How Does AI Fit Into Clinical Practice?
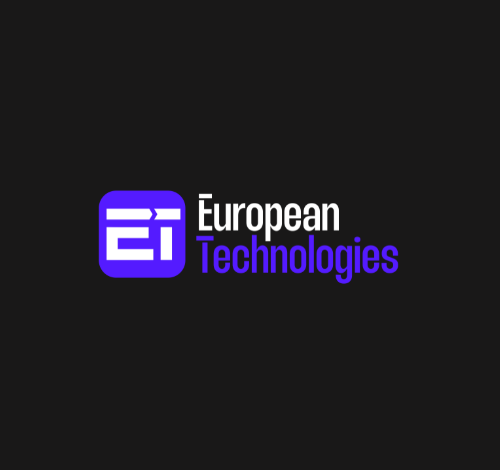
Artificial intelligence (AI) refers to computer systems that perform tasks that typically require human intelligence, such as recognizing patterns or making decisions. The field of AI is rapidly growing, and its applications in the medical field have the potential to revolutionize the way diseases are diagnosed and treated. Though AI is in its infancy, deep learning algorithms have already performed on par with or better than current gold standards for various patient care–related tasks.
Deep learning models have matched human experts’ performance in skin cancer classification, timely identification and adjustment of treatment strategies for septic patients, and medical imaging diagnostic procedures. However, most of these models have been retrospectively tested outside real-world contexts, and there is a lack of randomized controlled medical studies to support the hundreds of AI-enabled medical devices that have been approved by regulatory bodies.
For instance, the Epic Sepsis Model is a proprietary sepsis prediction model implemented in hundreds of US hospitals, but it has not been adequately tested despite widespread use. It showed poor ability to identify septic patients in an external validation test. A literature review on AI implementation in clinical practice in radiology revealed that few studies have been designed specifically to identify radiologists’ and doctors’ attitudes toward adopting AI innovations. These are just some of the factors contributing to uncertainty regarding AI systems in clinical practice, including risks for patients and doctors.
A Scoping Review
To provide a clearer understanding of AI in medicine, a review analyzed randomized studies for AI algorithms that had been used in clinical practice up to 2023 to understand the potential of this technology in improving care management, patient symptoms, and clinical decision-making efficiency. It also identified areas that require further research.
The scoping review included 86 randomized studies that predominantly focused on gastroenterology (43%), followed by 11 (13%) on radiology, five (6%) on surgery, and five (6%) on cardiology. Gastroenterology studies were consistent overall and included analyses of algorithm support for doctors. About 65% of these 37 gastroenterology studies were conducted by four research groups. Out of the total 86, only 18 randomized studies evaluated the effect of AI interventions on quality parameters related to care management in clinical practice.
Imaging, Management, Support
In managing diseases, AI systems have proved able to optimize insulin dosage and monitor hypotension, thus improving the average time patients spend within target ranges for blood glucose and blood pressure. AI also has reduced the rates of acute care and prostate tumor volume in radiation therapy and prostate brachytherapy applications.
In evaluating patient symptoms and behaviors, AI can immediately predict the risk for diabetic retinopathy, thus increasing patients’ adherence to advice compared with those awaiting a doctor’s evaluation. It also can reduce postoperative pain scores through a nociception monitoring system compared with an unassisted medical intervention on the patient.
In clinical decision support, AI can provide cancer mortality predictions that increase disease discussions between oncologists and patients. It also facilitates the identification of patients with atrial fibrillation who are at high risk for stroke and allows the doctor to avoid increasing new anticoagulant prescriptions.
In medical imaging, AI evaluated deep learning systems in 69% of the analyzed studies, predominantly involving videos (71%) rather than images (29%). Most were endoscopic studies (34 of 42 studies) in gastroenterology, in contrast with the predominance of image-based radiologic algorithms in academic literature and regulatory approvals.
International Collaboration
The distribution of studies across clinical specialties suggests that randomized AI studies are concentrated in gastroenterology, radiology, surgery, and cardiology. Notably, there is less emphasis on primary care than on specialized care, suggesting a potential area for future research.
Most studies were conducted in individual countries, primarily the United States, followed by China. According to the review authors, these data underscore a need for increased international collaboration and multicenter trials to ensure the generalizability of AI systems across different populations and healthcare systems.
The prevalence of gastroenterology studies based on videos indicates that clinical AI research is still homogeneous in terms of researchers, study designs, and outcome measures. This scenario requires further research to evaluate the effect of AI systems that incorporate clinical context or patient history in their decision-making process since these are crucial factors for many tasks related to medical practice.
This story was translated from Univadis Italy, which is part of the Medscape professional network, using several editorial tools, including AI, as part of the process. Human editors reviewed this content before publication.