Factors Slowing Down Gen AI Adoption
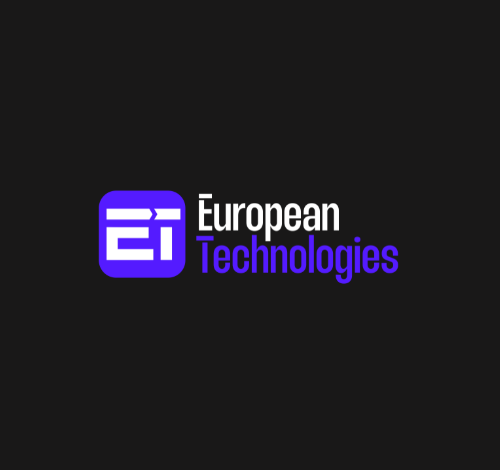
Factors Slowing Down Gen AI Adoption
As generative AI (gen AI) rolled out, it started a conversation at the board and C-suite levels about where companies can use and benefit from the technology.
As I explained in a blog earlier this year, companies changed the decision rights about adopting this technology to add departmental managers. When you add more stakeholders to a decisioning rights cycle, you also complicate and slow that cycle. Over time, the risk, security, and compliance people (who will orchestrate which pieces would become automated and routine) will need to be involved.
What does gen AI really do, and is it worth the effort to get through the compliance, security, and governance issues that the IT organization must put in place? Yes. It is a powerful technology.
Where Adoption Is Happening
Gen AI does not change the tech stack that the department uses. I think the best way to think about it is a product or feature extension for the existing tech stack that departments use.
It does not look like a particularly complicated technology to add to a tech stack. By complicated, I mean there is not a lot of systems integration or change to the existing tech stack. It is a feature extension, a product extension of what departments are already doing.
In most cases, it acts as a productivity boost to an individual or a function that already exists. That is the stage of the current state of play about where most of this going.
Microsoft got it right when it made its product, Copilot. It is complementary to what a company is already doing.
Does it potentially help individuals and functions work faster and better? Yes, in many respects. It is something that most individuals need to learn to use for themselves rather than a fundamental change to the business operations currently underway.
It may evolve over time to have a more expansive effect and radically change business operations. But at this time, most of the adoption looks to be in feature and product extensions of what is already going on.
Yet, this is where adoption seems to slow down or often seems to hit a wall. Here are some of the main factors slowing adoption.
Change Management
Are companies conducting the training necessary for individuals so they understand how to use the gen AI tool in a productive way? This looks a lot like the old change management challenges that companies faced with other technologies.
Price
Complicating the change management issues slowing adoption is ambiguous pricing by the foundational players for their products. Microsoft’s Copilot, for example, is priced at $30 a person. In numerous startups, products are priced at a high cost to operate in their current iteration.
I believe this pricing will fall fairly dramatically over the next 18 months because of several reasons. First, the current pricing is priced to value. Vendors attempt to make the argument that a product is so valuable that customers will pay a lot for it. But as the technology matures, the price to value and competitive tension will bring prices closer to the price to operate.
Another factor affecting price is that it is quite an expensive technology to operate. More advancements will be coming to the market very quickly, which will significantly lower the cost of operation. For instance, the cost of chips will drop. There will be some innovations in place that will make the CPU more prominent in the processing over the NVIDIA chips, which will lower the price further.
In addition, there are some potential efficiencies underway that will make the processing much more efficient in the actual queries.
Open source is another factor that will lower the operating cost and thus increase competitiveness and lower the price. Foundational models can do an adequate job, so companies will move significant tranches of operations into the open source models. This will cause the prices to drop.
Expertise Required
Serious change management and business operations issues will be the major gate that will slow down – and, in many instances, prevent – adoption. These issues will be the last mile to overcome.
Addressing those issues requires multiple skill sets and a multiple disciplinary model. It requires domain expertise, functional expertise, and potentially industry expertise to roll out this technology. A lot of these skills appear to coalesce into prompt engineering, where the user and or business process architects create the filters and questions needed to narrow the scope of answers and frame them in a way that is useful for the users. Although the system integration skills are substantially lighter than we first anticipated, it still requires some technical expertise in order to roll it out.