For Organizations That Depend On AI, Who Is On The AI Team?
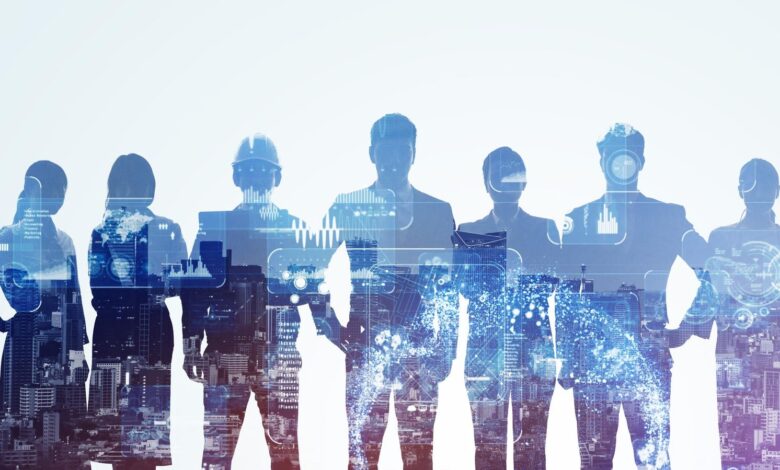
It seems that nowadays everyone is becoming an “AI expert” because they have done some prompt engineering or played around with AI tools that help them create music. Everyone now considers themselves to be an AI developer. However, not too long ago, the world of AI users and AI developers and engineers were very different.
How do you structure an AI team?
Group of people at an organization.
For organizations that increasingly depend on AI, who is really on the AI team? A recent AI Today podcast explored this topic of the evolving AI team. Before LLMs became all the rage, the world of AI users and AI developers and engineers really were very different. Just a few years ago if you wanted to do anything with AI, you needed highly-skilled, well-paid, and hard-to-find individuals. These so-called “unicorns” that companies were trying to look for. Companies couldn’t hire data scientists fast enough and the search for this talent was fierce.
Companies needed these highly skilled data scientists, machine learning engineers, data engineers, and these other well paid and hard to find individuals. They’re hard to find because you can’t just go to a few week code academy or read some things online and become a data scientist or become a machine learning engineer. It took a lot of time to get those skills.
But now with generative AI, really almost anybody can generate stuff with AI. And generative AI has really, truly shifted the landscape of who is able to create these AI outputs. However, it’s important to note that while anyone can now create and interact with AI, that doesn’t mean everyone is an AI Developer.
The Rise of the AI “Citizen Developer”
Generative AI has created a really interesting effect on organizations when it comes to AI teams. A few years ago if you polled organizations and asked who was working on AI projects, most people would probably say they weren’t working on any. But now, if you randomly poll an organization, and you ask that same question of “who here is doing something with AI”, it might actually be the majority of the company. Of course, what they’re thinking about is prompt engineering to help write better emails, or blog posts, or help generate slides. Or, they are using image generation tools, and maybe even AI things that are built into their existing tools with some AI sprinkled in to make them more efficient.
Just as spreadsheets democratized casual quantitative analysis, so too is Generative AI democratizing casual natural language processing and image generation and analysis tasks. The vast majority of Generative AI users are so-called “citizen AI developers” in much the same way that no-code and low-code users have been empowered as “citizen developers”. Some patterns of AI with narrow scope and rapid iteration cycles can indeed be accomplished with Citizen AI Developers. This is especially the case with the conversational pattern, and aspects of pattern & anomaly and recognition patterns.
And, it’s true that many basic AI projects can indeed be done with AI citizen developer casual users. Organizations don’t need to fight over highly skilled and highly paid talent for these applications. But even in these cases, we need to manage the quality and availability of data inputs and outputs, take into consideration trustworthy AI issues, and connect the outputs with other systems.
Also, these generative AI users may think of themselves as doing stuff with AI, they wouldn’t think of themselves as actual deep AI developers. They can’t build ML models or retrain these models. So, the AI citizen developer has a place at the organization, but they aren’t replacing the AI Specialists.
Who are the key players in AI?
AI specialists are still needed because the vast majority of the seven patterns of AI can’t be addressed with simple, generative AI applications. Patterns such as the autonomous pattern, goal driven pattern, or hyperpersonalization pattern require sophisticated, specialized teams and roles.
Take autonomous vehicles, for example. You can’t use large language models to make a vehicle move from point A to point B. So these traditional teams for AI project development are still needed. And these teams consist of data scientists, machine learning engineers, data engineers, operationalization teams as well. So when you’re actually putting your AI into the real world, we need to be able to have teams that can go ahead and actually do that.
The scope of the AI team is also growing. Teams now include AI project management roles as well as Trustworthy AI teams. These groups are responsible for privacy, compliance, risk, ethics, governance, and even aspects of intellectual property licensing and management.
The modern AI team is now much broader and complex than the previous teams of the past that were more “research-y” teams. AI has broadened to be much more all-encompassing, which means that AI is a team effort, and really everyone at the organization is on the AI team.
The Increasing role of AI Project Managers
Technology is only one part of the three legged stool. The other two legs are people &pProcess, which are more important. So many projects fail by throwing bad technology at bad problems. Unfortunately, there are still too many solutions in search of problems.
AI Project Managers and facilitators of all sorts are needed to keep AI projects on track. They help keep projects focused which means AI project management is still incredibly important. And following best practices AI methodologies is equally important. Methodologies such as CPMAI, the cognitive project management for AI methodology, provide PMs that step by step approach needed to AI project success.
As AI becomes core to the organization, the more everyone gets leveled up, and the more you are successfully running and managing AI projects, the more the organization wins!
(disclosure: I’m a co-host of the AI Today podcast)