How Generative AI Could Impact Network Planning, RAN Configuration, and Spectrum Management
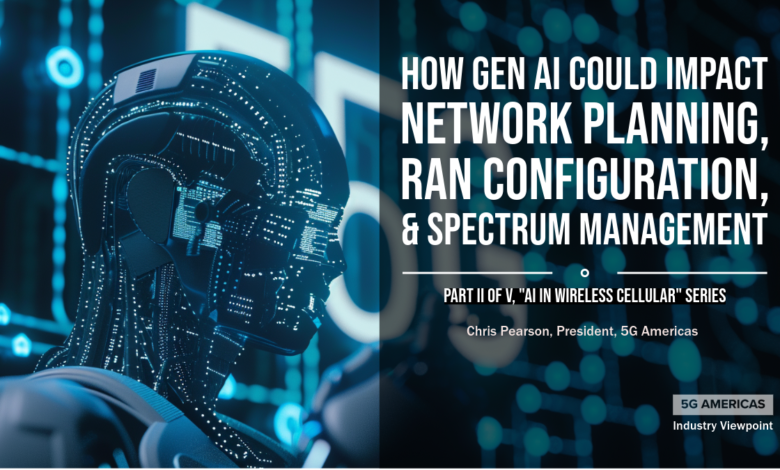
This is Part II of a five-part blog series on AI/ML in 5G and future wireless cellular networks. Part I was here.
Chris Pearson, President, 5G Americas (March 2024) – In my last blog post, AI and ML Evolve Alongside Wireless Cellular Networks, I talked about how artificial intelligence (AI) and machine learning (ML) have been partner technologies since the dawn of 5G networks. Indeed, the concurrent rise of Generative AI and 5G networks have represented both huge challenges and opportunities for both technologies.
In the United States, the first 5G commercial network was announced in December 2018, while OpenAI announced GPT (Generative Pre-trained Transformer), the first version of their transformer-based language model, in June 2018. This initial version of GPTs marked the beginning of a series of advancements in natural language processing technology, leading to the development of more sophisticated models like GPT-2, GPT-3, and subsequent iterations.
As transformer models have exploded onto the scene, the horizon for Generative AI use in wireless cellular networks has also greatly expanded to cover several key areas of network development and management. In this blog post, I’ll discuss several Generative AI use cases in wireless cellular networks – but please note that that is an emerging field. Not everything discussed here has been deployed by all 5G network operators around the world. In many cases, we are looking at proof-of concept stage applications.
But as 5G-Advanced specifications get finalized in 2024, AI/ML integration points to a tantalizing new era of building a more intelligent network. Some of these use cases include:
Network planning and deployment, particularly around automated small cell placement.When it comes to network design telecom companies can use Generative AI to model complex urban environments and predict how different building materials affect signal propagation. For instance, Generative AI could help in determining the optimal locations for small cells in cities to ensure wide coverage with minimal interference. In our joint-authored white paper with Small Cells Forum, entitled Precision Planning for 5G Era Networks and Small Cells, we looked at how Generative AI models can analyze geographical, urban, and environmental data to identify optimal sites for cell towers and small cells by generating simulations of various deployment scenarios to evaluate signal coverage and interference patterns. For instance, a Generative AI model could ingest data from urban maps, including building heights, materials, and density, along with existing network infrastructure, to propose the best locations for new installations that maximize coverage and minimize signal degradation.
Another area where Generative AI can be used involves the design of custom antenna patterns that are optimized for specific environments. Traditional antenna designs might not be efficient in complex urban landscapes or in scenarios where specific coverage patterns are needed. Generative AI can simulate and generate numerous antenna designs, testing each in virtual environments to identify which patterns provide the best coverage and signal strength for a given area. For example, in an area with numerous high-rise buildings, AI could design an antenna pattern that optimizes signal penetration and minimization of dead zones.
Another area involving Generative AI in network planning and deployment involves predictive network modeling for capacity planning. Capacity planning is crucial for ensuring that 5G networks can handle peak loads without degradation of service. Generative AI can create predictive models of network demand, considering variables such as time of day, special events, and even weather conditions that might affect user behavior. By generating and analyzing these predictive models, network designers can plan for necessary infrastructure upgrades or adjustments before demand peaks occur. For instance, ahead of a large public event, Generative AI could simulate the expected increase in demand to ensure the network can scale resources accordingly.
Optimizing fiber deployment routes with Generative AI is an area of growing interest. In connecting cell sites and backbone networks, laying down fiber optic cables is a significant part of network deployment. Generative AI can generate and evaluate multiple routing scenarios, taking into account the cost, geographical obstacles, and existing infrastructure. This process can result in the identification of the most efficient routes for fiber deployment, potentially saving significant time and resources. An example would be using AI to plot fiber routes in a new suburban development, ensuring optimal connectivity for 5G services while minimizing disruption and cost.
Enhancing energy efficiency is an increasingly important function for managing the ongoing operational costs of running a wireless cellular network and addressing global climate change. In Energy Efficiency and Sustainability in Mobile Communications Networks, 5G Americas explored the use of AI/ML modeling in designing network configurations that minimize energy use without compromising service quality. By simulating various network designs and operational scenarios, AI can identify configurations that achieve the best balance between energy efficiency and network performance. For example, it might generate a network design that allows certain components to enter a low-power state when demand is low, reducing overall energy consumption.
Additionally, Generative AI can be used with Radio Access Network (RAN) configuration, where Generative AI algorithms can potentially simulate millions of RAN configurations to find the best settings for specific conditions, such as high-density events in stadiums or concerts, adjusting parameters like power settings and handover thresholds to maintain service quality.
With Dynamic Beamforming Optimization, Generative AI can potentially create algorithms to dynamically adjust beamforming parameters in real-time, optimizing signal direction and strength based on current network conditions and user locations. For instance, in a stadium during a sports event, Generative AI might eventually be used to simulate and generate the optimal beam patterns to ensure consistent and high-quality connectivity for thousands of users, adjusting as the crowd moves or as demand shifts from one part of the stadium to another.
In Adaptive Cell Splitting, Generative AI can be used to simulate and implement adaptive cell splitting strategies in areas of high traffic demand for data. This involves creating smaller, more focused cells within a larger coverage area to manage capacity more effectively. For example, AI could generate configurations that split a cell covering a busy urban district during peak hours, reducing load per cell and improving service quality. This strategy can be reversed during off-peak hours to conserve resources.
With the advent of vRAN (Virtualized Radio Access Network), Generative AI can play a crucial role in configuring virtualized network functions to optimize performance and resource allocation. For instance, it could generate configurations that allocate more processing power to areas expecting high traffic volumes, such as business districts during working hours, and reallocate resources as demand patterns change.
Generative AI can help in creating models to reduce interference between cells, which is crucial for maintaining high-quality service in dense network deployments. By simulating various scenarios, AI can generate configurations that adjust power levels, beam directions, and frequencies to minimize interference. For example, in a densely populated residential area, Generative AI can configure the network to minimize interference at night when most users are at home and using their devices.
Load balancing across Multi-RAT (Radio Access Technology) environments is another key area where Generative AI can be useful.In environments where multiple Radio Access Technologies (RATs) coexist (e.g., LTE, 5G NR), Generative AI can generate configurations that optimally balance load across these technologies. This ensures users are always connected to the best available network based on their current needs and conditions. For instance, AI might shift non-critical data traffic to LTE during peak 5G usage times, keeping the 5G bandwidth available for high priority uses.
One key task of wireless cellular networks involves the critical component of wireless spectrum management. With dynamic spectrum allocation, Generative AI can be used to predict demand spikes and dynamically adjust spectrum allocation. For instance, during a major sporting event, AI could predict increased demand in certain areas and temporarily allocate more spectrum resources to accommodate the surge, enhancing user experience.
With constrained spectrum availability, Dynamic Spectrum Access (DSA) or “Dynamic Spectrum Sharing” (DSS) is becoming an increasingly important topic. This technology was originally designed to allow single network operators to use different radio access technologies, like 4G LTE and 5G NR, on the same spectrum channel. Indeed, it was designed to address the migration of 4G LTE over to 5G networks without requiring the immediate “sunsetting” of the spectrum for 4G LTE customers. In a world of Generative AI, network operators will have an even better tool to maximize the impact of Dynamic Spectrum Access, allowing them to simulate numerous scenarios to predict the best times and locations for its use. For instance, in a scenario where a 5G network shares spectrum with incumbent services.
Generative AI can also potentially be used to cushion the impact of the lack of spectrum availability through generating optimal sharing agreements and dynamic allocation schemes that maximize the spectrum’s utility across different networks. In certain complex scenarios involving multiple operators sharing the same spectrum bands, such as a densely populated urban area where spectrum resources are scarce, Generative AI could potentially help generate a shared spectrum allocation model that dynamically adjusts which operator can use specific parts of the spectrum based on current network load and demand predictions, ensuring fair use and optimal network performance for all.
However, note that creating optimal sharing agreements doesn’t address all the additional challenges of shared spectrum arrangements, such as interference management, equipment compatibility and standardization, fair sharing policies, licensing and rights, QoS and SLA management, investment and revenue sharing due to shared infrastructure or neutral host environments, data security and privacy, and other competitive dynamics.
By analyzing past data on spectrum demand across different times and locations, Generative AI can also predict future spectrum requirements and generate allocation strategies that preemptively address these needs. For example, ahead of a large public event, Generative AI could forecast increased demand for mobile services and generate a temporary spectrum allocation plan that accommodates this surge in traffic, ensuring high service quality without overloading the network.
As we covered in 5G Americas’ white paper Radio Frequency Receiver Performance, there is a growing challenge of out of band interference. With the growing use of all spectrum bands, managing interference becomes even more significant. Generative AI can create models that simulate the behavior of various devices and services within these different spectrum bands, generating strategies to optimize coexistence. For instance, it could develop a protocol for dynamically adjusting transmission power and channel selection for 5G NR-U deployments in urban areas, reducing interference with existing Wi-Fi networks while maximizing throughput for 5G users.
Generative AI can assist in the global harmonization of spectrum use by generating models that simulate the impact of different regulatory approaches on international spectrum availability. For example, it could analyze the potential for cross-border interference under different spectrum allocation schemes and generate recommendations for harmonizing use in border regions, facilitating smoother deployment of 5G services and reducing conflicts between neighboring countries.
For instance, countries A and B share a long border, and both are in the process of rolling out 5G networks. However, the spectrum bands allocated for 5G in Country A are already being used for satellite communication in Country B, leading to potential interference issues. Moreover, the regulatory frameworks and allocation strategies of the two countries have historically been misaligned, complicating cross-border spectrum management.
The Generative AI solution might begin by collecting and analyzing historical and real-time data on spectrum usage, regulations, and deployment plans from different countries, identifying potential areas for cross-border coordination based on interference patterns. It then employs Generative AI to simulate thousands of spectrum allocation scenarios across border regions, considering factors like population density, geography, existing infrastructure, and future plans. These simulations can help in identifying harmonization strategies that minimize interference and optimize spectrum use, such as dynamic sharing, coordinated allocations, or buffer zones. The AI further refines these strategies to recommend optimized allocation plans and regulatory changes, facilitating stakeholder engagement through detailed reports and visualizations that showcase the benefits and feasibility of these strategies. Upon agreement, the AI supports implementation and continues to learn from new data to adjust the harmonization strategy, ensuring effective spectrum use as conditions evolve.
As you can see, within the domain of network planning, deployment, RAN and spectrum management, there are a large number of ways that Generative AI can be potentially used. In Part III, we will take a deep dive into how it can be used in content optimization and delivery, virtualization and Network Slicing, and enhanced user experiences.
-Chris