Here’s What It Means For You
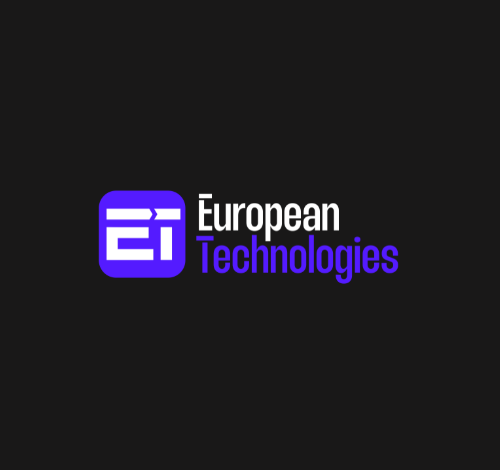
Nvidia CEO Jensen Huang delivers a keynote address during the GTC Artificial Intelligence Conference … [+]
Soon after Apple released the original iPhone, my father, an unlikely early adopter, purchased one. His plan? “I’ll keep it in the trunk for emergencies,” he told me.
At the time, he couldn’t foresee that this device would eventually replace maps, radar detectors, traffic reports on AM radio, CD players, and even coin-operated parking meters—not to mention the entire taxi industry.
Like most people, my father couldn’t imagine how a phone would completely transform our personal and professional lives. His was a typical response to revolutionary technology. We view innovations through the lens of what already exists, fitting the new into the familiar context of the old.
Generative AI is on a similar trajectory. While industries are investing billions in this technology to streamline processes, enhance operations and expedite R&D, even those well-versed in its capabilities find themselves stunned by AI’s breakneck progress. I count myself among them.
Six months ago, I began writing “ChatGPT, MD,” a book on the promise and perils of generative AI in medicine. Initially, I feared my optimism about the technology was too ambitious.
Based on its exponential growth projections—doubling in power every year—I envisioned it becoming a hub of medical expertise for doctors and patients within the next five years (at which point it will be 32 times more capable).
And yet, by the time my book published last week, I realized my estimated timeline might have been too conservative.
In March, Nvidia stunned the tech and healthcare industries with a flurry of announcements at its 2024 GTC AI conference. The California-based chipmaker, which owns 80% of the world’s semiconductor market, released a string of headline-grabbing products and partnership agreements that may radically change everything from diagnosis and care delivery to drug discovery and healthcare economics.
The most striking announcement came when Nvidia and Hippocratic AI revealed their collaboration on generative AI “agents,” which are said to surpass human nurses in performing a variety of tasks, and all at a markedly reduced cost.
According to company-released data, the AI bots are 16% better than nurses at identifying a medication’s impact on lab values; 24% more accurate detecting toxic dosages of over-the-counter drugs, and 43% better at identifying condition-specific negative interactions from OTC meds. All that at $9 an hour compared to the $39.05 median hourly pay for U.S. nurses. These AI nurse-bots are designed to make new diagnoses, manage chronic disease, and give patients a detailed but clear explanation of clinician’ advice.
These rapid developments suggest we are on the cusp of technology revolution, one that could reach global ubiquity far faster than the iPhone. Here are three major implications for patients and medical practitioners:
1. GenAI In Healthcare Is Coming Faster Than You Can Imagine
The human brain can easily predict the rate of arithmetic growth (whereby numbers increase at a constant rate: 1, 2, 3, 4). And it does reasonably well at comprehending geometric growth (a pattern that increases at a constant ratio: 1, 3, 9, 27), as well.
But even the most astute minds struggle to grasp the implications of continuous, exponential growth. And that’s what we’re witnessing with generative AI.
Imagine, for example, a pond with just one lily pad. Assuming the number of lilies will double every night, then the entire pond will be covered in just 50 days. Yet, on day 43, you would see only 1% of the pond’s surface covered. It seems hard to believe that just seven days later, the lily pads will completely fill the pond.
Experts project that AI’s computational progress will double roughly every year, if not faster. But even with conservative projections, ChatGPT and similar AI tools are poised to be 32 times more powerful in five years and over 1,000 times more powerful in a decade. That’s equivalent to your bicycle traveling as fast as a car and then, shortly after, a rocket ship.
This rate of advancement proves challenging for both healthcare providers and patients to understand, but it means that now is the time to prepare for what’s coming.
2. GenAI Will Be Different Than Past AI Models
When assessing the transformative potential of generative AI in healthcare, it’s crucial not to let past failures, such as IBM’s Watson, cloud our expectations. IBM set out ambitious goals for Watson, hoping it would revolutionize healthcare by assisting with diagnoses, treatment planning, and interpreting complex medical data for cancer patients.
I was highly skeptical at the time, not because of the technology itself, but because Watson relied on data from electronic medical records, which lack the accuracy needed to make reliable “narrow AI” diagnoses and recommendations.
In contrast, generative AI leverages a broader and more useful array of information sources. It not only pulls from published, peer-reviewed medical journals and textbooks but also will be able to integrate real-time information from global health databases, ongoing clinical trials, and medical conferences. It will soon incorporate continuous feedback loops from actual patient outcomes and clinician input. This extensive data integration will allow generative AI to continuously stay at the forefront of medical knowledge, making it fundamentally different from its predecessors.
That said, generative AI still requires a couple more generations before it can be widely used without direct clinician oversight. But Nvidia’s bold entry into healthcare signals a long-overdue willingness among tech companies to navigate the legal and regulatory hurdles of healthcare. Once an AI clinician chatbot is available, multiple other companies will quickly follow.
3. GenAI In Healthcare Will Be Ubiquitous (Hospital, Office And Home)
Just as my father never imagined that his iPhone (stored in his trunk) would evolve into an essential tool for navigating life, many Americans struggle to envision the transformative impact generative AI will have on healthcare.
The concept of accessing medical advice and expertise continuously—affordably, reliable, and conveniently around the clock—represents such a departure from current healthcare models that it’s easy for our minds to dismiss it as far-fetched. Yet it’s becoming increasingly clear that these capabilities are not just possible, but likely.
Daily, I receive feedback from both clinicians and patients who have interacted with current generative AI tools. The majority report that the responses, particularly when prompted effectively, align closely with clinician recommendations. This is a testament to the evolving accuracy and reliability of generative AI in healthcare settings, and it promises a revolution in medical care delivery in the near future.
A decade from now, we will look back at today’s skepticism in much the same way I think about my dad’s initial underestimation of his iPhone. We are on the cusp of a major shift, where generative AI will become as integral to healthcare as smartphones have become to daily life.