Exploring the Apache ecosystem for data analysis
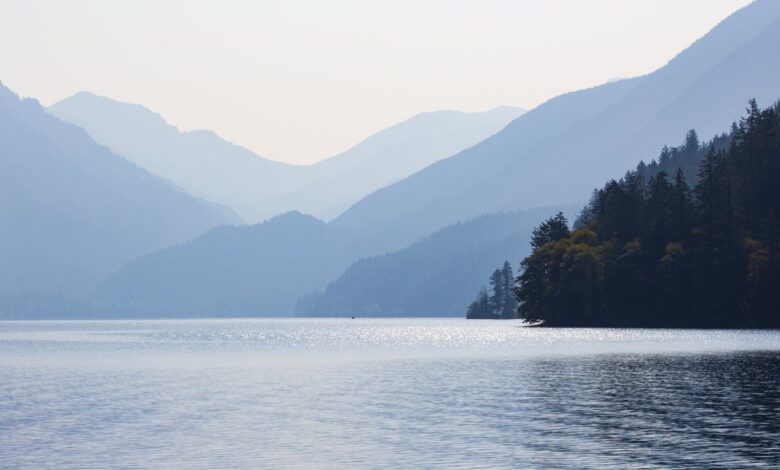
The Apache Software Foundation develops and maintains open source software projects that significantly impact various domains of computing, from web servers and databases to big data and machine learning. As the volume and velocity of time series data continue to grow, thanks to IoT devices, AI, financial systems, and monitoring tools, more and more companies will rely on the Apache ecosystem to manage and analyze this kind of data.
This article provides a brief tour of the Apache ecosystem for time series data processing and analysis. It will focus on the FDAP stack—Flight, DataFusion, Arrow, and Parquet—as these projects particularly affect the transport, storage, and processing of large volumes of data.
How the FDAP stack enhances data processing
The FDAP stack brings enhanced data processing capabilities to large volumes of data. Apache Arrow acts as a cross-language development platform for in-memory data, facilitating efficient data interchange and processing. Its columnar memory format is optimized for modern CPUs and GPUs, enabling high-speed data access and manipulation, which is beneficial for processing time series data.
Apache Parquet, on the other hand, is a columnar storage file format that offers efficient data compression and encoding schemes. Its design is optimized for complex nested data structures and is ideal for batch processing of time series data, where storage efficiency and cost-effectiveness are critical.
DataFusion leverages both Apache Arrow and Apache Parquet for data processing, providing a powerful query engine that can execute complex SQL queries over data stored in memory (Arrow) or in Parquet files. This integration allows for seamless and efficient analysis of time series data, combining the real-time capabilities of InfluxDB with the batch processing strengths of Parquet and the high-speed data processing capabilities of Arrow.
Specific advantages of using columnar storage for time series data include:
- Efficient storage and compression: Time series data typically consist of sequences of values recorded over time, often tracking multiple metrics simultaneously. In columnar storage, data is stored by column rather than by row. This means that all values for a single metric are stored contiguously, leading to better data compression because consecutive values of a metric are often similar or change gradually over time, making them highly compressible. Columnar formats like Parquet optimize storage efficiency and reduce storage costs, which is particularly beneficial for large volumes of time series data.
- Improved query performance: Queries on time series data often involve aggregation operations (like SUM, AVG) over specific periods or metrics. Columnar storage allows for reading only the columns necessary to answer a query, skipping irrelevant data. This selective loading significantly reduces I/O and speeds up query execution, making columnar databases highly efficient for the read-intensive operations typical of time series analysis.
- Better cache utilization: The contiguous storage of columnar data improves CPU cache utilization during data processing. Because most analytical queries on time series data process many values of the same metric simultaneously, loading contiguous column data into the CPU cache can minimize cache misses and improve query execution times. This is particularly beneficial for time series analytics, where operations over large data sets are common.
A seamlessly integrated data ecosystem
Leveraging the FDAP stack alongside InfluxDB facilitates seamless integration with other tools and systems in the data ecosystem. For instance, using Apache Arrow as a bridge enables easy data interchange with other analytics and machine learning frameworks, enhancing the analytical capabilities available for time series data. This interoperability helps build flexible and powerful data pipelines that can adapt to evolving data processing needs.
For example, many database systems and data tools have started supporting Apache Arrow to leverage its performance benefits and become part of the community. Some notable databases and tools in this camp include:
- Dremio: Dremio is a next-generation data lake engine that integrates directly with Arrow and has been an early adopter of Arrow Flight SQL. It uses Arrow Flight to enhance its query performance and data transfer speeds.
- Apache Drill: Apache Drill is an open source, schema-free SQL query engine for big data exploration. Apache Drill uses Apache Arrow for performing in-memory queries.
- Google BigQuery: Google BigQuery takes advantage of Apache Arrow for significant performance gains when transporting data on the back end. Arrow also enables more efficient data transfers between BigQuery and clients that support Arrow.
- Snowflake: Snowflake adopted Apache Arrow and Arrow Flight SQL to avoid serialization overhead and increase interoperability within the Arrow ecosystem.
- InfluxDB: InfluxDB uses the FDAP stack to enable open data architecture, increased performance, and improved interoperability with other databases and data analytics tools.
- Pandas: Similarly, the integration of Apache Arrow with Pandas has led to marked performance improvements in data operations for data scientists using Python.
- Polars: Polars is a DataFrame interface on top of an OLAP query engine implemented in Rust that also uses the Apache Arrow columnar format, allowing for easy integration with existing tools in the data landscape.
All of the databases that leverage Arrow Flight allow programmers to use the same boilerplate to query multiple sources. Pair this with the power of Pandas and Polars, and developers can easily unify data from multiple data stores and perform cross-platform data analytics and transformations. Take a look at the following blog posts to learn more: Query a Database with Arrow Flight and Reading Table MetaData with Flight SQL.
Apache Parquet’s efficient columnar storage format makes it an excellent choice for AI and machine learning workflows, particularly those that involve large and complex data sets. Its popularity has led to support across various tools and platforms within the AI and machine learning ecosystem. Here are some examples:
- Dask: Dask is a parallel computing library in Python. Dask supports Parquet files for distributed data processing, making it suitable for preprocessing large datasets before feeding them into machine learning models.
- Apache Spark: Apache Spark is a unified analytics engine for large-scale data processing. Spark MLlib is a scalable machine learning library that provides a wide range of algorithms for classification, regression, clustering, and more. Spark can directly read and write Parquet files, allowing for efficient data storage and access in big data machine learning projects.
- H2O.ai: H2O is an open-source, distributed, in-memory machine learning platform with support for a wide range of machine learning algorithms. It can import data from Parquet files for machine learning tasks (including forecasting and anomaly detection), offering a straightforward way to use Parquet-stored data in machine learning workflows.
Strong community support and innovation
The Apache ecosystem extends far beyond the FDAP stack. Being a part of the Apache ecosystem and contributing to upstream projects offers many advantages to companies, both technical benefits and business benefits. These advantages include:
- Access to innovations and cutting-edge technologies: The Apache Software Foundation hosts an array of projects at the forefront of technology in big data, cloud computing, database management, server-side technologies, and many other areas. Being part of this ecosystem provides companies with early access to innovations and emerging technologies, allowing them to stay competitive.
- Improved software quality: Contributing to upstream Apache projects allows companies to directly influence the quality and direction of software critical to their business operations. As active participants in the development process, companies can ensure that software meets their standards and requirements. Open-source projects often undergo rigorous peer review, leading to higher code quality and security standards.
- Community support and collaboration: Being part of the Apache ecosystem provides access to a vast community of developers and experts. This community can offer support, advice, and collaboration opportunities. Companies can leverage this collective knowledge to solve complex problems, innovate, and accelerate development cycles.
The Apache ecosystem has made notable contributions to the time series space. By offering a standardized, efficient, and hardware-optimized format for in-memory data, Apache Arrow enhances the performance and interoperability of existing database systems and sets the stage for the next wave of analytical data processing technologies. Apache Parquet provides an efficient, durable file format, easing the transport of data sets between analytics tools. And DataFusion provides a unified way to query disparate systems.
As the Apache ecosystem evolves and improves further, its influence on database technologies will continue to expand, enriching the tool set available to data professionals working not only with time series data but data of all kinds.
Anais Dotis-Georgiou is lead developer advocate at InfluxData.
—
New Tech Forum provides a venue for technology leaders—including vendors and other outside contributors—to explore and discuss emerging enterprise technology in unprecedented depth and breadth. The selection is subjective, based on our pick of the technologies we believe to be important and of greatest interest to InfoWorld readers. InfoWorld does not accept marketing collateral for publication and reserves the right to edit all contributed content. Send all inquiries to doug_dineley@foundryco.com.
Copyright © 2024 IDG Communications, Inc.