Harnessing Data Precision and AI for Global Impact
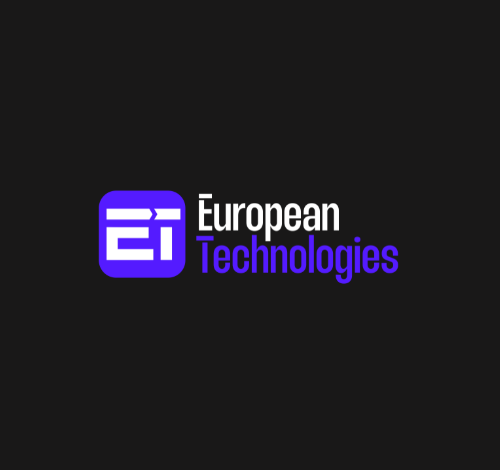
Bharti Patel, SVP of engineering at Hitachi Vantara, explains how a solid data infrastructure gives any organization a sturdy base to innovate, facilitating exploration into AI, automation, and data analysis for future advancements.
Generative AI seemed to come out of nowhere last year, blindsiding everybody. People thought: “This is going to change the world.” And they weren’t wrong. But, more recently, greater realism about AI has set in.
Gen AI is here to stay; more people are asking questions like:
- Can we trust the data?
- Can AI really move the needle for business?
- How can we create a strong data foundation for AI?
Let’s explore and address these questions.
Can We Trust the Data?
In his recent interview with historian and Sapiens author Yuval Noah Harari, late-night talk show host Stephen Colbert said AI models “Are just extensions of us… We made them. They’re us.”
Well, yes. However, AI models are also extensions of the data on which they are trained. And the ownership, quality, and relevance of data can vary greatly. Plus, as Harari said: AI can “Become potentially independent of us,” and it “Is the first technology in history that can make decisions by itself and can create new ideas by itself.”
Even when people are reviewing the decisions and output of AI, which is the best approach today, humans must take great care in how we train and how we use AI. That means ensuring the data on which AI is based is accurate because there is such a huge risk of hallucinations.
AI models based on bad data make bad decisions. Therefore, it is imperative for industries and society to work toward removing biases from data and making sure that data is accurate.
One way to do that is by using internal datasets, as suggested by Eric Hutto, who wrote in Forbes, rather than scraping the internet for data to feed your AI models. Another way is by examining target data sets and, when needed, supplementing them with data to strike the right balance and reflect the reality of situations.
Of course, data can probably never be 100% unbiased because, as Colbert’s comment alludes, the people who create the data are biased themselves. But now that generative AI has become such a force, people need to work collectively as a society to make data less biased.
Can AI Move the Needle for Business?
Some departments and disciplines already use data for reporting. However, such efforts are often aimed at highlighting the success of projects that teams have already executed. Data and AI become more impactful when used to understand what you can do better.
It’s not about finger-pointing. The aim is to build better organizations and outcomes with data.
Some companies are already using data and AI to drive business results. For example, McKinsey reports that mining company Freeport-McMoRan captured data using sensors on trucks, shovels, and stationary machines to get second-by-second performance readings and train AI to find operational improvements. The AI engine empowered the company to improve production by 10%, increasing its annual copper production by 200 million pounds, avoiding $1.52 billion in costs, and contributing to a more than $350 million EBITDA improvement. And because the company didn’t have to open a new plant, it didn’t need to get permits or disturb a new area.
There’s still some work to do on this front, but we’re getting there. AI and machine learning models will help us do that, and data science has progressed significantly in every function.
In the year ahead, expect to see far more organizations use data to gain AI insights and then take deliberate actions to add value rather than just continuing to use data for reporting.
See More: What Google Bard’s Missteps Teaches About Generative AI
How Can We Create a Strong Data Foundation for AI?
The growing use of AI, both by good companies and bad actors, will only elevate the importance of data and increase the risk related to data. Employ a resilient data architecture. That will enable you to store and replicate your data and failover automatically as needed.
The pathology department of St. Mary’s Hospital, a National Health Service hospital run by the Isle of Wight NHS Trust, recently implemented such a data architecture. It creates a strong data foundation on which the organization can build to create new innovations as it explores how AI, automation, and data analysis can support key processes across the pathology department.
AI and data stand to help pathology and patients in significant ways. As experts at leading healthcare organizations published in the September 2023 issue of the Journal of Medical Imaging: Artificial Intelligence and Digital Pathology paper:
“Pathology relies on accurate and timely diagnoses, of which both criteria have the potential to be aided by AI. Pathologists can leverage objective and reproducible evidence to support patient care much more readily than consensus opinion by fellow pathologists through use cases that span quantification, grading, quality control, prediction, and prognosis. It can also be used in an interactive way, guiding pathologists to suspicious regions and helping them interpret images that may otherwise be difficult to interpret.”
Whenever new technology emerges, people ask what the true use case will be. For AI and data, the real use cases are broad. However, the cycle for AI will be much shorter when real use cases arise because things are moving fast. Look for that to happen this year. And start asking – and trying to find answers to – your questions about AI in the months ahead.
Greater realism around AI, growing understanding of the importance of data accuracy, work to lay a strong data foundation for AI, and efforts to use data to drive improvement rather than just reinforce what we did will benefit teams, businesses, and the world in 2024 and beyond.
What steps has your organization taken to achieve data-driven improvements and prevent hallucinations caused by bias? Let us know on Facebook, X, and LinkedIn. We’d love to hear from you!
Image Source: Shutterstock