It’s not only AI that hallucinates
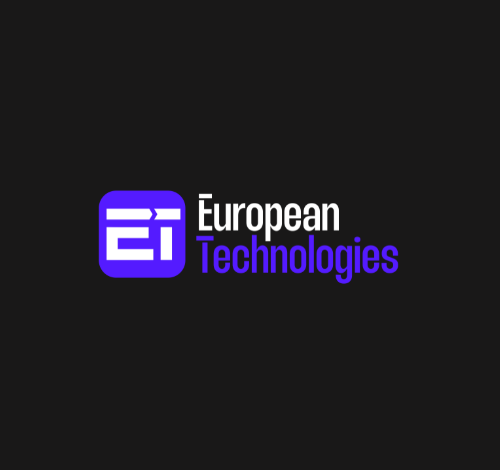
Stay informed with free updates
Simply sign up to the Artificial intelligence myFT Digest — delivered directly to your inbox.
It may be rash to extrapolate from a sample size of one (me). But I confess that my memory is not perfect: I forget some things, confuse others and occasionally “remember” events that never happened. I suspect some FT readers may be similarly muddle-headed. A smart machine might call this human hallucination.
We talk a lot about generative AI models hallucinating facts. We wince at the lawyer who submitted a court document containing fictitious cases invented by ChatGPT. An FT colleague, who prompted the chatbot to produce a chart of the training costs of generative AI models, was startled to see that the most expensive one it identified did not exist (unless the model has access to inside information). As every user rapidly discovers: these models are unreliable — just like humans. The interesting question is: are machines more corrigible than us? It may prove easier to rewrite code than rewire the brain.
One of the best illustrations of the fallibility of human memory was the testimony given by John Dean, legal counsel to the White House in Richard Nixon’s administration. In the Watergate hearings of 1973, Dean was known as “the human tape recorder” because of his remarkable memory. But unbeknown to Dean, Nixon had installed a real tape recorder in the Oval Office. Researchers have therefore been able to compare Dean’s account of critical conversations with the written transcriptions.
In a 1981 paper analysing Dean’s testimony, the psychologist Ulric Neisser highlighted several glaring lapses and reinterpretations of conversations in the lawyer’s account — as well as the difficulty of defining truth and accuracy. In his paper, Neisser drew a distinction between semantic and episodic memory. Dean was roughly right in remembering the overall gist of his conversations with Nixon — and the nature of the Watergate cover-up — even if he was precisely wrong about the details of particular episodes.
One could argue that large language models do the opposite: given all the data they ingest they should have good episodic memory (although with garbage inputs they can generate garbage outputs). But they still have poor semantic memory. Although an LLM would probably summarise the Oval Office recordings more faithfully than Dean recalled the conversations months later, it would have no contextual understanding of the significance of that content.
Researchers are working on ways to further improve generative AI models’ episodic memory and reduce hallucinations. A recent paper from Google DeepMind researchers proposed a new methodology called Safe — search-augmented factuality evaluator. Model-generated responses are broken down into constituent sentences and cross-checked against Google Search for factuality, or factual correctness. The paper claims this experimental system outperforms fact-checking human annotators in terms of accuracy and is more than 20 times cheaper.
“In the next few years we will be able to fact check the output of large language models to some good accuracy. I think that’s pretty useful,” one of the paper’s authors Quoc Le tells me. Hallucinations are both a feature of LLMs to be welcomed when it comes to creativity and a bug to be suppressed when it comes to factuality, he says.
In the meantime, LLMs can still muddle up creativity and factuality. For example, when I asked Microsoft Bing’s Copilot to tell me the world record for crossing the English channel on foot, it confidently replied: “The world record for crossing the English Channel entirely on foot is held by Christof Wandratsch of Germany, who completed the crossing in 14 hours and 51 minutes on August 14, 2020.” Handily, it even provided a citation for this fact. Unfortunately, the reference turned out to be an article posted last year highlighting the hallucinations generated by ChatGPT.
We should not only focus on how content is created, but also how it lands, according to Maria Schnell, chief language officer at RWS, which delivers tech-enabled text and translation services to more than 8,000 clients in 548 language combinations. In a world in which content is increasingly cheap and ubiquitous, it will become all the more important to tailor information to a specific audience in a format, language and cultural context they understand, and that requires a human touch.
“Accuracy is comparatively easy to automate. Relevance is not a given,” Schnell says. “We have to think about how content is received and that is where AI struggles.”
For the moment, at least, humans and machines can work fruitfully together to magnify their different capabilities and minimise their respective flaws.