Five Key Trends in AI and Data Science for 2024
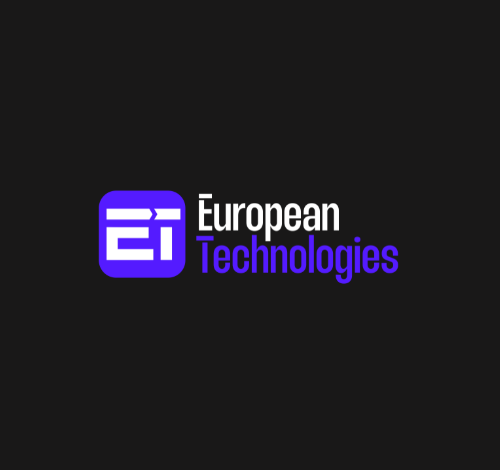
Topics
AI in Action
This column series looks at the biggest data and analytics challenges facing modern companies and dives deep into successful use cases that can help other organizations accelerate their AI progress.
More in this series
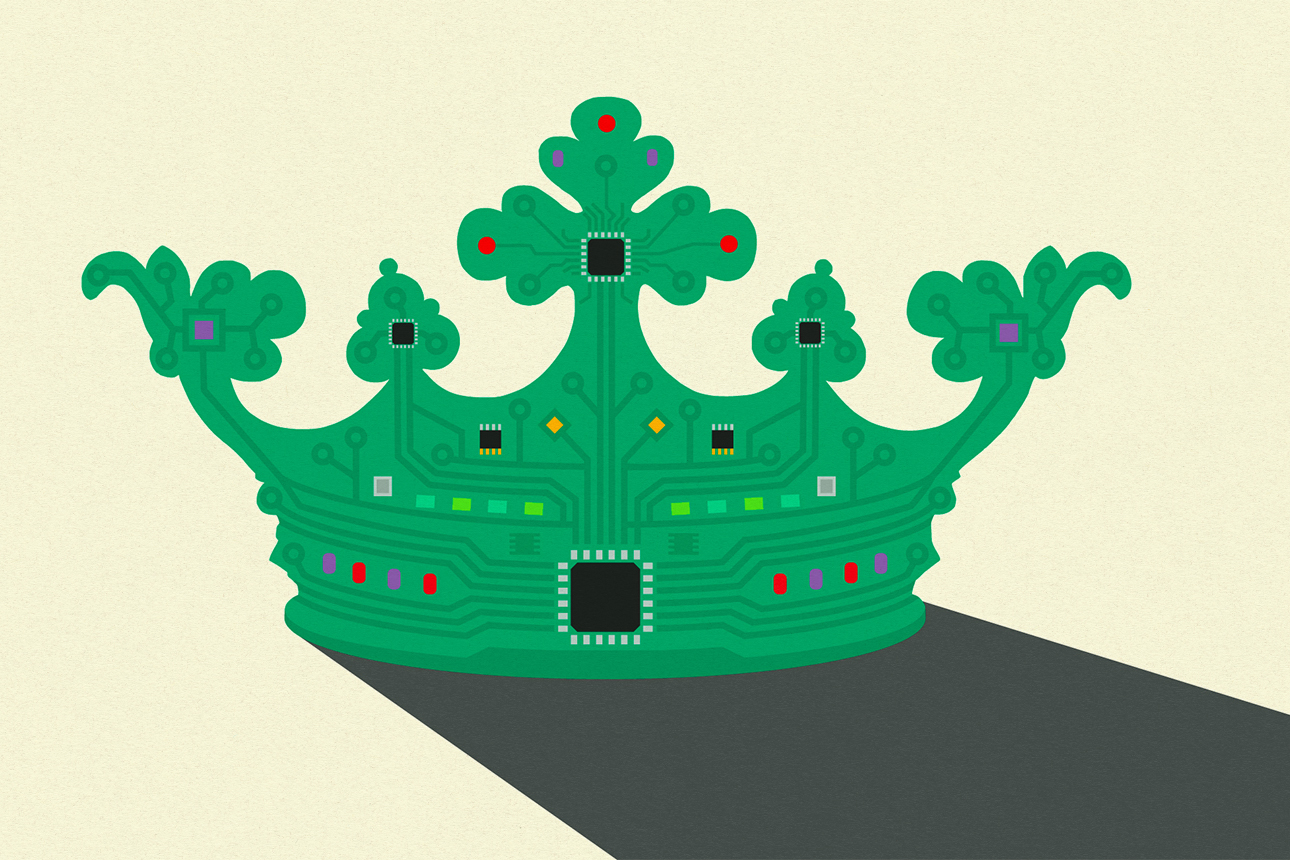
Carolyn Geason-Beissel/MIT SMR | Getty Images
Artificial intelligence and data science became front-page news in 2023. The rise of generative AI, of course, drove this dramatic surge in visibility. So, what might happen in the field in 2024 that will keep it on the front page? And how will these trends really affect businesses?
During the past several months, we’ve conducted three surveys of data and technology executives. Two involved MIT’s Chief Data Officer and Information Quality Symposium attendees — one sponsored by Amazon Web Services (AWS) and another by Thoughtworks. The third survey was conducted by Wavestone, formerly NewVantage Partners, whose annual surveys we’ve written about in the past. In total, the new surveys involved more than 500 senior executives, perhaps with some overlap in participation.
Get Updates on Leading With AI and Data
Get monthly insights on how artificial intelligence impacts your organization and what it means for your company and customers.
Please enter a valid email address
Thank you for signing up
Surveys don’t predict the future, but they do suggest what those people closest to companies’ data science and AI strategies and projects are thinking and doing. According to those data executives, here are the top five developing issues that deserve your close attention:
1. Generative AI sparkles but needs to deliver value.
As we noted, generative AI has captured a massive amount of business and consumer attention. But is it really delivering economic value to the organizations that adopt it? The survey results suggest that although excitement about the technology is very high, value has largely not yet been delivered. Large percentages of respondents believe that generative AI has the potential to be transformational; 80% of respondents to the AWS survey said they believe it will transform their organizations, and 64% in the Wavestone survey said it is the most transformational technology in a generation. A large majority of survey takers are also increasing investment in the technology. However, most companies are still just experimenting, either at the individual or departmental level. Only 6% of companies in the AWS survey had any production application of generative AI, and only 5% in the Wavestone survey had any production deployment at scale.
Surveys suggest that though excitement about generative AI is very high, value has largely not yet been delivered.
Production deployments of generative AI will, of course, require more investment and organizational change, not just experiments. Business processes will need to be redesigned, and employees will need to be reskilled (or, probably in only a few cases, replaced by generative AI systems). The new AI capabilities will need to be integrated into the existing technology infrastructure.
Perhaps the most important change will involve data — curating unstructured content, improving data quality, and integrating diverse sources. In the AWS survey, 93% of respondents agreed that data strategy is critical to getting value from generative AI, but 57% had made no changes to their data thus far.
2. Data science is shifting from artisanal to industrial.
Companies feel the need to accelerate the production of data science models. What was once an artisanal activity is becoming more industrialized. Companies are investing in platforms, processes and methodologies, feature stores, machine learning operations (MLOps) systems, and other tools to increase productivity and deployment rates. MLOps systems monitor the status of machine learning models and detect whether they are still predicting accurately. If they’re not, the models might need to be retrained with new data.
Producing data models — once an artisanal activity — is becoming more industrialized.
Most of these capabilities come from external vendors, but some organizations are now developing their own platforms. Although automation (including automated machine learning tools, which we discuss below) is helping to increase productivity and enable broader data science participation, the greatest boon to data science productivity is probably the reuse of existing data sets, features or variables, and even entire models.
3. Two versions of data products will dominate.
In the Thoughtworks survey, 80% of data and technology leaders said that their organizations were using or considering the use of data products and data product management. By data product, we mean packaging data, analytics, and AI in a software product offering, for internal or external customers. It’s managed from conception to deployment (and ongoing improvement) by data product managers. Examples of data products include recommendation systems that guide customers on what products to buy next and pricing optimization systems for sales teams.
But organizations view data products in two different ways. Just under half (48%) of respondents said that they include analytics and AI capabilities in the concept of data products. Some 30% view analytics and AI as separate from data products and presumably reserve that term for reusable data assets alone. Just 16% say they don’t think of analytics and AI in a product context at all.
We have a slight preference for a definition of data products that includes analytics and AI, since that is the way data is made useful. But all that really matters is that an organization is consistent in how it defines and discusses data products. If an organization prefers a combination of “data products” and “analytics and AI products,” that can work well too, and that definition preserves many of the positive aspects of product management. But without clarity on the definition, organizations could become confused about just what product developers are supposed to deliver.
4. Data scientists will become less sexy.
Data scientists, who have been called “unicorns” and the holders of the “sexiest job of the 21st century” because of their ability to make all aspects of data science projects successful, have seen their star power recede. A number of changes in data science are producing alternative approaches to managing important pieces of the work. One such change is the proliferation of related roles that can address pieces of the data science problem. This expanding set of professionals includes data engineers to wrangle data, machine learning engineers to scale and integrate the models, translators and connectors to work with business stakeholders, and data product managers to oversee the entire initiative.
Another factor reducing the demand for professional data scientists is the rise of citizen data science, wherein quantitatively savvy businesspeople create models or algorithms themselves. These individuals can use AutoML, or automated machine learning tools, to do much of the heavy lifting. Even more helpful to citizens is the modeling capability available in ChatGPT called Advanced Data Analysis. With a very short prompt and an uploaded data set, it can handle virtually every stage of the model creation process and explain its actions.
Of course, there are still many aspects of data science that do require professional data scientists. Developing entirely new algorithms or interpreting how complex models work, for example, are tasks that haven’t gone away. The role will still be necessary but perhaps not as much as it was previously — and without the same degree of power and shimmer.
5. Data, analytics, and AI leaders are becoming less independent.
This past year, we began to notice that increasing numbers of organizations were cutting back on the proliferation of technology and data “chiefs,” including chief data and analytics officers (and sometimes chief AI officers). That CDO/CDAO role, while becoming more common in companies, has long been characterized by short tenures and confusion about the responsibilities. We’re not seeing the functions performed by data and analytics executives go away; rather, they’re increasingly being subsumed within a broader set of technology, data, and digital transformation functions managed by a “supertech leader” who usually reports to the CEO. Titles for this role include chief information officer, chief information and technology officer, and chief digital and technology officer; real-world examples include Sastry Durvasula at TIAA, Sean McCormack at First Group, and Mojgan Lefebvre at Travelers.
This evolution in C-suite roles was a primary focus of the Thoughtworks survey, and 87% of respondents (primarily data leaders but some technology executives as well) agreed that people in their organizations are either completely, to a large degree, or somewhat confused about where to turn for data- and technology-oriented services and issues. Many C-level executives said that collaboration with other tech-oriented leaders within their own organizations is relatively low, and 79% agreed that their organization had been hindered in the past by a lack of collaboration.
We believe that in 2024, we’ll see more of these overarching tech leaders who have all the capabilities to create value from the data and technology professionals reporting to them. They’ll still have to emphasize analytics and AI because that’s how organizations make sense of data and create value with it for employees and customers. Most importantly, these leaders will need to be highly business-oriented, able to debate strategy with their senior management colleagues, and able to translate it into systems and insights that make that strategy a reality.