How to spot a deepfake: the maker of a detection tool shares the key giveaways | Artificial intelligence (AI)
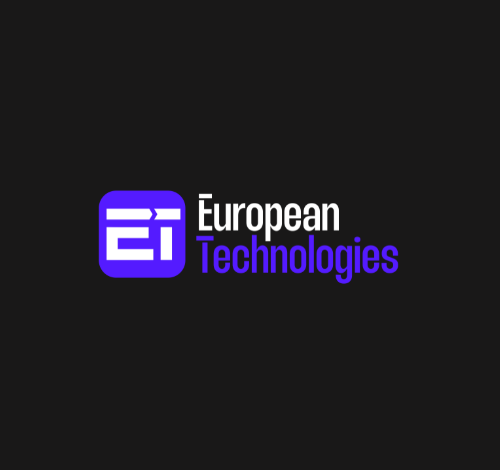
You – a human, presumably – are a crucial part of detecting whether a photo or video is made by artificial intelligence.
There are detection tools, made both commercially and in research labs, that can help. To use these deepfake detectors, you upload or link a piece of media that you suspect could be fake, and the detector will give a percent likelihood that it was AI-generated.
But your senses and an understanding of some key giveaways provide a lot of insight when analyzing media to see whether it’s a deepfake.
While regulations for deepfakes, particularly in elections, lag the quick pace of AI advancements, we have to find ways to figure out whether an image, audio or video is actually real.
Siwei Lyu made one of them, the DeepFake-o-meter, at the University of Buffalo. His tool is free and open-source, compiling more than a dozen algorithms from other research labs in one place. Users can upload a piece of media and run it through these different labs’ tools to get a sense of whether it could be AI-generated.
The DeepFake-o-meter shows both the benefits and limitations of AI-detection tools. When we ran a few known deepfakes through the various algorithms, the detectors gave a rating for the same video, photo or audio recording ranging from 0% to 100% likelihood of being AI-generated.
AI, and the algorithms used to detect it, can be biased by the way it’s taught. At least in the case of the DeepFake-o-meter, the tool is transparent about that variability in results, while with a commercial detector bought in the app store, it’s less clear what its limitations are, he said.
“I think a false image of reliability is worse than low reliability, because if you trust a system that is fundamentally not trustworthy to work, it can cause trouble in the future,” Lyu said.
His system is still barebones for users, launching publicly just in January of this year. But his goal is that journalists, researchers, investigators and everyday users will be able to upload media to see whether it’s real. His team is working on ways to rank the various algorithms it uses for detection to inform users which detector would work best for their situation. Users can opt in to sharing the media they upload with Lyu’s research team to help them better understand deepfake detection and improve the website.
Lyu often serves as an expert source for journalists trying to assess whether something could be a deepfake, so he walked us through a few well-known instances of deepfakery from recent memory to show the ways we can tell they aren’t real. Some of the obvious giveaways have changed over time as AI has improved, and will change again.
“A human operator needs to be brought in to do the analysis,” he said. “I think it is crucial to be a human-algorithm collaboration. Deepfakes are a social-technical problem. It’s not going to be solved purely by technology. It has to have an interface with humans.”
Audio
A robocall that circulated in New Hampshire using an AI-generated voice of President Joe Biden encouraged voters there not to turn out for the Democratic primary, one of the first major instances of a deepfake in this year’s US elections.
When Lyu’s team ran a short clip of the robocall through five algorithms on the DeepFake-o-meter, only one of the detectors came back at more than 50% likelihood of AI – that one said it had a 100% likelihood. The other four ranged from 0.2% to 46.8% likelihood. A longer version of the call generated three of the five detectors to come in at more than 90% likelihood.
This tracks with our experience creating audio deepfakes: they’re harder to pick out because you’re relying solely on your hearing, and easier to generate because there are tons of examples of public figures’ voices for AI to use to make a person’s voice say whatever they want.
But there are some clues in the robocall, and in audio deepfakes in general, to look out for.
AI-generated audio often has a flatter overall tone and is less conversational than how we typically talk, Lyu said. You don’t hear much emotion. There may not be proper breathing sounds, like taking a breath before speaking.
Pay attention to the background noises, too. Sometimes there are no background noises when there should be. Or, in the case of the robocall, there’s a lot of noise mixed into the background almost to give an air of realness that actually sounds unnatural.
Photos
With photos, it helps to zoom in and examine closely for any “inconsistencies with the physical world or human pathology”, like buildings with crooked lines or hands with six fingers, Lyu said. Little details like hair, mouths and shadows can hold clues to whether something is real.
Hands were once a clearer tell for AI-generated images because they would more frequently end up with extra appendages, though the technology has improved and that’s becoming less common, Lyu said.
We sent the photos of Trump with Black voters that a BBC investigation found had been AI-generated through the DeepFake-o-meter. Five of the seven image-deepfake detectors came back with a 0% likelihood the fake image was fake, while one clocked in at 51%. The remaining detector said no face had been detected.
Lyu’s team noted unnatural areas around Trump’s neck and chin, people’s teeth looking off and webbing around some fingers.
Beyond these visual oddities, AI-generated images just look too glossy in many cases.
“It’s very hard to put into quantitative terms, but there is this overall view and look that the image looks too plastic or like a painting,” Lyu said.
Videos
Videos, especially those of people, are harder to fake than photos or audio. In some AI-generated videos without people, it can be harder to figure out whether imagery is real, though those aren’t “deepfakes” in the sense that the term typically refers to people’s likenesses being faked or altered.
For the video test, we sent a deepfake of Ukrainian president Volodymyr Zelenskiy that shows him telling his armed forces to surrender to Russia, which did not happen.
The visual cues in the video include unnatural eye-blinking that shows some pixel artifacts, Lyu’s team said. The edges of Zelenskiy’s head aren’t quite right; they’re jagged and pixelated, a sign of digital manipulation.
Some of the detection algorithms look specifically at the lips, because current AI video tools will mostly change the lips to say things a person didn’t say. The lips are where most inconsistencies are found. An example would be if a letter sound requires the lip to be closed, like a B or a P, but the deepfake’s mouth is not completely closed, Lyu said. When the mouth is open, the teeth and tongue appear off, he said.
The video, to us, is more clearly fake than the audio or photo examples we flagged to Lyu’s team. But of the six detection algorithms that assessed the clip, only three came back with very high likelihoods of AI generation (more than 90%). The other three returned very low likelihoods, ranging from 0.5% to 18.7%.