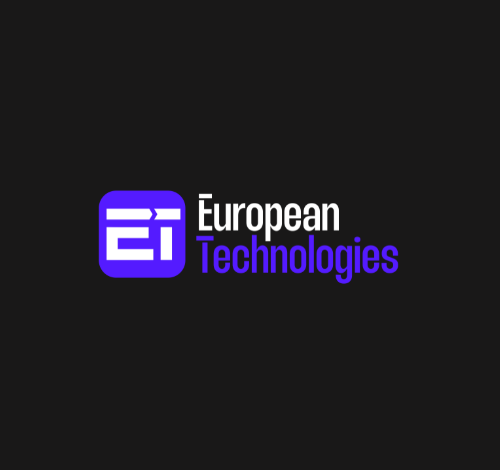

For many years, financial institutions have looked to AI to drive innovation and efficiency. More than just a buzzword, AI has helped many reshape their value proposition, streamline processes, increase accuracy, and tease out insights many thought none were in the past.
Recently, machine learning (ML) algorithms have become the main focus within the broader AI space, led by the pervasive generative AI.
CDOTrends caught up with Naeem Siddiqi, senior advisor for risk and quantitative solutions at SAS, at the sidelines of a financial services conference in Bangkok and discovered what he had to say about AI’s role in finance.
The AI evolution: From hype to value
Siddiqi dispels the notion of sudden, groundbreaking shifts. Instead, he underscores a gradual evolution within the industry.
Obviously, the hype surrounding AI/ML in its early days has subsided, as banks are finding practical use cases for the technology, particularly with complex data sets where they see better results.
Below are some major use cases Siddiqi cites:
- Knowledge Discovery: ML helps uncover patterns in data that are too complex for traditional methods, such as income estimation and cash shortfall.
- Income Estimation: Lenders in Turkey are among the first to adopt ML lending fully. However, Siddiqi notes that this use case is unique as “most regulators and validation folks are a bit hesitant about using these models to lend money.”
- Fraud and Risk Assessment: Transactional data is being leveraged to improve behavioral models.
“Machine learning models are better at modeling the relationship between thousands of factors than something like logistic regression on the other side,” says Siddiqi.
Another major application is using LLMs to sift through “400-page ESG reports.”
The data quality imperative
Siddiqi strikes a balance between enthusiasm about AI’s potential and a clear understanding of the challenges.
While many promising use cases exist, it’s clear that ethical considerations, data quality, and compliance will remain central to successful AI integration within the finance sector.
First and foremost, the success of many ML models depends entirely on the quality of the data they’re trained on.
Siddiqi warns companies not to expect magic from poorly managed or low-quality data. The best results will come from well-governed and maintained data sources.
Financial institutions that invest in robust data infrastructure will reap the most significant benefits.
Yet, everything boils down to data quality. “I like to tell people AI is not magic,” says Siddiqi.
Navigating complex data and regulations
Siddiqi acknowledges the complexity of working with datasets from multiple sources.
He highlights that many large banks continue to face issues with legacy systems and integrating disparate data. “Endless data warehouse” projects often leave these banks with massive, unwieldy data sets.
Silos compound this challenge. Data ownership and resistance against data sharing can make holistic decision-making difficult.
Siddiqi admits neo-banks or digital banks have an edge. “They are better off because they started with modern infrastructure. Some of the larger financial institutions have systems from the 1970s.”
Yet, Siddiqi feels financial institutions are beginning to take data modernization more seriously. The more successful banks are developing digital silos with modern infrastructure for new initiatives. This streamlines AI applications and helps avoid the pitfalls of endless integration projects.
There’s also a push for greater integration. One such effort is bringing together AML (anti-money laundering), fraud, and risk management.
When discussing compliance credit risk and risk management within the bank, the conversation on the AML side was very separate. However, with the integration of different platforms, there is a chance for more holistic customer management and decision-making.
Siddiqi sees this integration as a positive trend, although still in its very early stages.
Potential areas of concern
Siddiqi acknowledges the challenge of AI compliance, mainly when dealing with data from multiple jurisdictions.
Global standardization of AI regulations is unlikely, so banks must balance local compliance with a global perspective.
Recently, explainability has become a significant area of concern for financial institutions. Regulations like the E.U. AI Act, which focus on use cases, make explainability vital.
Siddiqi noted that all financial institutions face challenges in AI explainability and data bias when working with ML models. “Banks have biased data because origination data is inherently biased.”
While techniques like the reject inference and Shapley value help to fix bias and interpret specific models, there are still concerns about using ML in high-risk use cases.
“All of these issues are really about confidence, and no number can define what that is,” says Siddiqi.
Numbers also won’t help financial institutions manage jurisdictional nuances and evolving interpretations of laws. The Court of Justice of the European Union’s issue of a landmark judgment on Article 22 of the General Data Protection Regulation (GDPR) is one good example. It found that the credit scoring system that SCHUFA (German credit reference agency) deployed was, in principle, contrary to the GDPR as it was as as an “automated individual decision.”
Equally concerning is causality. Correlations found within large datasets may be questionable and potentially unethical. Siddiqi stresses the importance of human oversight in AI processes for ethical use and smart analytics.
These are hard questions, but questions that banks need to grapple with, says Siddiqi.
Synthetic data: A limited role
A key topic these days surrounds the use of synthetic data. Synthetic data is artificially generated data that closely mirrors real-world data’s statistical properties and patterns. It offers realistic information without directly using sensitive customer data, making it a valuable asset in various applications.
While many have suggested using synthetic data in credit risk assessment and loan underwriting, Siddiqi is not overly concerned. He sees ample availability of alternative data (cell phone data, Netflix data, etc.), making synthetic data less necessary.
Synthetic data may be helpful in low-incidence areas like fraud detection, but it’s a mixed bag. It still needs to be integrated tightly with data pipelines and modeling processes, and it may even exacerbate bias found in real-world data.
AI in finance: The long, curvy road ahead
In the end, Siddiqi believes that no amount of AI will fix bad data, although it will make efficient use of what you have.
He sees the most impactful AI projects in finance coming from institutions that take a ‘start small’ approach, focusing on tangible goals and specific issues rather than sweeping overhauls. These will be the ones that successfully combine strong data management, strategic modernization, and targeted AI deployment.
Clearly, the ethical challenges of AI in finance are far from solved. Responsible AI demands careful consideration at every stage, from black-box models to the risk of hidden bias in vast data sets. Financial institutions that navigate these complexities must balance human judgment with AI-powered insights.
Siddiqi’s call for a “smart analytics culture” highlights this need: the true power of AI in finance lies not in automation but in enhancing human expertise.
Image credit: iStockphoto/FOTOKITA