Estimating the energy consumption for residential buildings in semiarid and arid desert climate using artificial intelligence
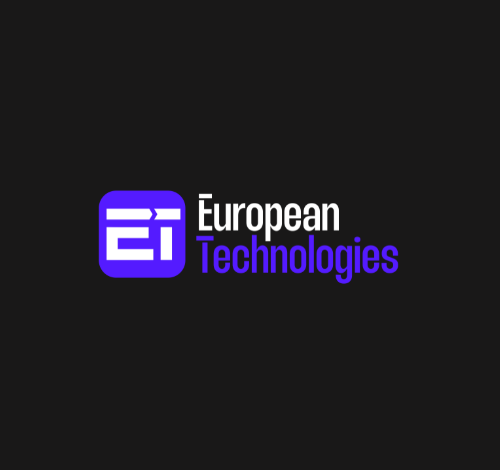
Elbeltagi, E., Wefki, H. & Khallaf, R. Sustainable building optimization model for early-stage design. Buildings 13(1), 74 (2022).
Das, S., Swetapadma, A., Panigrahi, C. & Abdelaziz, A. Y. Improved method for approximation of heating and cooling load in urban buildings for energy performance enhancement. Electr. Power Comp. Syst. 48(4–5), 436–446 (2020).
El-Sayed, A. H. A., Khalil, A. & Yehia, M. Modeling alternative scenarios for Egypt 2050 energy mix based on LEAP analysis. Energy 266, 126615 (2023).
Seyedzadeh, S., Rahimian, F. P., Glesk, I. & Roper, M. Machine learning for estimation of building energy consumption and performance: A review. Visual. Eng. 6, 1–20 (2018).
IEA International Energy Agency. Key World Energy Statistics (IEA, 2015).
Swan, L. G. & Ugursal, V. I. Modeling of end-use energy consumption in the residential sector: A review of modeling techniques. Renew. Sustain. Energy Rev. 13, 1819–1835 (2009).
Mui, K. W., Satheesan, M. K. & Wong, L. T. Building cooling energy consumption prediction with a hybrid simulation Approach: Generalization beyond the training range. Energy Build. 276, 112502 (2022).
Al-Shargabi, A. A., Almhafdy, A., Ibrahim, D. M., Alghieth, M. & Chiclana, F. Buildings’ energy consumption prediction models based on buildings’ characteristics: Research trends, taxonomy, and performance measures. J. Build. Eng. 54, 104577 (2022).
Ilbeigi, M., Ghomeishi, M. & Dehghanbanadaki, A. Prediction and optimization of energy consumption in an office building using artificial neural network and a genetic algorithm. Sustain. Cities Soc. 61, 102325 (2020).
Amasyali, K. & El-Gohary, N. M. A review of data-driven building energy consumption prediction studies. Renew. Sustain. Energy Rev. 81, 1192–1205 (2018).
Mansour, D. M. & Ebid, A. M. Modeling of heat transfer in massive concrete foundations using 3D-FDM. Civ. Eng. J. 9(10), 2430–2444 (2023).
Mansour, D. M. & Ebid, A. M. Predicting thermal behavior of mass concrete elements using 3D finite difference model. Asian J. Civ. Eng. 25(2), 1601–1611 (2024).
Elbeltagi, E., Wefki, H., Abdrabou, S., Dawood, M. & Ramzy, A. Visualized strategy for predicting buildings energy consumption during early design stage using parametric analysis. J. Build. Eng. 13, 127–136 (2017).
Welle, B., Haymaker, J. & Rogers, Z. ThermalOpt: A methodology for automated BIM-based multidisciplinary thermal simulation for use in optimization environments. Build. Simul. 4, 293–313 (2013).
Biswas, M. R., Robinson, M. D. & Fumo, N. Prediction of residential building energy consumption: A neural network approach. Energy 117, 84–92 (2016).
Castelli, M., Trujillo, L., Vanneschi, L. & Popovič, A. Prediction of energy performance of residential buildings: A genetic programming approach. Energy Build. 102, 67–74. https://doi.org/10.1016/j.enbuild.2015.05.01310.1016/j.enbuild.2015.05.013 (2015).
Tahmassebi, A. & Gandomi, A. H. Building energy consumption forecast using multi-objective genetic programming. Measurement 118, 164–171 (2018).
Mat Daut, M. A. et al. Building electrical energy consumption forecasting analysis using conventional and artificial intelligence methods: A review. Renew. Sustain. Energy Rev. 70, 1108–1118. https://doi.org/10.1016/j.rser.2016.12.015 (2017).
Yazici, I., Beyca, O. F. & Delen, D. Deep-learning-based short-term electricity load forecasting: A real case application. Eng. Appl. Artif. Intell. 109, 104645 (2022).
Jamei, M. et al. Estimating the density of hybrid nanofluids for thermal energy application: Application of non-parametric and evolutionary polynomial regression data-intelligent techniques. Measurement 189, 110524 (2022).
Yin, Z.-Y. & Jin, Y.-F. Optimization-based evolutionary polynomial regression. Pract. Optim. Theory Geotech. Eng. https://doi.org/10.1007/978-981-13-3408-5_5 (2019).
Khan, S. U. et al. Towards intelligent building energy management: AI-based framework for power consumption and generation forecasting. Energy Build. 279, 112705 (2023).
Runge, J. & Saloux, E. A comparison of prediction and forecasting artificial intelligence models to estimate the future energy demand in a district heating system. Energy 269, 126661 (2023).
Olu-Ajayi, R., Alaka, H., Sulaimon, I., Sunmola, F. & Ajayi, S. Building energy consumption prediction for residential buildings using deep learning and other machine learning techniques. J. Build. Eng. 45, 103406 (2022).
Yang, W. et al. A combined deep learning load forecasting model of single household resident user considering multi-time scale electricity consumption behavior. Appl. Energy 307, 118197 (2022).
Li, X. & Yao, R. Modelling heating and cooling energy demand for building stock using a hybrid approach. Energy Build. 235, 110740 (2021).
Zou, Y., Xiang, K., Zhan, Q. & Li, Z. A simulation-based method to predict the life cycle energy performance of residential buildings in different climate zones of China. Build. Environ. 193, 107663 (2021).
D’Amico, A., Ciulla, G., Traverso, M., Brano, V. L. & Palumbo, E. Artificial Neural Networks to assess energy and environmental performance of buildings: An Italian case study. J. Clean. Prod. 239, 117993 (2019).
Mohammadi, M., Talebpour, F., Safaee, E., Ghadimi, N. & Abedinia, O. Small-scale building load forecast based on hybrid forecast engine. Neural Process. Lett. 48, 329–351 (2018).
Ullah, I., Ahmad, R. & Kim, D. A prediction mechanism of energy consumption in residential buildings using hidden Markov model. Energies 11(2), 358 (2018).
Fayaz, M. & Kim, D. A prediction methodology of energy consumption based on deep extreme learning machine and comparative analysis in residential buildings. Electronics 7(10), 222 (2018).
Ascione, F., Bianco, N., De Stasio, C., Mauro, G. M. & Vanoli, G. P. Artificial neural networks to predict energy performance and retrofit scenarios for any member of a building category: A novel approach. Energy 118, 999–1017 (2017).
Mocanu, E., Nguyen, P. H., Gibescu, M. & Kling, W. L. Deep learning for estimating building energy consumption. Sustain. Energy Grids Netw. 6, 91–99 (2016).
Samuelson, H., Claussnitzer, S., Goyal, A., Chen, Y. & Romo-Castillo, A. Parametric energy simulation in early design: High-rise residential buildings in urban contexts. Build. Environ. 101, 19–31 (2016).
Fumo, N. & Biswas, M. R. Regression analysis for prediction of residential energy consumption. Renew. Sustain. Energy Rev. 47, 332–343 (2015).
Fan, C., Xiao, F. & Wang, S. Development of prediction models for next-day building energy consumption and peak power demand using data mining techniques. Appl. Energy 127, 1–10 (2014).
Elbeltagi, E. & Wefki, H. Predicting energy consumption for residential buildings using ANN through parametric modeling. Energy Rep. 7, 2534–2545 (2021).
Elhabyb, K., Baina, A., Bellafkih, K. & Deifalla, A. F. Machine Learning Algorithms for Predicting Energy Consumption in Educational Buildings. Int. J. Energ. Res. 2024, 1–19. https://doi.org/10.1155/2024/6812425 (2014).