Generative vs predictive AI: Both are powerful tools, but manual intervention isn’t going away anytime soon – Opinion News
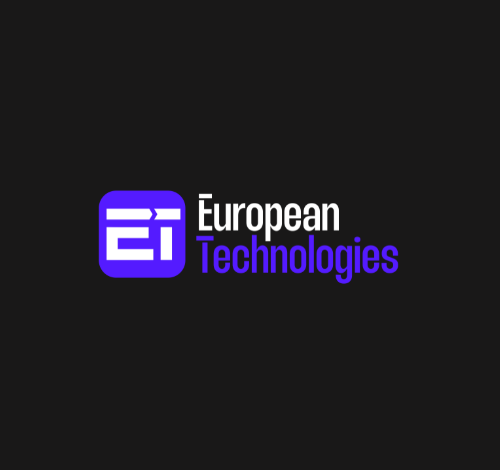
By Siddharth Pai
While generative AI is relatively new, predictive AI has been around for some time, gathering steam and potency with much less fanfare than its newer and flashier younger sibling. For today’s installment, I will discuss a comparison between predictive AI, which has proliferated across a series of applications that touch our lives (whether we know it or not), and generative AI, which is the type that is currently grabbing all the limelight. Both are transformative in their capabilities, but in different contexts.
Generative AI models are designed to create new content. Based on the patterns and data they have been trained on, they can produce text like parts of this column, images, music, and more. Prominent examples include GPT-4 by OpenAI, which generates human-like text, and DALL-E, which creates images from textual descriptions. Just as a lark, I queried it to see if it can help with this column and what it thinks of its genre and the older sibling. To do so, I fashioned specific queries and received feedback on these models’ need for constant human intervention and training to reduce error rates. It acted like a souped-up search engine while responding.
That said, generative AI models are susceptible to several types of errors. First, as I discussed in my last column, they “hallucinate”, meaning they can generate plausible but factually incorrect or nonsensical outputs. For example, a text generation model might produce grammatically correct but factually inaccurate statements. Also inherent in these models is bias. Since these models learn from large data sets — essentially the entire internet — that contain biases, the biases can be reproduced or even amplified. Further, the outputs may lack coherence or relevance while generating creative content, particularly in longer texts or complex images.
Predictive AI models, on the other hand, are designed to forecast outcomes based on historical data. These models are extensively used in finance, medicine, and marketing for stock price prediction, patient diagnosis, and customer behaviour analysis. For some years now, we have been subjected to this piece of AI through targeted marketing and advertising on our smartphones, or we have benefitted from better medical diagnoses or aids to wealth creation by investing in the stock markets.
Like generative AI, predictive AI models also face their own set of challenges. These models might perform exceptionally well on training data but can fail to mould to new, hitherto unseen data, leading to inaccurate predictions. Moreover, the accuracy of predictive models heavily depends on the quality and completeness of the historical data fed to them. Poor data can lead to poor predictions, which can be dangerous when applied in fields like medicine. Further, predictive AI models can mistake correlation for causation, leading to flawed predictions and decisions.
To be fair to this sort of AI, this obfuscation of correlation (when two numbers move together in a defined pattern) with causation (where one number is in fact causing the other number to move in tandem) is a mistake that human statisticians and data analysts have long made. I would also submit that Indian astrologers (the genuine ones) who use sidereal mathematics to predict how mathematically measurable movements in planetary positions affect our future can be prone to this error if they are not experts.
Generative and predictive AI models require extensive manual training and continuous improvement to minimise mistakes. The nature of this training, however, varies significantly. For generative models, the training process involves using diverse and well-curated data sets to train them.
This can help reduce biases and improve the quality of the generated content, and continuously fine-tuning models with specific data sets can help them generate more accurate and relevant outputs. This often involves human feedback loops where outputs are evaluated and corrected. Techniques like reinforcement learning from human feedback can be employed, where human reviewers provide feedback on generated outputs, and the model learns to produce better results over time.
Effective predictive model training requires ensuring that the historical data is clean, relevant, and comprehensive. This might involve dealing with missing values and outliers and ensuring the data represents a real-world scenario.
Additionally, identifying and creating the right features that the model will use to make predictions is crucial. This often requires domain expertise to ensure that the model focuses on the most relevant aspects of the data. Continuously validating the model on new data and adjusting it based on performance helps maintain accuracy and generalisability.
Google DeepMind has pioneered techniques such as using adversarial training in generative models, where two neural networks (a generator and a discriminator) are trained simultaneously, improving the realism and quality of the generated outputs. They have also explored self-supervised learning, where models learn to predict parts of the data they haven’t seen before, enhancing their creativity and accuracy.
Separately, Google has been working on automating this training; CEO Sundar Pichai wrote, “Designing neural nets is extremely time-intensive and requires an expertise that limits its use to a smaller community of scientists and engineers. That’s why we’ve created an approach…. showing that neural nets can design neural nets.” According to Amazon, neural nets create an adaptive system that computers use to learn from their mistakes and improve continuously. Thus, artificial neural networks attempt to solve complicated problems, like summarising documents or recognising faces, with greater accuracy (go.aws/455Y8vG).
Both generative and predictive AI are powerful tools. But their rise will require curated data sets, human feedback, data scrubbing to improve data quality, and meticulous data preprocessing and validation. Manual intervention and supervision aren’t going away anytime soon.
The author is a technology consultant and venture capitalist.
Disclaimer: Views expressed are personal and do not reflect the official position or policy of Financial Express Online. Reproducing this content without permission is prohibited.