Generative AI vs. Machine Learning
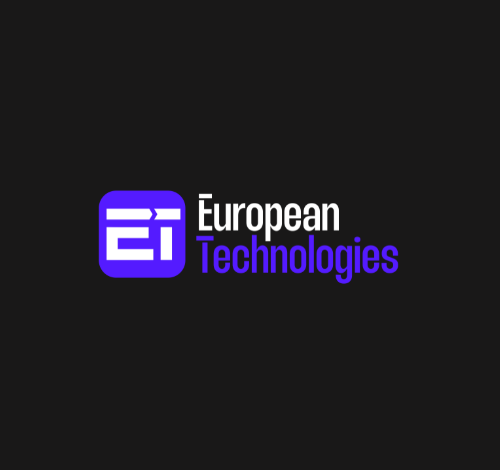
Comparing and contrasting Generative AI and Machine Learning
The hottest topic at Shoptalk in Las Vegas a few weeks ago was generative AI. 44% of attendees surveyed wanted to see generative AI case studies and to learn more about its potential in helping drive business performance.
An interesting follow-up question might have been, “What benefits do you expect from generative AI?” Followed by, “Do you know the difference between generative AI and machine learning?”
Do you know the difference? This article, jointly developed by David Slavick, Founder Ascendant Loyalty and Dr. Mark Chrystal, CEO Netail, gives you a solid overview of what you need to know about both Generative AI and Machine Learning.
We share excerpts from the full article, which you can find here.
Generative Artificial Intelligence (AI): describes algorithms (such as ChatGPT) that can be used to create new content, including audio, code, images, text, simulations, and videos. The most commonly used generative AI tool is ChatGPT which, for us dummies, stands for generative pretrained transformer. Another common generative AI tool is image generator DALL-E (an ode to surrealist artist Salvador Dali and Pixar robot WALL-E).
All the buzz is about Generative AI, but the more “practical” and actionable tools and solutions that marketers use today primarily leverage machine learning. If you’ve evolved from basic modeling and segmentation to machine learning over the past 10 years, you might be surprised to know that this was enabled through supervised machine learning models that both predict and classify. Indeed, you may have also used some unsupervised machine learning models to segment and cluster your data. Supervised learning models use labeled training data and employ a hypothesis of what correct output should look like. Unsupervised learning models do not.
The best method for determining where to use a Machine Learning vs Generative AI algorithm is to identify whether the task involves working with language, images, or numerical data. For language-based tasks, generative AI is likely to provide the best results. Conversely, for numerical analysis, Machine Learning techniques are required.
In fact, generative AI is not good at working with numerical data. Producing new images from text prompts (language) is a generative AI task. At present, analyzing existing images (computer vision) is actually a numerical task and best performed by machine learning models.
Step to Implementing AI in Customer Loyalty include
- Identify Quick Wins
- Experiment with an Expret Partner
- Build Organizational Knowledge
- Develop and Communicate an AI Strategy
- Address Barriers to Progress
- Learn and Repeat
In the future, generative AI will play a crucial role in loyalty and affinity programs by enabling personalized interactions and member experiences via chatbots and website content. Generative AI can deliver the 1:1 personalized experience that members “expect” from today’s digital channels by customizing what they see, how they interact and what offers are presented in any given session.
Your challenge in 2024 is to explore generative AI with your analytical partners and seek opportunities to improve customer interactions at every touch point, at scale. Better customer service (faster, more consistent, more courteous, more accessible – no wait times), and to produce truly personalized content that will get your valued customers to say “they’ve shown me that they know me”.
To learn more, read the full article here and look for David Slavick at the upcoming CRMC event 20-22 May 2024 in Chicago.