Keeping the human touch: Why people remain essential in the age of AI
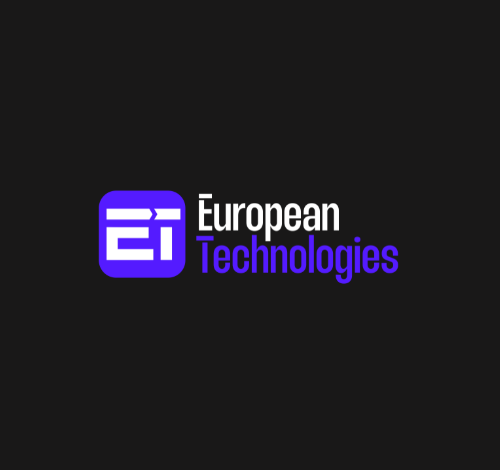
It’s a year since the launch of ChatGPT, an event that marked an inflection point in AI adoption. This innovation catapulted AI from a futuristic concept to an everyday reality. As the speed and sophistication of AI tools increase, we must ask ourselves: Where does human agency sit in an AI-enabled future?
To answer this question, consider a lesson from Betty Crocker. When the iconic instant cake mix was launched in the 1950s, suddenly home bakers needed only to add water and stir. The process was virtually effortless and foolproof, producing the perfect sponge cake in minutes. Nevertheless, many US housewives were not keen.
In a bid to boost sales, the brand brought in consumer psychologist Ernest Dichter, who uncovered the problem: the cake mix was so simple to use that housewives felt guilty for doing so. So, Betty Crocker changed the recipe to ask bakers to add fresh eggs, an extra step that, while elementary, added a “personal touch” and changed how customers perceived and valued the brand.
The story demonstrates the reluctance to dispense with human effort and a counterintuitive desire to retain some personal involvement in process (even if less convenient than letting the AI do its work unaided), a phenomenon now known as the “Betty Crocker Effect.” Today’s AI developers should take note: full automation is not always the first choice for customers, even if it’s the easiest, cheapest or most efficient option.
The human advantage
For businesses, the attractions of AI are obvious. Almost 85% of top executives believe it will give their organizations a competitive edge, whether that’s through reduced costs, increased efficiency, improved customer service, better forecasting or faster decision-making. As this technology continues to gain traction, the global AI market is expected to reach up to $2tn by 2030.
But in our haste to adopt AI, we must not forget the value of the human touch. While some advanced AI systems may replicate the human decision-making process, they may never access the subjective insights and instincts accumulated through years of lived experience.
Imagine a machine learning (ML) algorithm programmed to manage the supply chain of an industrial manufacturer. The program could recommend a particular driver, route and vehicle to transport materials from factory to warehouse, considering real-time factors such as traffic, logistical capacity and current weather conditions. In most cases, it would be able to identify the most efficient option, relying on data metrics alone.
However, the AI model has inbuilt limitations. For instance, it can’t account for unforeseen variables that deviate from historical data, or the socio-cultural factors that influence situations – a driver who’s likely fasting for Ramadan, for example, or a flash flood causing congestion in a nearby town. The model can handle known patterns and scenarios, but it lacks the data to adapt to sudden changes. Nor can it modulate the decision-making process to consider more intuitive influences, such as a driver returning from a long lay-off owing to illness.