Generative AI: Balancing innovation and risk
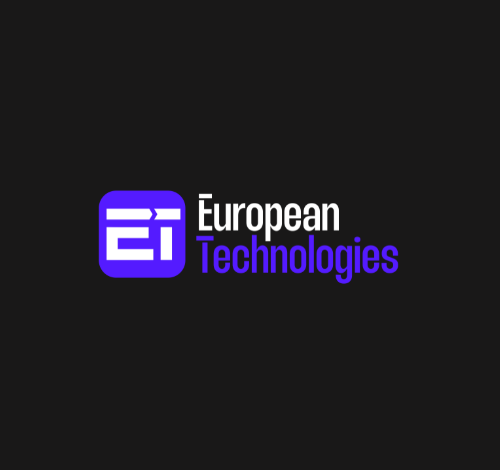
Government organisations want to leverage generative AI to improve the quality of their services. But what should they be aware of in terms of risk and governance?
Like with any emerging technology, it is always a fine balance between innovation and addressing the new risks and challenges that arise. Generative AI has certain particularities because of the way that these large models are trained by using vast amounts of data. Ensuring that the model providers have not breached intellectual property or misuse sensitive data during training is essential to ensure the ethical use of AI as well as continuity of service.
Data privacy is another critical consideration. Public sector bodies need assurances that valuable personal data from citizens and residents has not been used to further train these publicly available models.
Additionally, the potential for generative AI models to “hallucinate” or provide false or misleading outputs is a risk that requires careful monitoring and evaluation.
How can government organisations mitigate against some of these risks?
Firstly, I would recommend that government organisations don’t ban generative AI technologies but rather empower users by creating policies that allow them to experiment responsibly. The same governance controls that apply to other IT applications.
A great way to increase fidelity can be achieved by augmenting the generative AI model with an organisation’s proprietary data. This grounds model responses in a curated knowledge base that contains verified information sources whilst making the generative AI application relevant to the use case.
Government organisations should also continuously evaluate model performance based on custom metrics such as accuracy and safety before selecting the best foundational models (FM) for their use case. Experimenting with different parameters and involving humans in the testing process throughout are important factors to ensure the model is fit for purpose.
AI is only as good as its data – what sort of challenges do organisations face as to the availability and quality of their data?
Data is a strategic asset for government organisations, that can really make generative AI use cases become relevant to their domains.
However, a common challenge for government bodies is data siloing across different agencies and departments. This results in a lack of discoverability and understanding of the data; therefore, increasing the risk of training machine learning models on incomplete or biased datasets, resulting in skewed outputs.
Implementing a business data catalogue can improve data visibility and provide essential metadata like data categorisation and access controls. This empowers those building generative AI applications too.
If you liked this content…
Data quality is paramount, so organisations should adopt a “quality-first” data strategy and apply to it at all stages of the application development lifecycle, from requirements-gathering to continuous monitoring. Automating data quality checks and remediation workflows helps maintain high standards at scale.
What other important aspects should organisations know behind adopting generative AI or any other emerging innovations?
To really accelerate generative AI adoption, government organisations should take a people-centric approach. This starts with ensuring that staff at all levels are trained so that generative AI can be used carefully and responsibly.
In addition to this, ensuring that any new technology is used safely is key. With generative AI, government organisations will want to make sure that they enforce guardrails so that generative AI can’t be used for unethical purposes, for example removing toxic language or avoiding certain topics.
What are some of the applications you’re seeing for generative AI in the public sector?
The top application that I’m seeing is generative AI enhancing existing processes through conversational search interfaces. This allows more intuitive knowledge retrieval for case workers. This is also being applied to healthcare with organisations such as Genomics England, which is using this pattern to identify gene-disease associations in research articles. This knowledge could lead to better diagnoses and outcomes for patients.
Developer productivity is also a rapidly growing use case, with generative AI assisting in previously challenging tasks such as code optimisation and explaining and documenting legacy codebases.
I’m also seeing intelligent document processing being transformed, with generative AI providing summarisation capabilities and conversational search features. This can boost staff productivity with employees spending less time on low-value tasks such as synthesising texts and searching through documentation collections.
What first steps should government organisation take to adopt generative AI in a safe manner?
First, select the right use case. Government organisations will want to prioritise both the most impactful and the most feasible use cases to get started. This is a great opportunity to build confidence in technology across the organisation.
Secondly, empower teams across all levels to innovate with tools and training. Creating a safe environment for staff to experiment with guardrails, and appropriate governance will allow teams to build skills across the organisation.
Finally work towards a proof-of-concept and leverage expertise from industry partners to deliver that first use case successfully before scaling further adoption.