Gen AI And Its Malicious Impact On The Cyber-Physical Threat Landscape
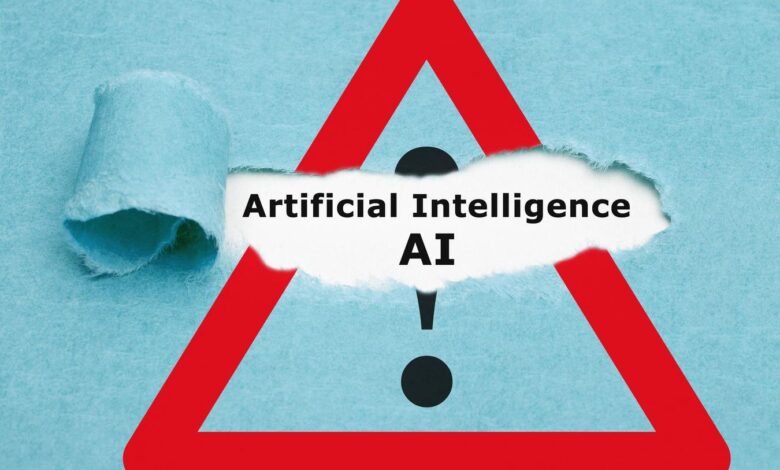
Above all the novel technologies introduced into major industries in recent years, generative AI solutions seem to have dominated the international collective consciousness. While the general public often discusses the broader societal effects, such as the spread of misinformation online and how AI could affect job availability, world leaders and researchers are becoming more worried about more immediate threats.
US intelligence officials have sounded the alarm that recent rapid advancements in AI could outstrip government regulation. At the same time, Microsoft-supported studies reveal that 87% of UK organizations are at risk of AI-powered cyber-attacks. This situation has led to a shifting threat landscape. But what specific malicious uses of general AI are experts concerned about?
The Threats Posed by Gen AI
While the general public may view generative AI as an exciting tool to create content and streamline workflows, the reality for many organizations is slightly more concerning. With the ability to craft convincing fabrications and process large amounts of data autonomously, malicious actors can (and in many cases already have) infiltrate once-impenetrable systems.
In the hands of malicious actors, general AI models have the capability to scan and analyze entire organizational computer systems, identifying and exploiting the most prevalent attack vectors and vulnerabilities. The primary concern is the speed and accuracy at which many modern algorithms can perform such functions, opening the door to significant security risks.
● Social engineering – Gen AI models are increasingly being deployed to create fake credentials, audio files and video footage designed to trick targets into sharing private data and passwords. With studies suggesting only 73% of people can detect AI-generated speech, and many being unable to reliably identify fake images, gaining entry to high-risk systems may now be as simple as asking keyholders for access.
● Physical attacks – As many as 86% of industrial organizations are believed to have adopted Industrial Internet of Things (IIoT) solutions in recent years, meaning internet-connected devices currently control a large number of physical systems. The FBI has warned lawmakers of real-world attempts to infiltrate IIoT systems using gen AI models, with the intention to override physical systems and destroy essential infrastructure. In simulated environments, these attacks have been shown to enable hackers to damage physical equipment, leading to malfunctions, explosions, and fires.
● Data breaches – According to recent reports, as many as 55% of data loss protection events involve users inputting personally identifiable information into generative AI tools, reflecting the general public’s widespread misuse of this technology. This risk becomes even more concerning when hackers intentionally deploy gen AI models to access and expose confidential information through social engineering strategies and brute-force attacks to circumvent traditional cybersecurity protections.
● Theft of technology – A major concern for many organizations is the worry that their own gen AI models could be compromised and turned against them, a threat that has been realized recently through internal breaches of well-known systems. If malicious actors were to gain access to AI models developed by national intelligence agencies, their capabilities would be increased dramatically, posing a significant security threat.
Defending Against Gen AI Threats
With cyber-physical attacks becoming a more common and expected threat for organizations across most major industries, attention must be directed to intelligent defensive installations and strategies. Stakeholders must review existing cyber and physical security solutions with AI-infused processes in mind to reliably protect sensitive systems from sophisticated threats.
If organizations are to accept that gen AI threats will likely continue to impact operations in the coming years, leaders and security teams must find reliable ways to use this technology to their advantage. Research published in 2023 suggests that 53% of organizations acknowledge the relationship between gen AI and cybersecurity risks, though only 38% are believed to be actively mitigating these threats. So, how can gen AI be utilized defensively?
Threat Detection and Response
As gen AI models can continuously analyze new information and adapt to identified changes in threat signifiers, organizations can proactively utilize these tools to protect core systems from sophisticated attacks. Gen AI solutions can be used to autonomously review historical data to identify anomalous actions that could signify novel risks. Systems can then instantly alter key operational configurations to reliably address evolving threats in real time.
By positioning gen AI models to continuously monitor network activity for signs of unusual behavior, organizations can ensure immediate action is taken to contain and neutralize attacks. The concept is particularly important for organizations pursuing some degree of physical and cybersecurity convergence, as attacks can be contained before cybercriminals are able to infiltrate controls associated with physical security systems and IIoT installations.
Vulnerability Patch Generation
Gen AI models wielded by malicious entities often rely on the analysis of an organization’s internal systems to identify exploitable vulnerabilities. Teams can proactively defend against this type of attack by deploying their own gen AI tools. These systems can be designed to automatically generate appropriate virtual patches for newly uncovered vulnerabilities.
Models can draw from internal and external datasets to appropriately test patches in controlled environments. This ensures fixes can be applied and optimized autonomously without interfering with critical operations, compromising physical systems, or incurring unnecessary downtime.
Enhanced Credential Security
Organizations can utilize gen AI models to generate fabricated credentials for testing and optimization purposes. For example, AI-generated biometric data, including facial recognition and fingerprint patterns, can be created to train internal systems to spot fabricated credentials. This same principle can be applied to text-based tactics, helping leaders to teach employees the tell-tale signs of AI-generated social engineering strategies.
Conclusion
Cyber-attacks have been a growing concern for governments and organizations across the world for many years, though with continued advancements in the field of generative AI, it is expected such threats may become increasingly sophisticated. This risk is only exacerbated by the increasing commonality of converged physical and cybersecurity systems, with 90% of global organizations believing cyber-attacks to pose a threat to physical security solutions.
For leaders and security personnel to reliably mitigate the malicious impact of gen AI on the cyber-physical threat landscape, teams must be prepared to use such technologies to their advantage. Organizations can reliably protect key assets from sophisticated attacks by harnessing the power of gen AI to continuously monitor, address, and take action against cyber threat activity.