Emerging paradigms in microwave imaging technology for biomedical applications: unleashing the power of artificial intelligence
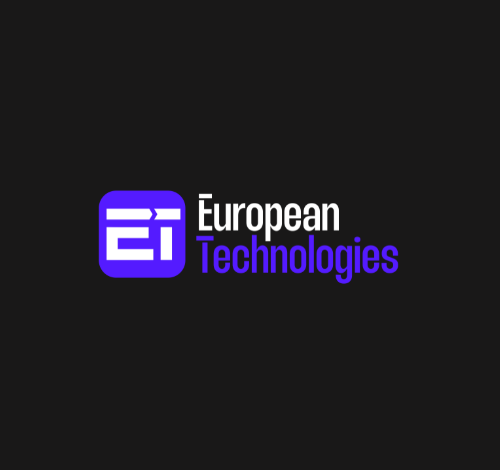
Sharma, P., Jain, S., Gupta, S. & Chamola, V. Role of machine learning and deep learning in securing 5g-driven industrial iot applications. Ad Hoc Netw. 123, 102685 (2021).
Shaheen, M. Y. Applications of artificial intelligence (ai) in healthcare: A review. ScienceOpen Preprints 1–8, https://doi.org/10.14293/S2199-1006.1.SOR-.PPVRY8K.v1 (2021).
Johnson, K. B. et al. Precision medicine, ai, and the future of personalized health care. Clin. Transl. Sci. 14, 86–93 (2021).
Janjic, A., Akduman, I., Cayoren, M., Bugdayci, O. & Aribal, M. E. Safe-microwave imaging device for breast cancer early screening and diagnostics. In Electromagnetic Imaging for a Novel Generation of Medical Devices: Fundamental Issues, Methodological Challenges and Practical Implementation, 273–292 (Springer, 2023).
Yago Ruiz, Á., Cavagnaro, M. & Crocco, L. An effective framework for deep-learning-enhanced quantitative microwave imaging and its potential for medical applications. Sensors 23, 643 (2023).
Wang, M., Scapaticci, R., Cavagnaro, M. & Crocco, L. Towards a microwave imaging system for continuous monitoring of liver tumor ablation: Design and in silico validation of an experimental setup. Diagnostics 11, 866 (2021).
Eini Keleshteri, M. et al. Demonstration of quantitative microwave imaging using an ideal veselago lens. IEEE Open J. Antennas Propag. 3, 1324–1340 (2022).
Fazli, R. & Momeni, H. Microwave imaging of small scatterers by music algorithm using a novel source number detection method. Progr. Electromagn. Res. C 127, 145–156 (2022).
Benedetti, M. et al. An innovative microwave-imaging technique for nondestructive evaluation: Applications to civil structures monitoring and biological bodies inspection. IEEE Trans. Instrum. Measur. 55, 1878–1884 (2006).
Kazemi, F., Mohanna, F. & Ahmadi-Shokouh, J. Nondestructive high-resolution microwave imaging of biomaterials and biological tissues. AEU Int. J. Electronics Commun. 84, 177–185 (2018).
Haynes, M., Stang, J. & Moghaddam, M. Real-time microwave imaging of differential temperature for thermal therapy monitoring. IEEE Trans. Biomed. Eng. 61, 1787–1797 (2014).
Lu, P. Development of Novel Algorithms for Microwave Medical Imaging Applications. Ph.D. thesis (King’s College London, 2023).
Lalitha, K. & Manjula, J. Non-invasive microwave head imaging to detect tumors and to estimate their size and location. Phys. Med. 13, 100047 (2022).
Wu, M., Shu, J. et al. Multimodal molecular imaging: current status and future directions. Contrast Media Mol. Imaging 2018, 1382183 (2018).
Mukherjee, S., Su, Z., Udpa, L., Udpa, S. & Tamburrino, A. Enhancement of microwave imaging using a metamaterial lens. IEEE Sens. J. 19, 4962–4971 (2019).
Di Donato, L. & Morabito, A. Microwave Imaging and Electromagnetic Inverse Scattering Problems (MDPI Basel, Switzerland, 2020).
Kumar, P., Chauhan, S. & Awasthi, L. K. Artificial intelligence in healthcare: review, ethics, trust challenges & future research directions. Eng. Appl. Artif. Intell. 120, 105894 (2023).
Shao, W. & Du, Y. Microwave imaging by deep learning network: Feasibility and training method. IEEE Trans. Antennas Propag. 68, 5626–5635 (2020).
Rekanos, I. T. Neural-network-based inverse-scattering technique for online microwave medical imaging. IEEE Trans. Magn. 38, 1061–1064 (2002).
Hu, X., Xu, F., Guo, Y., Feng, W. & Jin, Y.-Q. Mdli-net: Model-driven learning imaging network for high-resolution microwave imaging with large rotating angle and sparse sampling. IEEE Trans. Geosci. Remote Sens. 60, 1–17 (2022).
Reimer, T. & Pistorius, S. The diagnostic performance of machine learning in breast microwave sensing on an experimental dataset. IEEE J. Electromagn. RF Microwaves Med. Biol. 6, 139–145 (2021).
Levshinskii, V. et al. Application of data mining and machine learning in microwave radiometry (mwr). In Biomedical Engineering Systems and Technologies: 12th International Joint Conference, BIOSTEC 2019, Prague, Czech Republic, February 22–24, 2019, Revised Selected Papers 12, 265–288 (Springer, 2020).
BİÇER, M. B., ELİİYİ, U. & ELİİYİ, D. T. Deep learning-based classification of breast tumors using raw microwave imaging data. Politeknik Dergisi https://doi.org/10.2339/politeknik.1056839 (2023).
Muhammad, S. N., Isa, M. M. & Jamlos, F. Review article of microwave imaging techniques and dielectric properties for lung tumor detection. In AIP conference proceedings, vol. 2203, 020012 (AIP Publishing LLC, 2020).
Aldhaeebi, M. A. et al. Review of microwaves techniques for breast cancer detection. Sensors 20, 2390 (2020).
Guo, L., Alqadami, A. S. & Abbosh, A. Stroke diagnosis using microwave techniques: Review of systems and algorithms. IEEE J. Electromagn. RF Microwaves Med. Biol. 7, 122–135 (2022).
AlSawaftah, N., El-Abed, S., Dhou, S. & Zakaria, A. Microwave imaging for early breast cancer detection: Current state, challenges, and future directions. J. Imaging 8, 123 (2022).
Goryanin, I. et al. Passive microwave radiometry in biomedical studies. Drug Discov. Today 25, 757–763 (2020).
Semenov, S. Microwave tomography: review of the progress towards clinical applications. Philos. Trans. R. Soc. A 367, 3021–3042 (2009).
Kwon, S. & Lee, S. Recent advances in microwave imaging for breast cancer detection. Int. J. Biomed. Imaging 2016, 5054912 (2016).
Wang, L. Early diagnosis of breast cancer. Sensors 17, 1572 (2017).
Hagness, S. C., Taflove, A. & Bridges, J. E. Two-dimensional fdtd analysis of a pulsed microwave confocal system for breast cancer detection: Fixed-focus and antenna-array sensors. IEEE Trans. Biomed. Eng. 45, 1470–1479 (1998).
Klemm, M., Craddock, I., Leendertz, J., Preece, A. & Benjamin, R. Experimental and clinical results of breast cancer detection using uwb microwave radar. In 2008 IEEE Antennas and Propagation Society International Symposium, 1–4 (IEEE, 2008).
Klemm, M., Craddock, I. J., Leendertz, J. A., Preece, A. & Benjamin, R. Radar-based breast cancer detection using a hemispherical antenna array-experimental results. IEEE Trans. Antennas Propag. 57, 1692–1704 (2009).
Henriksson, T. et al. Clinical trials of a multistatic uwb radar for breast imaging. In 2011 Loughborough Antennas & Propagation Conference, 1–4 (IEEE, 2011).
Fear, E. & Sill, J. Preliminary investigations of tissue sensing adaptive radar for breast tumor detection. In Proceedings of the 25th Annual International Conference of the IEEE Engineering in Medicine and Biology Society (IEEE Cat. No. 03CH37439), vol. 4, 3787–3790 (IEEE, 2003).
Williams, T. C., Fear, E. C. & Westwick, D. T. Tissue sensing adaptive radar for breast cancer detection-investigations of an improved skin-sensing method. IEEE Trans. Microwave Theory Tech. 54, 1308–1314 (2006).
Bond, E. J., Li, X., Hagness, S. C. & Van Veen, B. D. Microwave imaging via space-time beamforming for early detection of breast cancer. IEEE Trans. Antennas Propag. 51, 1690–1705 (2003).
Elsdon, M., Leach, M., Skobelev, S. & Smith, D. Microwave holographic imaging of breast cancer. In 2007 International Symposium on Microwave, Antenna, Propagation and EMC Technologies for Wireless Communications (IEEE, 2007). https://doi.org/10.1109/mape.2007.4393791.
Larsen, L. E. & Jacobi, J. H. Microwave scattering parameter imagery of an isolated canine kidney. Med. Phys. 6, 394–403 (1979).
Rosen, B. R.Microwave thermography for the detection of breast cancer a discussion and evaluation of a 6 GHz system. Ph.D. thesis, (Massachusetts Institute of Technology, 1980).
Larsen, L. E. & Jacobi, J. H. Methods of microwave imagery for diagnostic applications. In Diagnostic Imaging in Medicine 68–123 (Springer, 1983).
Larsen, L. E., Jacobi, J. H. et al. Medical applications of microwave imaging, vol. 32 (IEEE press New York, 1986).
Hawley, M., Broquetas, A., Jofre, L., Bolomey, J. C. & Gaboriaud, G. Microwave imaging of tissue blood content changes. J. Biomed. Eng. 13, 197–202 (1991).
Meaney, P. M., Paulsen, K. D., Hartov, A. & Crane, R. Microwave imaging for tissue assessment: Initial evaluation in multitarget tissue-equivalent phantoms. IEEE Trans. Biomed. Eng. 43, 878–890 (1996).
Hagness, S. C., Taflove, A. & Bridges, J. E. Three-dimensional fdtd analysis of a pulsed microwave confocal system for breast cancer detection: Design of an antenna-array element. IEEE Trans. Antennas Propag. 47, 783–791 (1999).
Preece, A., Green, J., Pothecary, N. & Johnson, R. Microwave imaging for tumour detection. In IEE Colloquium on Radar and Microwave Imaging, 9–1 (IET, 1994).
Fear, E. C. & Stuchly, M. A. Microwave system for breast tumor detection. IEEE Microwave Guided Wave Lett. 9, 470–472 (1999).
Meaney, P. M., Paulsen, K., Chang, J. & Fanning, M. Initial microwave imaging experiments in ex-vivo breast tissue. In Proceedings of the First Joint BMES/EMBS Conference. 1999 IEEE Engineering in Medicine and Biology 21st Annual Conference and the 1999 Annual Fall Meeting of the Biomedical Engineering Society (Cat. N, vol. 2, 1130–vol (IEEE, 1999).
Bolomey, J. C., Pichot, C. & Garboriaud, G. Planar microwave imaging camera for biomedical applications: Critical and prospective analysis of reconstruction algorithms. Radio Sci. 26, 541–549 (1991).
Jofre, L. et al. Medical imaging with a microwave tomographic scanner. IEEE Trans. Biomed. Eng. 37, 303–312 (1990).
Meaney, P. M., Fanning, M. W., Li, D., Poplack, S. P. & Paulsen, K. D. A clinical prototype for active microwave imaging of the breast. IEEE Trans. Microwave Theory Tech. 48, 1841–1853 (2000).
Fear, E., Li, X., Hagness, S. & Stuchly, M. Confocal microwave imaging for breast cancer detection: localization of tumors in three dimensions. IEEE Trans. Biomed. Eng. 49, 812–822 (2002).
Li, X., Davis, S., Hagness, S., van der Weide, D. & Van Veen, B. Microwave imaging via space-time beamforming: experimental investigation of tumor detection in multilayer breast phantoms. IEEE Trans. Microwave Theory Techn. 52, 1856–1865 (2004).
Rubæk, T., Kim, O. S. & Meincke, P. Computational validation of a 3-d microwave imaging system for breast-cancer screening. IEEE Trans. Antennas Propag. 57, 2105–2115 (2009).
Yu, C. et al. Active microwave imaging ii: 3-d system prototype and image reconstruction from experimental data. IEEE Trans. Microwave Theory Tech. 56, 991–1000 (2008).
Semenov, S. Y., Svenson, R. H. & Tatsis, G. P. Microwave spectroscopy of myocardial ischemia and infarction. 1. experimental study. Ann. Biomed. Eng. 28, 48–54 (2000).
Semenov, S. Y. et al. Dielectrical spectroscopy of canine myocardium during acute ischemia and hypoxia at frequency spectrum from 100 khz to 6 ghz. IEEE Trans. Med. Imaging 21, 703–707 (2002).
Semenov, S. Y. et al. Microwave tomography for detection/imaging of myocardial infarction. i. excised canine hearts. Ann. Biomed. Eng. 31, 262–270 (2003).
Semenov, S. Y. et al. Development of microwave tomography for functional cardiac imaging. In 2004 2nd IEEE International Symposium on Biomedical Imaging: Nano to Macro (IEEE Cat No. 04EX821), 1351–1353 (IEEE, 2004).
Zhang, Z. Q. & Liu, Q. H. Three-dimensional nonlinear image reconstruction for microwave biomedical imaging. IEEE Trans. Biomed. Eng. 51, 544–548 (2004).
Golnabi, A. H., Meaney, P. M., Geimer, S., Zhou, T. & Paulsen, K. D. Microwave tomography for bone imaging. In 2011 IEEE International Symposium on Biomedical Imaging: From Nano to Macro, 956–959 (IEEE, 2011).
Meaney, P. M. et al. 3d microwave bone imaging. In 2012 6th European Conference on Antennas and Propagation (EUCAP), 1770–1771 (IEEE, 2012).
Bisio, I. et al. Brain stroke microwave imaging by means of a newton-conjugate-gradient method in lp banach spaces. IEEE Trans. Microwave Theory Tech. 66, 3668–3682 (2018).
Meaney, P. M. et al. Microwave imaging for neoadjuvant chemotherapy monitoring: Initial clinical experience. Breast Cancer Res. 15, 1–16 (2013).
Scapaticci, R., Bucci, O., Catapano, I., Crocco, L. et al. Differential microwave imaging for brain stroke followup. Int. J. Antennas Propag. 2014, 312528 (2014).
Zamani, A., Rezaeieh, S. & Abbosh, A. Lung cancer detection using frequency-domain microwave imaging. Electronics Lett. 51, 740–741 (2015).
Babarinde, O., Jamlos, M., Soh, P., Schreurs, D.-P. & Beyer, A. Microwave imaging technique for lung tumour detection. In 2016 German Microwave Conference (GeMiC), 100–103 (IEEE, 2016).
Gilmore, C., Zakaria, A., Pistorius, S. & LoVetri, J. Microwave imaging of human forearms: Pilot study and image enhancement. J. Biomed. Imaging 2013, 19–19 (2013).
Islam, M., Mahmud, M., Islam, M. T., Kibria, S. & Samsuzzaman, M. A low cost and portable microwave imaging system for breast tumor detection using uwb directional antenna array. Sci. Rep. 9, 15491 (2019).
Janjic, A. et al. Safe: A novel microwave imaging system design for breast cancer screening and early detection-clinical evaluation. Diagnostics 11, 533 (2021).
Moloney, B. M. et al. Microwave imaging in breast cancer–results from the first-in-human clinical investigation of the wavelia system. Acad. Radiol. 29, S211–S222 (2022).
Santos, K. C., Fernandes, C. A. & Costa, J. R. Feasibility of bone fracture detection using microwave imaging. IEEE Open J. Antennas Propag. 3, 836–847 (2022).
Rodriguez-Duarte, D. O. et al. Experimental assessment of real-time brain stroke monitoring via a microwave imaging scanner. IEEE Open J. Antennas Propag. 3, 824–835 (2022).
Rodriguez-Duarte, D. O. et al. Towards a microwave imaging device for cerebrovascular diseases monitoring: from numerical modeling to experimental testing. In Electromagnetic Imaging for a Novel Generation of Medical Devices: Fundamental Issues, Methodological Challenges and Practical Implementation, 203–233 (Springer, 2023).
Santos, K. C., Fernandes, C. A. & Costa, J. R. Validation of a compact microwave imaging system for bone fracture detection. IEEE Access 11, 63690–63700 (2023).
Alkhodari, M., Zakaria, A. & Qaddoumi, N. Monitoring bone density using microwave tomography of human legs: a numerical feasibility study. Sensors 21, 7078 (2021).
Laskari, K. et al. Joint microwave radiometry for inflammatory arthritis assessment. Rheumatology 59, 839–844 (2020).
Owda, A. Y. & Owda, M. Early detection of skin disorders and diseases using radiometry. Diagnostics 12, 2117 (2022).
Amin, B., Shahzad, A., O’halloran, M., Mcdermott, B. & Elahi, A. Experimental validation of microwave imaging prototype and dbim-imatcs algorithm for bone health monitoring. IEEE Access 10, 42589–42600 (2022).
Henriksson, T. et al. Human brain imaging by electromagnetic tomography: a mobile brain scanner for clinical settings. In 2022 16th European Conference on Antennas and Propagation (EuCAP), 1–5 (IEEE, 2022).
Goryanin, I., Ovchinnikov, L., Vesnin, S. & Ivanov, Y. Monitoring protein denaturation of egg white using passive microwave radiometry (mwr). Diagnostics 12, 1498 (2022).
Alagee, E. R. & Assalem, A. et al. Brain cancer detection using u-shaped slot vivaldi antenna and confocal radar based microwave imaging algorithm. Am. Acad. Sci. Res. J. Eng. Technol. Sci. 66, 1–13 (2020).
Liu, S., Shang, X., Lu, Y. & Huang, L. Full waveform autofocus inversion based microwave induced transcranial thermoacoustic tomography with a human skull validated. Appl. Phys. Lett. 121, 243702 (2022).
Li, W., Zhang, S., Xing, D. & Qin, H. Pulsed microwave-induced thermoacoustic shockwave for precise glioblastoma therapy with the skin and skull intact. Small 18, e2201342 (2022).
Fedeli, A., Estatico, C., Pastorino, M. & Randazzo, A. Microwave detection of brain injuries by means of a hybrid imaging method. IEEE Open J. Antennas Propag. 1, 513–523 (2020).
Hosseinzadegan, S., Fhager, A., Persson, M., Geimer, S. D. & Meaney, P. M. Discrete dipole approximation-based microwave tomography for fast breast cancer imaging. IEEE Trans. Microwave Theory Tech. 69, 2741–2752 (2021).
Kaur, G. & Kaur, A. Monostatic radar-based microwave imaging of breast tumor using an ultra-wideband dielectric resonator antenna (dra) with a sierpinski fractal defected ground structure. MAPAN 37, 917–928 (2022).
Kaur, G. & Kaur, A. Monostatic radar-based microwave imaging of breast tumor detection using a compact cubical dielectric resonator antenna. Microwave Optical Technol. Lett. 63, 196–204 (2021).
Zhou, S. et al. A preliminary study of ultrasound-guided microwave ablation for non-puerperal mastitis treatment. Breast Care, https://doi.org/10.1159/000527128 (2022).
Rodriguez-Duarte, D., Vasquez, J. T. & Vipiana, F. Hybrid simulation-measurement calibration technique for microwave imaging systems. In 2021 15th European Conference on Antennas and Propagation (EuCAP), 1–5 (IEEE, 2021).
Emilov, B. et al. Diagnostic of patients with covid-19 pneumonia using passive medical microwave radiometry (mwr). Diagnostics 13, 2585 (2023).
Gopalakrishnan, K. et al. Applications of microwaves in medicine leveraging artificial intelligence: Future perspectives. Electronics 12, 1101 (2023).
Panayides, A. S. et al. Ai in medical imaging informatics: current challenges and future directions. IEEE J. Biomed. Health Informatics 24, 1837–1857 (2020).
Khalid, N. et al. Efficient deep learning approaches for automated tumor detection, classification, and localization in experimental microwave breast imaging data. In 2023 IEEE International Symposium on Circuits and Systems (ISCAS), 1–4 (IEEE, 2023).
Shi, F. et al. Review of artificial intelligence techniques in imaging data acquisition, segmentation, and diagnosis for covid-19. IEEE Rev. Biomed. Eng. 14, 4–15 (2020).
Zhou, Z.-H. Machine learning (Springer Nature, 2021).
Zhang, M.-L. & Zhou, Z.-H. Ml-knn: A lazy learning approach to multi-label learning. Pattern Recognit. 40, 2038–2048 (2007).
Likas, A., Vlassis, N. & Verbeek, J. J. The global k-means clustering algorithm. Pattern Recognit. 36, 451–461 (2003).
Salcedo-Sanz, S., Rojo-Álvarez, J. L., Martínez-Ramón, M. & Camps-Valls, G. Support vector machines in engineering: an overview. Wiley Interdiscip. Rev. Data Min. Knowl. Discov. 4, 234–267 (2014).
Navada, A., Ansari, A. N., Patil, S. & Sonkamble, B. A. Overview of use of decision tree algorithms in machine learning. In 2011 IEEE control and system graduate research colloquium, 37–42 (IEEE, 2011).
Liu, Y., Wang, Y. & Zhang, J. New machine learning algorithm: Random forest. In International Conference on Information Computing and Applications, 246–252 (Springer, 2012).
Chen, T. & Guestrin, C. Xgboost: A scalable tree boosting system. In Proceedings of the 22nd ACM SIGKDD international conference on knowledge discovery and data mining, 785–794 (ACM, 2016).
Shrestha, A. & Mahmood, A. Review of deep learning algorithms and architectures. IEEE Access 7, 53040–53065 (2019).
LeCun, Y., Bengio, Y. & Hinton, G. Deep learning. Nature 521, 436–444 (2015).
Albawi, S., Mohammed, T. A. & Al-Zawi, S. Understanding of a convolutional neural network. In 2017 international conference on engineering and technology (ICET), 1–6 (IEEE, 2017).
Pu, Y. et al. Variational autoencoder for deep learning of images, labels and captions. In Advances in neural information processing systems 29 (NeurIPS, 2016).
Hitaj, B., Ateniese, G. & Perez-Cruz, F. Deep models under the gan: information leakage from collaborative deep learning. In Proceedings of the 2017 ACM SIGSAC conference on computer and communications security, 603–618 (ACM, 2017).
Veličković, P. et al. Graph attention networks. Preprint at https://arxiv.org/abs/1710.10903 (2017).
Wang, Y. & Gong, X. A neural network approach to microwave imaging. Int. J. Imaging Syst. Technol. 11, 159–163 (2000).
Kerhet, A., Raffetto, M., Boni, A. & Massa, A. A svm-based approach to microwave breast cancer detection. Eng. Appl. Artif. Intell. 19, 807–818 (2006).
Woten, D. Artificial neural networks for breast cancer detection using micro antennas (University of Arkansas, 2007).
Abbosh, Y. M., Yahya, A. F. & Abbosh, A. Neural networks for the detection and localization of breast cancer. In 2011 International Conference on Communications and Information Technology (ICCIT), 156–159 (IEEE, 2011).
Yahya, A. F., Abbosh, Y. M. & Abbosh, A. Microwave imaging method employing wavelet transform and neural networks for breast cancer detection. In Asia-Pacific Microwave Conference 2011, 1418–1421 (IEEE, 2011).
Hahn, C. & Noghanian, S. Heterogeneous breast phantom development for microwave imaging using regression models. J. Biomed. Imaging 2012, 6–6 (2012).
Persson, M. et al. Microwave-based stroke diagnosis making global prehospital thrombolytic treatment possible. IEEE Trans. Biomed. Eng. 61, 2806–2817 (2014).
Al-Hadidi, M. R., Alarabeyyat, A. & Alhanahnah, M. Breast cancer detection using k-nearest neighbor machine learning algorithm. In 2016 9th International Conference on Developments in eSystems Engineering (DeSE), 35–39 (2016).
Gerazov, B. & Conceicao, R. C. Deep learning for tumour classification in homogeneous breast tissue in medical microwave imaging. In IEEE EUROCON 2017 -17th International Conference on Smart Technologies (IEEE, 2017). https://doi.org/10.1109/eurocon.2017.8011175.
Guo, L. & Abbosh, A. Stroke localization and classification using microwave tomography with k-means clustering and support vector machine. Bioelectromagnetics 39, 312–324 (2018).
Shah, P., Chen, G. & Moghaddam, M. Learning nonlinearity of microwave imaging through deep learning. In 2018 IEEE International Symposium on Antennas and Propagation and USNC/URSI National Radio Science Meeting, 699–700 (IEEE, 2018).
Rana, S. P. et al. Machine learning approaches for automated lesion detection in microwave breast imaging clinical data. Sci. Rep. 9, https://doi.org/10.1038/s41598-019-46974-3 (2019).
Levshinskii, V. et al. Using ai and passive medical radiometry for diagnostics (mwr) of venous diseases. Comput. Methods Progr. Biomed. 215, 106611 (2022).
Beyraghi, S. et al. Microwave bone fracture diagnosis using deep neural network. Sci. Rep. 13, 16957 (2023).
Patel, P. & Raina, A. Comparison of machine learning algorithms for tumor detection in breast microwave imaging. In 2021 11th International Conference on Cloud Computing, Data Science Engineering (Confluence) (IEEE, 2021). https://doi.org/10.1109/confluence51648.2021.9377191.
Pelicano, A. C., Araujo, N. A. M. & Conceicao, R. C. Preliminary development of anatomically realistic breast tumor models for microwave imaging. In 2022 16th European Conference on Antennas and Propagation (EuCAP) (IEEE, 2022). https://doi.org/10.23919/eucap53622.2022.9769591.
Conceição, R. C. et al. Classification of breast tumor models with a prototype microwave imaging system. Med. Phys. 47, 1860–1870 (2020).
Webb, G. I., Keogh, E. & Miikkulainen, R. Naïve bayes. Encyclopedia of machine learning 15, 713–714 (2010).
Abdi, H. & Williams, L. J. Principal component analysis. Wiley Interdiscip. Rev. Comput. Stat. 2, 433–459 (2010).
Chen, A., Gu, Y. & Zhang, S. Svm-based microwave breast tumour classification. In 2021 International Conference on Public Health and Data Science (ICPHDS), 174–177 (IEEE, 2021).
Sami, H., Sagheer, M., Riaz, K., Mehmood, M. Q. & Zubair, M. Machine learning-based approaches for breast cancer detection in microwave imaging. In 2021 IEEE USNC-URSI Radio Science Meeting (Joint with AP-S Symposium) (IEEE, 2021). https://doi.org/10.23919/usnc-ursi51813.2021.9703518.
Anguita, D., Ghelardoni, L., Ghio, A., Oneto, L. & Ridella, S. The k’ in k-fold cross validation. In 20th European Symposium on Artificial Neural Networks, Computational Intelligence and Machine Learning (ESANN), 441–446 (i6doc. com publ, 2012).
Ambrosanio, M., Autorino, M. M., Franceschini, S., Baselice, F. & Pascazio, V. Microwave breast imaging via deep learning. In 2022 IEEE 19th International Symposium on Biomedical Imaging (ISBI) (IEEE, 2022). https://doi.org/10.1109/isbi52829.2022.9761470.
Li, J. et al. Dynamic weight agnostic neural networks and medical microwave radiometry (mwr) for breast cancer diagnostics. Diagnostics 12, 2037 (2022).
Zhang, J. et al. Deep-learning-enabled microwave-induced thermoacoustic tomography based on sparse data for breast cancer detection. In IEEE Transactions on Antennas and Propagation (IEEE, 2022).
al Khatib, S. K., Naous, T., Shubair, R. M. & el Misilmani, H. M. A deep learning framework for breast tumor detection and localization from microwave imaging data. In 2021 28th IEEE International Conference on Electronics, Circuits, and Systems (ICECS) (IEEE, 2021). https://doi.org/10.1109/icecs53924.2021.9665521.
Ekblom, E. & Svensson, R. Generative adversarial network for generation of artificial microwave data for stroke detection, Chalmers ODR thesis (Chalmers ODR, 2021).
Gurumurthy, S., Kiran Sarvadevabhatla, R. & Venkatesh Babu, R. Deligan: Generative adversarial networks for diverse and limited data. In Proceedings of the IEEE conference on computer vision and pattern recognition, 166–174 (IEEE, 2017).
Al-Saffar, A., Guo, L. & Abbosh, A. Graph attention network in microwave imaging for anomaly localization. IEEE J. Electromagn. RF Microwaves Med. Biol. 6, 212–218 (2022).
Edwards, K., LoVetri, J., Gilmore, C. & Jeffrey, I. Machine-learning-enabled recovery of prior information from experimental breast microwave imaging data. Progr. Electromagn. Res. 175, 1–11 (2022).
Ambrosanio, M., Franceschini, S., Pascazio, V. & Baselice, F. An end-to-end deep learning approach for quantitative microwave breast imaging in real-time applications. Bioengineering 9, https://www.mdpi.com/2306-5354/9/11/651 (2022).
Ojaroudi, M., Bila, S. & Salimitorkamani, M. A novel machine learning approach of hemorrhage stroke detection in differential microwave head imaging system. In 2020 European Conference on Antennas and Propagation, https://unilim.hal.science/hal-03047739 (2020).
Roohi, M., Mazloum, J., Pourmina, M.-A. & Ghalamkari, B. Machine learning approaches for automated stroke detection, segmentation, and classification in microwave brain imaging systems. Progr. Electromagn. Res. C 116, 193–205 (2021).
Mariano, V., Casu, M. R. & Vipiana, F. Simulation-based machine learning training for brain anomalies localization at microwaves. In 2022 16th European Conference on Antennas and Propagation (EuCAP) (IEEE, 2022). https://doi.org/10.23919/eucap53622.2022.9769504.
Khoshdel, V., Asefi, M., Ashraf, A. & LoVetri, J. Full 3d microwave breast imaging using a deep-learning technique. J. Imaging 6, 80 (2020).
Hossain, A., Islam, M. T. & Almutairi, A. F. A deep learning model to classify and detect brain abnormalities in portable microwave based imaging system. Sci. Rep. 12, https://doi.org/10.1038/s41598-022-10309-6 (2022).
Ninković, D. et al. Deep learning enhanced microwave imaging for brain diagnostics. In 2023 17th European Conference on Antennas and Propagation (EuCAP), 1–4 (IEEE, 2023).
Budach, L. et al. The effects of data quality on machine learning performance. Preprint at https://arxiv.org/abs/2207.14529 (2022).
Wang, L. et al. Microwave breast imaging based on deep learning. In 2022 7th International Conference on Image, Vision and Computing (ICIVC), 749–755 (IEEE, 2022).
Gadepally, R., Gomella, A., Gingold, E. & Lakhani, P. Generalization of artificial intelligence models in medical imaging: A case-based review. Preprint at https://arxiv.org/abs/2211.13230 (2022).
Loh, H. W. et al. Application of explainable artificial intelligence for healthcare: A systematic review of the last decade (2011–2022). Comput. Methods Programs Biomed. 226, 107161 (2022).
Justus, D., Brennan, J., Bonner, S. & McGough, A. S. Predicting the computational cost of deep learning models. In 2018 IEEE international conference on big data (Big Data), 3873–3882 (IEEE, 2018).
Bartoletti, I. Ai in healthcare: Ethical and privacy challenges. In Artificial Intelligence in Medicine: 17th Conference on Artificial Intelligence in Medicine, AIME 2019, Poznan, Poland, June 26–29, 2019, Proceedings 17, 7–10 (Springer, 2019).
Widner, K. et al. Lessons learned from translating ai from development to deployment in healthcare. Nat. Med. 29, 1304–1306 (2023).
Kelly, C. J., Karthikesalingam, A., Suleyman, M., Corrado, G. & King, D. Key challenges for delivering clinical impact with artificial intelligence. BMC Med. 17, 1–9 (2019).
Shujaat, S., Bornstein, M. M., Price, J. B. & Jacobs, R. Integration of imaging modalities in digital dental workflows-possibilities, limitations, and potential future developments. Dentomaxillofacial Radiol. 50, 20210268 (2021).
Li, X. et al. Digital breast tomosynthesis versus digital mammography: integration of image modalities enhances deep learning-based breast mass classification. Eur. Radiol. 30, 778–788 (2020).
Schizas, N., Karras, A., Karras, C. & Sioutas, S. Tinyml for ultra-low power ai and large scale iot deployments: A systematic review. Fut. Internet 14, 363 (2022).
Yang, J., Li, H. B. & Wei, D. The impact of chatgpt and llms on medical imaging stakeholders: perspectives and use cases. Meta Radiol. 1, 100007 (2023).
Mello-Thoms, C. & Mello, C. A. Clinical applications of artificial intelligence in radiology. Brit. J. Radiol. 96, 20221031 (2023).
Liu, P. et al. Impact of deep learning-based optimization algorithm on image quality of low-dose coronary ct angiography with noise reduction: a prospective study. Acad. Radiol. 27, 1241–1248 (2020).
Liu, J. et al. Artificial intelligence-based image enhancement in pet imaging: Noise reduction and resolution enhancement. PET Clin. 16, 553–576 (2021).
Shukla, P. K. et al. Ai-driven novel approach for liver cancer screening and prediction using cascaded fully convolutional neural network. J. Healthc. Eng. 2022, 4277436 (2022).
Wang, C., He, T., Zhou, H., Zhang, Z. & Lee, C. Artificial intelligence enhanced sensors-enabling technologies to next-generation healthcare and biomedical platform. Bioelectronic Med. 9, 17 (2023).
Dack, E. et al. Artificial intelligence and interstitial lung disease: Diagnosis and prognosis. Investig. Radiol. 58, 602–609 (2023).
Saraswat, D. et al. Explainable ai for healthcare 5.0: opportunities and challenges. IEEE Access 10, 84486–84517 (2022).
Stancombe, A. E. & Bialkowski, K. S. Portable biomedical microwave imaging using software-defined radio. In 2018 Asia-Pacific Microwave Conference (APMC), 572–574 (IEEE, 2018).
Khalid, N., Qayyum, A., Bilal, M., Al-Fuqaha, A. & Qadir, J. Privacy-preserving artificial intelligence in healthcare: Techniques and applications. Comput. Biol. Med. 158, 106848 (2023).
Albaaj, A., Norouzi, Y. & Moradi, G. et al. Microwave imaging system of breast cancer detection by using support vector machine method. J. Popul. Therapeutics Clin. Pharmacol. 30, 314–324 (2023).
Nemez, K., Asefi, M., Baran, A. & LoVetri, J. A faceted magnetic field probe resonant chamber for 3d breast mwi: A synthetic study. In 2016 17th International Symposium on Antenna Technology and Applied Electromagnetics (ANTEM), 1–3 (IEEE, 2016).
Song, J., Shen, T. & Wang, Q. An image post-processing approach based on fully dense u-net for microwave induced thermo-acoustic tomography. IEEE J. Electromagn. RF Microwaves Med. Biol. 7, 59–64 (2022).