How generative AI helps deliver value-based testing
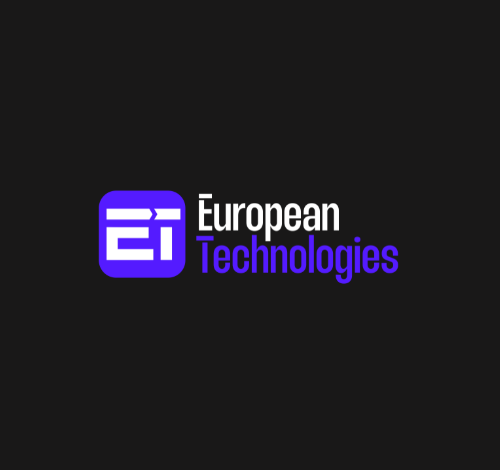
In association with Keysight Technologies
By Jonathon Wright, chief technologist, Keysight Technologies
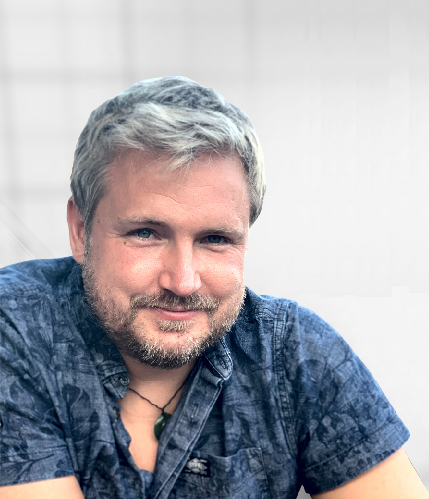
The world of IT regularly changes out of all recognition. When IT in business largely consisted of mainframe applications, client service, and thick client systems, users were expected to follow the workflow of the application. If you didn’t follow the workflow and something didn’t work, it was your fault, not the system’s fault.
Testing built up around similarly rigid architectures, with exacting governance, standards, and organisations around them.
Today, we have multiple devices, applications, and connectivities. The importance of user experience (UX) means applications must work how users want them to, not vice versa.
This has all made testing a thousand times more complicated than it used to be. But in the same time, testing hasn’t really evolved.
Choose two of better, faster, cheaper
Yet, competition is fiercer than ever. Whether it’s internal IT systems or customer-facing systems, organisations need faster release cadences to enhance customer satisfaction and drive competitive advantage, delivered at lower cost with better quality.
But as we all know, when it comes to the well-known product triangle of better, faster, cheaper, you can only choose two.
Agile and DevOps helped us to speed up release cadences and introduced the concept of the minimum viable product.
Still, testing struggles to keep up with DevOps speeds, and that’s a problem that isn’t easily solved.
You can increase speed by lowering testing requirements, but this reduces quality. You can increase quality by maintaining testing requirements, but this reduces speed. You can throw resource at testing to increase speed and maintain quality, but this increases cost. In the first two scenarios, you damage the user experience. In the third, you cost the business more.
Test automation goes some of the way to helping, but not far enough. And so, business starts to lose faith in the IT function’s ability to deliver what’s required – solutions that are better, faster, and cheaper.
At the heart of this reputational issue is whether the business views the QA function as a cost or as an enabler. Too often, it is seen as a cost.
Testing is a critical business enabler
In fact, QA should be viewed as an enabler – doing what the business needs it to do – driving the quality that will enhance the UX, increase customer satisfaction, boost reputation, and deliver competitive advantage.
So, how do we deliver testing better, faster, and cheaper to restore testing’s reputation and have it viewed as a critical business enabler?
The answer is that you use Gen AI tools such as Generative AI (GAI). GAI turbo-charges the human-in-the-loop so the QA function becomes the value-adding team that gives the business what it wants.
A quick intro to GAI
Eggplant Generative AI (GAI) is a fine-tuned large language model (LLM) specifically designed for testing.
The base model is trained on ISO/IEEE/BSI/ISTQB testing material from trusted sources, giving confidence on the quality of the inputs.
It is also trained on industry verticals such as healthcare, telecommunications, or aerospace and defense, so it brings industry-specific insights. And it gives its sources, so you can apply knowledge to confirm the quality of the response.
The base model can be improved to the user’s precise requirements by feeding it proprietary knowledge. And it is offline, it 100 per cent secure and EU AI Act-complaint.
GAI can generate all the automation assets, all the models, and all the tests needed in any scenario. It can also streamline requirements and provide test case optimisation for example by eliminating duplicates.
With the upcoming release of Sentient Test Expert (STE) from Keysight’s Eggplant, you can give STE any digital interface, and it will utilise next-generation cognitive reckoning technology powered by Large Action Models (LAM) and Large Vision Models (LLaVA) to create a Universal Language Test Translator (ULTT) that allows STE to autonomically test it.
Human testers can assess the test suggestions, apply their own knowledge to discard the ones that add no value and ask STE to run the ones that have possibilities.
These capabilities mean that test automation can finally realise its potential.
Supercharge the human-in-the-loop
The critical component of a truly successful DevOps approach is someone who is a specialist in the business side of things and a specialist in the technical requirements of implementation and testing.
GAI has both. When you layer humans-in-the-loop on top, you add in the knowledge worker with wisdom to be able to validate and verify that everything expressed by every stakeholder and every permutation is correct.
It gives you the ability to translate the subject matter of the business into something the IT and the technical teams can use to build an application and automate its testing.
This speeds up the pipeline and helps people on both sides of the equation to collaborate and work together effectively.
It means we can properly shift left, bring testing right into the requirements phase of development, embed UX from the start – and elevate the value of testing. It’s a game-changer that helps us deliver what the business wants and needs.