From Birth to Death: Data Product Management’s Cycle
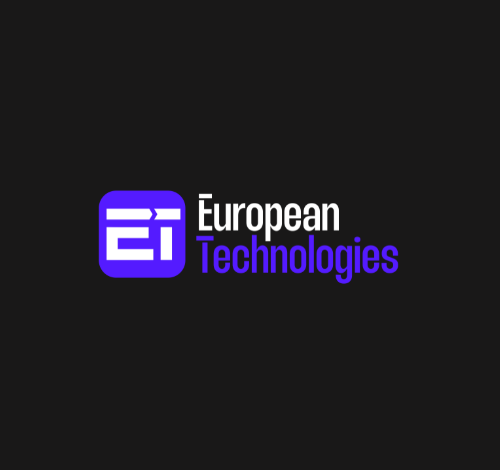
The Gist
- Data insight. Data product management requires a meticulous approach to meet specific business needs.
- Product clarification. What are data products? They can range from raw data sets to comprehensive dashboards.
- Lifecycle perspective. Data products have a defined lifecycle, from inception to potential obsolescence.
“A data product is a self-contained, ready to consume data solution that we organize to solve a specific business problem,” said Aminul Khan, global head of marketing data strategy and data ecosystem at General Motors. “It springs naturally from any organization that manages data as if it were a product — shaping it to meet a specific set of needs for a specific set of customers. Like other consumer products, a data product must be consumer friendly and available at the right time. It must serve a purpose that is in demand and be delivered with an instruction manual (or metadata) on how to use it.”
Devavrat Bapat, head of AI/ML data products for Cisco added, “Data products can be developed for consumers within the organization (internal data products) or for those outside the organization (external data products). Whether internal or external, we have to look at them holistically. We need to make sure it’s useful to somebody.”
Let’s take a look at data product management.
Data Product Management: What Are Data Products?
A data product could simply be a raw data set used by the data science team. Or it could be a dashboard that provides insights to internal operations. Some of the more sophisticated ones mix information from different sources — for example, a 360-degree view of the customer, product information and market surveys.
Data Product Life cycles
All data products have a life cycle that begins with a careful analysis of the requirements and ends when either the needs change or one or more of the data sources is no longer appropriate. Competent data professionals should be involved at both the beginning and the end to make sure the following data product management steps occur:
1) The data product isn’t built until all the right people reach a common understanding of who will use it and why.
2) The data product is killed as soon as it ceases to be relevant.
Related Article: What Does a Data Product Manager Do?
The Birth of a Data Product
“Very often people jump in too quickly, building data systems and pipelines before examining their purpose,” said Khan. “They know where the data is and how to get it, but they don’t necessarily know the why. Unfortunately, this is a common problem, even in today’s world. And I see it in large mature organizations, which tells me we have a lot more to do to educate organizations on data product management practices.”
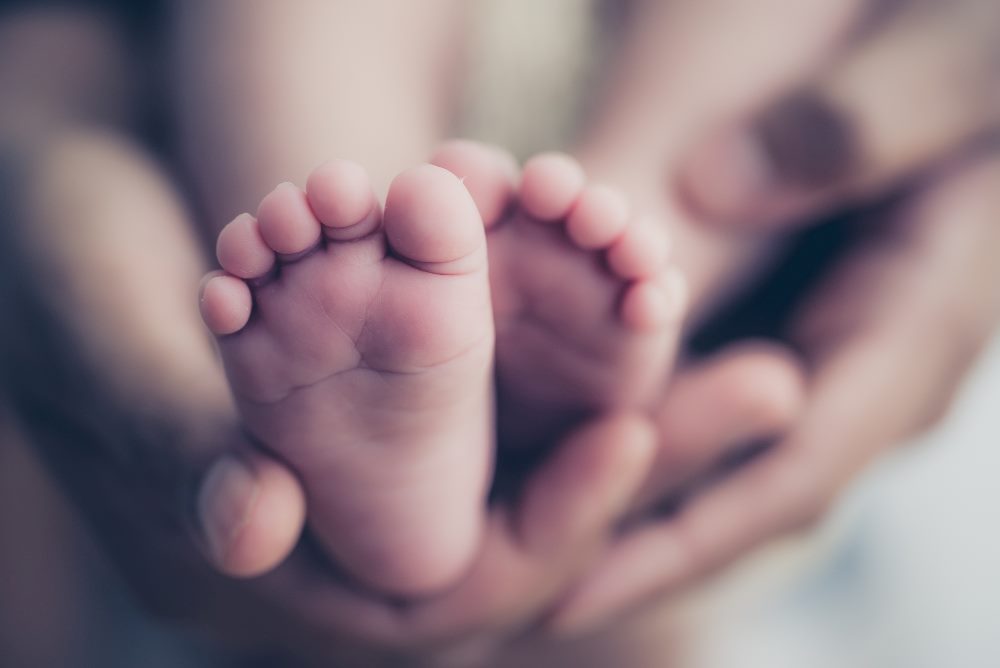
Just like any other product, a data product shouldn’t be designed until all parties involved have a clear definition of the users and their needs. Data leaders should ask at least the following questions before starting to design one:
-
What is it going to do for the business?
-
Are people going to use it or will systems use it?
-
Which kinds of people or systems will use it?
-
How often will it be used?
-
How often does the data need to be updated?
-
How important is the data quality?
“The degree of data quality depends on the use case,” said Khan. “Data needs to be highly accurate and very well defined when compliance is involved. But in other instances, where we are looking to make directional analysis there is acceptable variability on data quality and we shouldn’t let perfect become the enemy of progress .”
Related Article: Finally, a Data Book for CMOs Detailing Data Monetization Strategies
Data Product Challenges
Know the problem
Data professionals should never assume stakeholders and end users know exactly what problem needs to be solved. For example, end users might say they want to automate a process to save them from having to manually extract or analyze data. In this case, the data professional should take the discussion one step further and ask why the data is needed in the first place.
Departmental Issues
Another challenge is that different departments in the organization may be trying to solve different parts of the same problem. The data professional should bring the groups together to figure out how to solve the problem with one data product — or a small number of data products. Sometimes data ownership becomes an issue — one department might want to maintain control over certain information. In other cases, budget may come into the discussion—several departments may have to contribute to the costs of building and maintaining the data product.
Rapid Value Realization
“In the past, I’ve gone through a process called ‘rapid value realization,’ which is similar in concept to a Google Design Sprint,” said Khan. “You bring in stakeholders, customers, and the people who are going to solve the problem. Then you try out different technical solutions by asking participants to act out how they would use each of the potential data products. Ask them, ‘If I give you this now, show me what you would do with it?’
“Going through this process challenges participants to question whether they will truly use the data product you’re building and whether it will deliver the value they need. Sometimes they find out they’ve been asking the wrong questions. The discovery process helps people get their heads around what data — and specifically, what presentation of the data — will help them address their business challenges.”
Figuring It All Out
It’s only after getting the right answers to the right questions that you can start figuring out not only what data you need, but also where you get it, how you present it, and how you control access to it. At this early stage of the life cycle, data professionals should already start thinking about end of life.
Related Article: Customer Data Management Is the Key to Consumer Trust, Profitability
Data Product Management: The Death of a Data Product
Operationalizing the Data Product
“In data product you have two types of life cycle, one is the entire life cycle from ingestion to operationalization,” said Bapat. “I deliberately use the word operationalization to distinguish from using prototypes, because one of the mistakes people make is to develop a nice prototype and then doing nothing to make it ready for operation. Operationalizing the data product is what generates the highest amount of value.”
When to Kill the Data Product
“And then the second level of life cycle is how to keep the data product relevant and when to kill the data product once we believe that it has fulfilled its use. For example, if there is a business model that has migrated from channel-led revenue generation to marketing-led revenue generation, some of the data products required for channel recommendations might no longer be relevant. At some point you have to marry your data product life cycle to the business life cycle, so you can more easily determine which ones to keep and which ones to kill.”
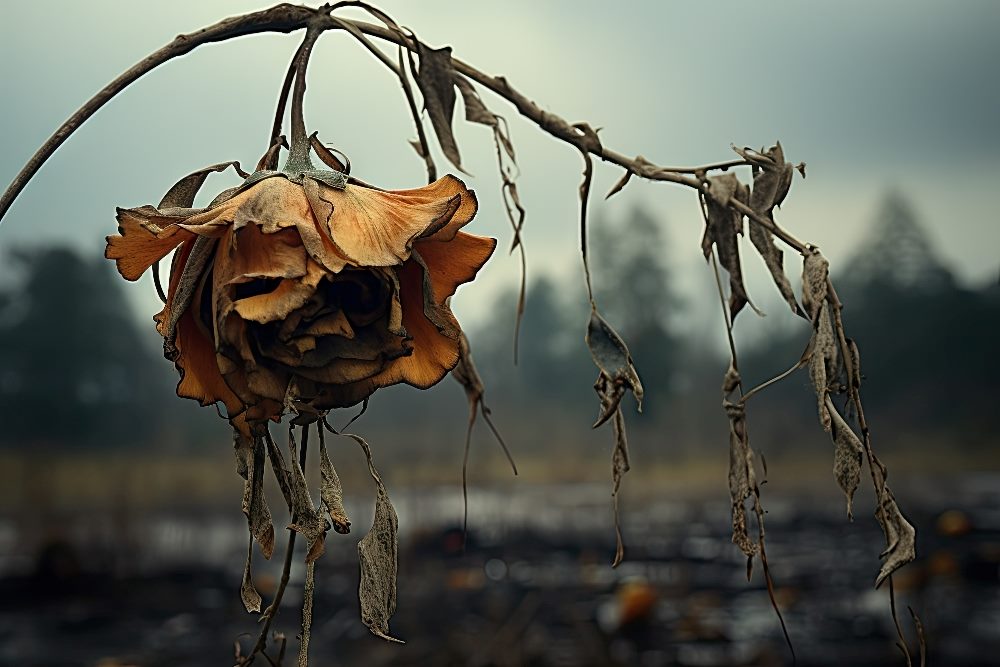
With business strategies and and data product management processes constantly evolving, it’s very easy for a data product to become obsolete, leading to what some data professionals refer to as data pollution — the data behaves differently than expected. “Users don’t have time to look for pollution versus accuracy,” said Bapat. “They only care about the next deal or the next business decision. It’s the responsibility of the data professional to kill a data product that is no longer valid or appropriate.”