Generation AI in Asia Pacific
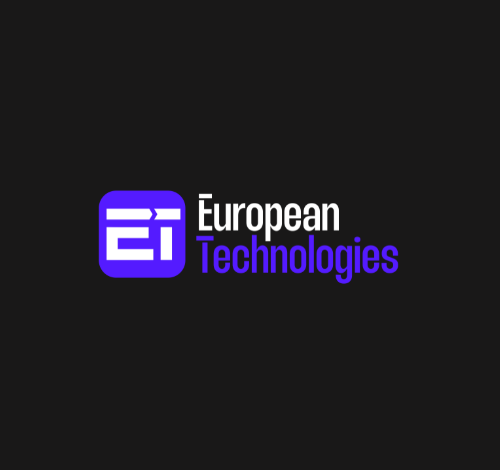
2. Empower your employees to own their AI journey
Employees are taking center stage when it comes to AI adoption. Generation AI has outpaced businesses in making use of this technology at work. Yet business leaders need to empower workers to build the knowledge and capabilities to use this tool effectively in a work context.
Just because an individual employee is more likely to use or is more frequently using gen AI applications does not mean they’re making full use of the technology. Only 50% of employees believe they are fully leveraging the potential of gen AI. Taking proactive action to increase understanding can help. Employees who took more action—such as researching AI, experimenting with applications, and collaborating with peers—are 51% more likely to feel they’re fully utilizing gen AI at work.
The quickly changing nature of gen AI applications means that businesses that try to develop comprehensive training material or courses for their employees will find their material is quickly outdated. Shorter training sessions with practical examples, and even real-world case studies, can be more effective to intrigue employees to learn more.
Leveraging expertise from trusted partners and vendors will help ensure employees receive the latest information. These partners will have the best understanding of what can be done with their products and have a view on lessons around best practices from their client base.
One way to empower employees is to encourage greater collaboration between users within the business. In some cases, competitions or showcases can be used to highlight relevant examples amongst employees and for the business to garner new ideas that otherwise may not have been implemented.
3. Develop your data infrastructure and data governance iteratively as needed to embrace generative AI
Once a business has developed the AI strategy along with the associated use cases to realize value, they’ll need to ensure that their data infrastructure and governance setting enable their desired use cases.
The rapid adoption of gen AI has relied on open-sourced platforms and communities of support. However, more advanced use cases that offer the greatest return for businesses will require AI models to be trained on internal business data.
Traditional forms of AI highlighted the need to improve the quality of structured data including spreadsheets and customer relationship management databases. Gen AI has proven extremely valuable in semistructured or unstructured data such as text documents, emails, video footage, and audio recordings. One example of semistructured data could involve pulling together a proposal to win new work for an engineering company based on previous proposal themes, experience, and methodology.
Data quality is the key enabling factor to leveraging gen AI models trained on business information. However, one Deloitte Access Economics study looking at data maturity levels of businesses across Asia Pacific found that half of businesses have basic or beginner levels of data maturity. This suggests substantial room for improving data capabilities across the region.19
But where should businesses begin when it comes to improving data quality? The gen AI strategy should guide where improvements in data quality offer the largest return or value. Data sets that can be used for multiple purposes, such as employee data accessed by employees themselves as well as human resource reporting and resourcing purposes, should be prioritized when it comes to data cleaning.
Successful gen AI implementation depends on a robust data governance, and working with large language models for business use cases requires the implementation of quality and privacy considerations to drive responsible AI. According to a survey of business and technology leaders conducted for Deloitte’s State of Generative AI in the Enterprise report, the biggest concerns related to governance included: intellectual property issues (35%) and misuse of client or customer data (34%).20 Having an established governance framework for managing risks, ethical issues, and other outcomes to address these governance concerns is a necessary prerequisite for any gen AI use case.