Ensuring Successful Implementation of Generative AI: What Sets the Winners Apart | by GeoTechInsights | Apr, 2024
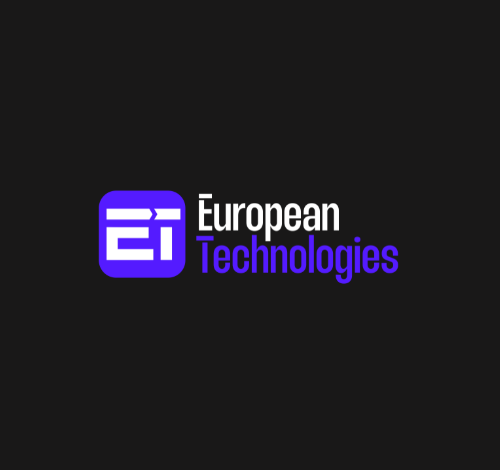
Most companies face the risk of failing to effectively integrate generative AI into their operations. Here is my proposed strategy to avoid being among them.
As developer conferences are starting to feel like a Taylor Swift concert, and programmers have their own version of Swifties for Jensen Huang, NVIDIA’s CEO, the corporate race to harness generative AI has reached a climactic point. Amidst this frenzy, companies are feeling the pressure to integrate state-of-the-art generative AI solutions, driven by the technology’s transformative potential. Yet, this enthusiasm is a double-edged sword.
The tech sector’s current hype masks a critical truth that is often forgotten: tech companies are not just offering innovations; they are selling dreams, with a more important goal of selling products. Amidst this dazzling salesmanship, discerning generative AI’s true and proven capabilities from the hype becomes crucial. Generative AI’s capabilities can be dissected into three distinct layers: the superfluous, the core, and the emerging. The understanding of this tripartite framework is essential for successful implementation of generative AI into money making operations.
My incremental strategy advocates that non-tech companies should harness generative AI’s core capabilities to stretch their operational proficiency, such as enhancing sales processes or improving product development, rather than attempting to stretch the technology’s capabilities into unproven areas. This role of expanding the boundaries of generative AI should be reserved for tech and unicorn companies, who are tasked with exploring and extending its emerging capabilities. By doing so, non-tech companies can optimize their use of generative AI to strengthen their core business functions without overreaching into the technology’s future and unproven potential.
Understanding the distinct capabilities of generative AI — superfluous, core, and emerging — is crucial for its effective implementation.
At the basic level are the superfluous capabilities, where generative AI is employed for tasks that simpler technologies could easily handle, this is generally a forceful integration just for show. For example, using generative AI for basic keyword searches, a function well within the reach of traditional search algorithms or basic natural language processing (NLP), such as filtering emails by keywords.
The emerging capabilities represent the most advanced and experimental aspects of generative AI. These include its ability to perform tasks requiring high levels of human reasoning and specialized multimodal domain specific knowledge; its use for medical diagnostics would fall under this category. They embody the frontier of AI technology, offering innovative applications yet fraught with risks and implementation challenges. These are promised and not proven applications of the technology and the attempted implementation of these capabilities are costly, risky, and inefficient for corporations.
The core capabilities, however, are where generative AI truly shines. These include advanced natural language understanding, sophisticated language generation, and dynamic content creation. These strengths enable it to autonomously draft coherent and contextually relevant texts, such as reports or narratives, and interactively summarize or extract key information from documents, marking a significant enhancement over traditional methods. These capabilities are similar to what a human with little to no training can perform in any given domain.
Effective real-world applications of generative AI hinge on focusing on its core strengths, particularly in language processing. Misjudging these categories can lead to significant waste of resources and potential failure.
When companies rush to adopt the emerging capabilities of generative AI, they often exceed their grasp, courting failure. This approach can erode internal confidence in AI systems, drain financial resources, and lead to the loss of critical opportunities. It’s akin to hastily constructing a bridge without ensuring stability: while it might appear to advance the company’s technological edge, the inherent instability risks a catastrophic collapse, potentially setting back the organization by years.
Consider the misguided effort to harness generative AI for human-like reasoning, a capability beyond its current core functions. Such attempts typically yield subpar results, undermining user trust and setting a negative precedent for the technology’s future use within the company. Not only does this fail to provide a return on investment, but it also leaves the company without a proper foundation for further AI integration.
The incremental strategy’s initial step is for the business and engineering units to identify practical use cases where generative AI’s current core capabilities can be applied. The business unit should bring forward unresolved inefficiencies in their systems which were blocked by prior technological limitations. The engineering team should then identify if the solutions to those problems fall under generative AI’s current core capabilities. This is generally a back and forth process that requires lots of collaborative work. The engineering team will learn about the business and the business team will learn about the technology and its limitations.
After the identification stage comes the implementation. This will require a lot of backend work for the engineering team to find alignment between hardware, software, and its workforce allowing for the productionization of this new technology. This means new frameworks, powerful hardware infrastructure, and upskilling its engineers, making the first use case the longest to complete.
Once these initial projects become self-sustaining through generated profits and the engineering team has built the tools and implemented the infrastructure required for this new technology, enough time will have passed for to the point where the boundary of what is considered emerging today gradually shifts to become the core capability of tomorrow, driven by advancements from tech giants like Meta, Google, and OpenAI.
The corporation using this method can now more easily implement the newly transitioned core capabilities to extend its existing use cases for generative AI. They will now be able to do so more quickly thanks to the accumulation of tools and knowledge from its first round of industry applications. This will create a cycle of rinse and repeat.
The incremental strategy promises that by focusing on implementing only the core capabilities of generative AI, the corporation will build the solid foundation required to implement future advancements more rapidly with each iteration. This reinforces the partnership between business and engineering teams while fostering an environment where trust in AI solutions multiplies alongside tangible, industry-specific enhancements.
To maximize the benefits of this approach, companies should not view smaller incremental projects as just stepping stones to a grander future vision of generative AI application. Instead, these projects should be valued as significant achievements in their own right. Treating them merely as means to an end undermines the gradual building of the company’s innovative capacity; and signifies a deviation from a true incremental strategy. This risks premature scaling beyond the company’s capabilities, leading to potential failure. Furthermore, these smaller, incremental projects will generally address long-standing inefficiencies within the company which exist because of prior technological limitations. Seizing these opportunities to make minor adjustments to existing workflows, while seemingly small, can massively impact a company’s productivity. Neglecting these changes risks missing out on the substantial benefits of proven generative AI capabilities.
If a corporation decides to invest resources in exploring emerging capabilities, these projects should primarily be viewed as educational ventures with no set application expectations, consuming only a small portion of the total resources dedicated to generative AI initiatives. These exploratory projects should be managed distinctively, with every action, decision, and outcome — both failures and successes — meticulously recorded and analyzed. This systematic documentation serves as a valuable learning tool, aiding the organization in recognizing the exact moment a capability transitions from emerging to core. This ensures that a functionality is well-understood and established as a core capability before being fully integrated into the incremental strategy.
I am not implying that machine learning engineers operating at non-tech companies should not strive to innovate. Engineers working in tech companies should create innovative technologies and focus on stretching these technologies’ emerging capabilities and translating them into core functionalities. Engineers in non-tech companies should use these innovative technologies at their true and tested level to create innovative applications of these technologies for their companies industry specific needs. Both are doing innovative work but what they are innovating is different.