Leveraging AI Tools to Navigate and Integrate Big Data
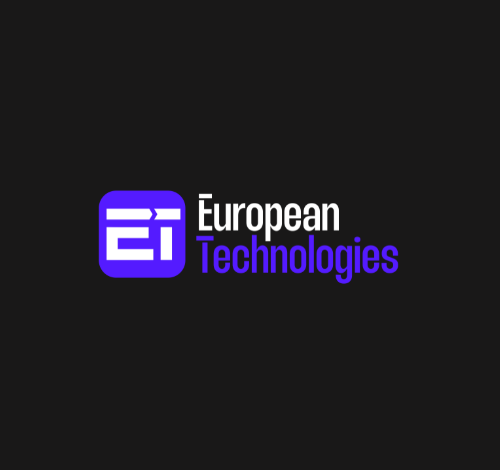
68293_LM_Informatics_eBook_JL V3
INFORMATICS RESOURCE GUIDE
Leveraging AI for Streamlined Data Integration in Modern LIMS and Informatics
Insights on Navigating your Information using AI and Machine Learning tools
ENHANCING
Data Management and Integration
BUILDING
a Robust Bioinformatics Platform
STREAMLINING
Laboratory Operations
Table of Contents
3 Tackling Complexity with Clarity: Harnessing AI Tools for Data Management and Integration in Modern Laboratory Informatics
5 Enhancing Data Management and Integration in Modern LIMS with AI and ML Tools
8 Streamline your Lab’s Operations by Adopting a Lab Integration System
10 Building Resilience: A Short Guide to Implementing a Robust Bioinformatics Platform
14 Can an ELN be Used as a LIMS?
2 Lab Manager
16 AI Solutions to Streamline Cell Line Development Processes
Introduction
Tackling Complexity with Clarity: Harnessing AI Tools for Data Management and Integration in Modern Laboratory Informatics
Cutting-edge AI tools provide clear solutions to integrate and manage vast volumes of data with ease and reliability for efficient processes and precise outcomes
In today’s rapidly evolving scientific landscape, laboratory information management systems (LIMS) play a major role in managing data integral to research and development across nu- merous scientific disciplines. Despite their extensive use, as laboratories continue to generate increasingly complex and greater volumes of data, researchers have begun incorporating artificial intelligence (AI) and machine learning (ML) technologies within LIMS as solutions for big data integration and management.
Introduction
From enhanced data integration capabilities to improved data analysis and interpretation, AI and ML tools elevate LIMS and promote the transition to adaptive learning systems that offer automated compliance and quality control, user-friendly interfaces, and predictive mainte- nance. They provide these benefits while setting the stage for a highly optimized laboratory research environment.
Leveraging AI and ML tools in the laboratory helps enhance and transform data management from a laborious, manual task to a dynamic, streamlined process with significant improve- ments in operational efficiency.
This e-book discusses trends in AI tools and innovations in modern LIMS, streamlining laboratory operations using a lab integration system,
implementing a robust bioinformatics platform, debating the possibility of using ELNs as LIMS, and AI solutions to streamline cell line development. The proper implementation and use of AI tools in modern laboratories can assist researchers in deriving holistic insights from their experiments and freeing time from rudimentary and repetitive tasks for what is most important, research.
Enhancing Data Management and Integration in
Modern LIMS with AI and ML Tools
AI and ML help empower LIMS in a data-centric approach toward efficient laboratory operations and processes
by Ajay P. Manuel, PhD
Artificial intelligence (AI) and machine learning (ML) technologies have transformed the rapidly evolving digital landscape. This is especially true in the case of laboratory environments where researchers face the daunting reality of tackling an ever-increasing volume and complexity of data. Modern Laboratory Information Management Sys- tems (LIMS) have begun adopting AI and ML tools to streamline laboratory processes, enhance collaboration and decision-making, and promote a data-centric approach to laboratory operations and scientific research.
What do AI and ML have to offer for LIMS?
Incorporating AI and ML in laboratory operations can help in various ways:
Enhance efficiency and cost-effectiveness concerning instrument maintenance, data integrity, inventory man- agement, and stability studies
Predict system failures, alleviate instrument downtime, and enable preventative maintenance
Monitor experimental results in real time while helping maintain data integrity, and keep tabs on audit trails
Optimize inventory management, and streamline work- flow processes via software automation
Ensure regulatory compliance and facilitate compre- hensive sample tracking
The benefits of AI and ML for laboratory data management and integration are vast and can help streamline processes, promote data-centric decision-making, and reduce costs.
AI-enhanced laboratory systems can provide alternative solutions for laboratory operations and also help optimize re- source utilization. As a result, this allows researchers to save valuable time that may otherwise be spent on rudimentary and repetitive manual tasks.
Challenges and Considerations
The convergence of AI and ML technologies in LIMS empowers researchers with various tools to handle large vol- umes of complex data, make effective decisions, and estab- lish operational visibility. Nevertheless, challenges remain. Implementation of AI and ML technologies is not cheap and
requires significant initial investments. Data security and privacy concerns alongside compliance with regulatory stan- dards and ever-evolving frameworks are also crucial for the successful implementation and incorporation of these tools in diverse laboratory environments. As AI and ML tools con- tinue to be developed at a breakneck pace, researchers can expect further innovations to help drive alternative solutions to these challenges and promote greater integration of these technologies in modern laboratories.
Empowering LIMS
A variety of AI and ML tools are now utilized to enhance existing LIMS:
-
Natural Language Processing (NLP) algorithms enable computers to understand and process human language. With LIMS, NLP helps automate data entry by extracting relevant details from unstructured data while enhancing data accuracy and accessibility.
-
Robotics Process Automation (RPA) involves the use of software robots to automate repetitive tasks such as data entry and sample logging with LIMS. RPA helps reduce the weight of data management, especially within their integration across platforms such as ELNs, while providing for streamlined data flow.
-
Predictive Analytics, powered by machine learning models, can elevate LIMS by helping anticipate equipment failures, optimize resource allocation, reduce downtime, enhance
efficiencies, and altogether set the pace for effective inventory management.
-
Image Recognition and Processing algorithms can help analyze data in images, and for LIMS, automate experimental image analysis in microscopy and chromatography as well as improve data connectivity and usability for storing, retrieving, and analyzing image data.
-
Decision Support Systems powered by AI can help lab managers make informed decisions based on the corresponding analytics on data managed by LIMS.
-
Anomaly Detection using ML can be utilized with LIMS to help labs identify early experimental outliers due to procedural errors or important discoveries, thus helping save precious resources, and time, and improving the reliability of experimental outcomes.
Streamline your Lab’s Operations by Adopting a Lab Integration System
Integrated data streams are essential to making effective data-driven decisions for your lab operations
by Ajay P. Manuel, PhD
Thhere precision and accuracy are concerned, critical, da- ta-driven decisions are not just a preference but a necessity.
This is especially true in laboratory environments where data-driven decisions play a vital role in enabling lab manag-
ers to make informed choices and pave the way for efficient and safe scientific inquiry reinforced by effective operational management. Lab integration systems help support these
efforts in their ability to bring together data from various lab assets and informatics platforms for holistic decision-making.
Data umbrella
Data in laboratories are often stored in separate databases, departments, or systems, making data management a major challenge for lab managers. A lab integration system helps consolidate data from various sources into a single, cloud- based repository. The once-siloed data now becomes far more accessible, interoperable, and reproducible. This is particularly helpful for researchers to establish holistic con- clusions on the results of relevant experiments. Unlike LIMS and ELNs that require manual tasks such as file transfers and data transcription, lab integration systems help researchers reclaim their valuable time by automating data streams and reducing errors.
Building an infrastructure
Getting your lab ready for an integration system requires some processes and infrastructure to be set in place. To allow a smooth transition, these processes must be relatively stable so that they can be automated easily. For starters, the lab must have minimal cloud infrastructure, underutilized instruments, and an established wet lab. Every lab has its own unique set of workflows and processes with some neces- sitating custom development. Thus, it is equally important that a lab is also equipped with dedicated IT support to lead the implementation of data automation.
What defines a lab integration system?
A robust lab integration system possesses a user-centric de- sign that streamlines data management, promotes collabora- tion, and accelerates scientific research. Several key features that identify with an ideal lab integration system include:
Effective data capture and harmonization, for con- sistency and interoperability, from diverse sources including instruments, PCs, APIs, cloud platforms, and external services
Automated workflows that reduce manual intervention and minimize the risk of errors
Seamless integration with existing laboratory soft- ware and tools
Efficient data traceability and reproducibility through the management of metadata associated with experi- mental protocols and results
Flexibility and accessibility for enhanced collaboration for users across the organization
Easy scalability and adaptability to growing laboratory needs, data volumes, and expanding workflows
Advanced analytical capabilities alongside compre- hensive audit trails and version control mechanisms
User-friendly interface and intuitive design for ease of use and maintenance supplemented by healthy securi- ty measures to protect data integrity and confidentiality
How do lab managers benefit?
An effective lab integration platform makes the lab manager’s life easier by offering a unified approach to data management and decision-making. Through automation and interoper- ability, lab integration systems help drive innovation and collaboration in scientific research while providing solutions to rudimentary and repetitive manual tasks, minimizing human error, and helping unlock new insights.
“A robust lab integration system possesses a user-centric design that streamlines data management, promotes collaboration, and accelerates scientific research.”
Building Resilience: A Short Guide to
Implementing a Robust Bioinformatics Platform
How to ensure success when developing a reliable and scalable biological data environment
by Jordan Willis, BSc
The goal of any bioinformatics platform is to function as a centralized infrastructure of tools and resources that support analytical and computational processes to manage and eval- uate biological data. These platforms must be robust, able to perform consistently and scale up with the lab. Bioinformat- ics platforms can vary widely in design and scope, but some common implementation and maintenance strategies will help keep your platform robust.
Define the launch objectives and system requirements
Thhat is the main purpose and function of the bioinformat- ics platform in your research? Are there features, software, operating systems, or bioinformatics pipelines that must be included to handle specific data types? Thhat are the inputs (e.g., RNA-seq data) and the outputs (e.g., data visualization and statistical analysis)? How will the data be stored?
Having a well-defined concept to build upon is crucial for understanding where the project is heading. Compatibility between operating systems, software, and bioinformatics pipelines is paramount for seamlessly producing and analyz- ing data. Educate yourself on the differences between storage infrastructures such as local storage, cloud computing, or a hybrid system. These choices can affect the throughput and scalability of your platform.
A knowledgeable, well-trained user base with access to accu- rate documentation will increase both user productivity and confidence in their results.
Provide documentation, user training, and feedback mechanisms
Thho will be using the platform and how will they be trained and onboarded? How will software and training materials
be stored, accessed, and reviewed? Thill there be a dedicated support team or help desk? How will users provide feedback regarding bugs and errors?
A knowledgeable, well-trained user base with access to accurate documentation will increase both user productivity and confidence in their results. The ability to rapidly provide feedback to support teams will facilitate timely responses and minimize downtime. Set a schedule for documentation review to ensure materials are updated.
“A knowledgeable, well-trained user base with access to accurate documentation will increase both user productivity and confidence in
their results.”
Perform system testing and quality assurance
How will the system and software be benchmarked and analyzed? Thhich quality assurance tests will be performed before launch? How often will the system undergo mainte- nance and performance testing?
Predefine the maximum workload that the platform can perform by using stress tests with high data volume and user counts. Testing for data integrity, quality control, stress, and reproducibility will ensure that the data is valid, and the retrieval and analysis results are consistent between runs.
Validate security protocols and system monitoring
Is the platform open to the internet? Thhat security measures (e.g., firewalls, data encryption, etc.) will be implemented?
How will security and user activity be monitored?
The continued success of your platform launch hinges upon predictive planning.
Verification of the user authentication process can help determine any user-based security concerns. Verify that sensitive tasks requiring high-level security clearance are locked to specific user roles. Routine security reviews and penetration testing can identify technical issues or suspi- cious activity.
Scaling up and staying flexible
How can your platform remain flexible? Thhen is the appro- priate time to scale up your infrastructure? How will up- grades to the platform’s existing infrastructure be addressed?
User count, computational demands, and storage require- ments will increase over time and must be scaled up appro- priately through various methods such as scalable storage solutions (e.g., cloud-based), database optimization, and
load-balancing mechanisms for systems and servers. Platform flexibility can be achieved through modular architecture design and plug-and-play hardware, allowing for digital components to be added or removed as needed to facilitate new workflows or data types.
Planning is critical
The continued success of your platform launch hinges upon predictive planning. By answering the hardest questions during planning, you’ll develop a platform that provides a robust backbone for your research needs.
Product Spotlight
A complete laboratory solution to improve your scientific experience!
Thermo Scientific™ SampleManager™ software serves as the ultimate solution for managing your laboratory, data, and procedural workflows seamlessly. Comprising a laboratory information management system (LIMS), scientific data management system (SDMS), and lab execution system (LES), this software integrates with other enterprise systems, instruments, equipment, and even your customers’ systems, ensuring enhanced compliance and productivity throughout your business.
Unlike traditional software solutions that require extensive customization, SampleManager software offers unparalleled configurability to align with your specific business processes and laboratory workflows. This flexibility enables you to tailor the software precisely to your needs without the need for costly and time-consuming customization efforts.
SampleManager LIMS elevates productivity, data integrity, and compliance within your organization. With the latest version of SampleManager LIMS, users can experience an enhanced desktop interface, offering a more spacious, intuitive, and accessible user experience. Additionally, features such as sample containment, chain of custody management, and barcode interaction have been further optimized, providing users with a superior scientific experience.
Can an ELN be Used as a LIMS?
Thhether an ELN or LIMS is the best solution for you depends on the type of lab you run and what it needs
by Gail Dutton and Ajay P. Manuel, PhD
Thhether an electronic lab notebook (ELN) can double as a lab information management system (LIMS)-and vice
versa-depends on the type of lab you run and what it needs. If your lab is running experiments to advance science and develop new products, its needs are considerably different from those of quality assurance or public health labs that engage in high-volume sample testing or routine analyses.
Traditional ELNs and LIMS are both used for specific pur- poses and also have their fair share of limitations.
Consider their purpose
ELNs are designed for use at the bench to help scientists capture and describe experiments. The timelines they pro- vide can be vital in protecting intellectual property claims, especially when those claims are challenged by competitors.
They generally enhance efficiency and reduce overall costs, but they are not a panacea. Although ELNs may provide a level of data analytics, help manage workflows, and are search-
“…the focus of LIMS is on what happened in the experiment, but not why or how it happened.”
able, they are unable to report on sample and structured ex- perimental data for accurate, flexible, and efficient reporting.
A LIMS, in contrast, manages scientific samples, test data, and processes system-wide, from sample registration through the reporting of results. They provide sample-specific sum- maries of test results across multiple tests and protocols and also track reagents. Because they enable automated work- flows, they help reduce human errors during data input and sample processing. They do not, however, store the details
of the experiments and, therefore, lack the context needed to interpret the results. Experts state that the focus of LIMS is on what happened in the experiment, but not why or how it happened.
Therefore, for R&D organizations, a LIMS cannot replace an ELN. But, for routine testing labs, neither can an ELN replace a LIMS. Instead, lab managers find they often need elements of both in one solution.
Hybrid solutions may work
To meet this need, the lines between LIMS and ELNs are blurring. The convergence of these two systems has been happening over the past decade with ELN providers adding features to manage samples and procedures whereas LIMS providers have added lab-oriented features. This progress is seen in action via LIMS and ELN solutions that provide digital coverage of laboratory activities and cloud-based data management systems that bridge ELN and LIMS
helping effectively process data while also allowing user raw data access.
The convergence of ELNs and LIMS has been happening for the past decade.
The benefit is ease of management with experts stating that application convergence can reduce the number of solutions to implement, much like the case of convergence of separate printer, fax, and scanning devices into all-in-one systems in the early 1990s.
Focus on your Goals
In deciding between a basic ELN or LIMS, or a hybrid solu- tion that offers some of each, experts recommend that users think about what they will use the software for. Both ELN and LIMS include some level of tracking capabilities. Report generation is another commonality, enabling LIMS reports to be added to ELN records. LIMS is more suitable for perform- ing sample analysis while ELNs help track sample inventory.
Thhile some hybrid systems may blend capabilities effec- tively, be conscious of potential trade-offs in each solution you consider. Additionally, many vendors still tend to work within their own hardware ecosystems or with limited third parties. Consequently, interoperability with other systems and with a variety of lab instruments may be an issue.
Beyond the capabilities of the systems themselves, also consider your lab’s IT environment. For example, determine whether the system you favor needs a cloud computing system or specialized IT infrastructure. Think about how it would be integrated into your inventory, registration system, and other databases and what that entails to enable data to be moved among systems and instruments as needed. Consult your IT department and be sure to balance the scientific and business aspects of any system you are considering.
Lab managers in biotech and pharmaceutical companies or others creating new science need the ability to track and document experiments, so an ELN is needed. On the other hand, a LIMS is suitable for routine lab testing facilities. As such, before deciding for your lab, take the time to deter- mine exactly what you need and why. For labs that need the scientific functionality of an ELN as well as the tracking and management capabilities of a LIMS, a hybrid may be the best choice.
“The convergence of ELNs and LIMS has been happening
for the past decade.”
AI Solutions to Streamline Ce l Line Development Processes
Digital solutions, enhanced by AI and ML tools, can improve processes, data analysis, and collaboration in cell line development
by Morgana Moretti, PhD and Ajay P. Manuel, PhD
Cell line development (CLD) plays a crucial role in drug discovery and the manufacturing of biopharmaceuticals- usually recombinant proteins such as monoclonal antibodies, bispecifics, and biosimilars.
Data silos between CLD process steps, operators, and labs can lead to rework, bottlenecks, and issues with data integri- ty and compliance.
Avoiding these problems in the face of more data points than ever requires solutions aimed at consolidating data, making it accessible and understandable, and enabling interfaces that maximize monitoring and collaboration. Advanced bioinfor- matics tools and knowledge management systems, incorpo- rating artificial intelligence (AI) and machine learning (ML) tools, are becoming increasingly important in this context.
The digital lab approach: incorporating AI and ML tools
The digital lab approach involves leveraging software and other digital tools to streamline laboratory processes, data analysis, and communication. The incorporation of AI and machine learning tools are added enhancements, and the ben- efits are multifold: improved data accuracy and transparency, efficient resource management, better decision-making, and elevated collaboration among research groups and sites. As such, AI and ML tools have a lot to offer in cell line devel- opment and can help transform CLD from a traditionally seg- mented process to an integrated and streamlined workflow.
Bioinformatics, LIMS, and the CLD process
The CLD process encompasses several stages, from vec- tor construction and sequence verification to cell growth/ expansion and data management. Digital solutions offer the means to optimize the process while ensuring high-quality outputs and strict compliance with regulatory standards.
Nevertheless, manual analysis and review of the procured data remains a necessity at every stage of the CLD process, particularly, microscopy. Remote inspection, facilitated by cloud storage and automated imaging, has somewhat helped bridge this gap but the persistent need for routine monitor- ing and decision-making remains a handicap.
Current bioinformatics software can process, interpret, and visualize data. Thhen combined with cloud computing plat- forms, they can efficiently manage the large datasets typical
Tips for choosing AI tools in cell line development
-
Prioritize those that integrate well with existing lab infrastructure, LIMS, equipment, and database systems.
-
Make sure to consider that the relevant AI tool can handle the diverse types of data collected in the CLD workflow.
-
Lean towards AI tools that have been rigorously tested and validated using independent datasets.
-
Look for AI tools that are user-friendly and accessible to researchers, while also being flexible and offering customization options.
-
Ensure that the AI tool complies with the relevant regulatory requirements, data privacy, and security standards.
-
Evaluate and confirm that the AI tool can scale seamlessly to manage evolving and growing volumes of data, and computational demands.
in the CLD process, offering scalable storage and processing power. Laboratory information management systems (LIMS) are also essential and come into play at the very beginning when samples are logged and tests are scheduled, and again at the end, to manage the collection, storage, and reporting of results.
What does AI bring to the table?
The incorporation of AI and ML tools in CLD sets the stage for unprecedented efficiency and precision in the creation of novel cell lines for various applications. AI and ML tools can consume vast amounts of genomic and phenotypic data, and help predict the behavior of cells, thus enabling researchers to identify optimal cell lines with the desired characteristics.
Through predictive modeling, AI algorithms can reduce as well as minimize resource loss while accelerating the CLD process. Learning from experimental data, ML algorithms
can help researchers improve and refine their workflow strat- egies, and consequently, facilitate innovations and break- throughs. By enabling researchers to review recommenda- tions and suggestions remotely, AI tools set the pace in a collaborative and coordinated approach which frees time for researchers to focus on other tasks. A representative example is the use of AI-driven decision-making concerning imaging. Innovations in ML and computer vision provide for accurate quantification of cellular characteristics, such as morphology, which is crucial for cell culture growth and development.
By leveraging advanced analytics, predictive modeling, automation, and decision support, AI tools elevate the capa- bilities of LIMS systems. Applying these tools in the CLD workflow empowers labs to generate comprehensive reports
and conduct thorough analyses of data. This capability is invaluable for presenting findings, sharing insights with stakeholders, and supporting decision-making.
The path forward
Combining lab automation, digital solutions, and AI tools appears to be the path toward faster and more efficient outcomes. Lab automation increases operational efficiency, digital solutions provide the necessary infrastructure for data management, and analytics tools offer the deep insights needed for informed decision-making. This integrated approach supports research progress while addressing issues related to resources, human errors, turnaround times, redun- dancies, and data inconsistencies.
Thermo Fisher Scientific is a leading global provider of scientific research and laboratory services, catering to a wide range of industries including healthcare, pharmaceuticals, biotechnology, and academia. With a strong commitment to enabling their customers to make the world healthier, cleaner, and safer, Thermo Fisher Scientific offers a diverse portfolio of innovative products, technologies, and services. Their offerings include analytical instruments, reagents, consumables, software, and services for life sciences research, diagnostics, and applied markets. By combining cutting-edge technology, expertise, and a customer-centric approach, Thermo Fisher Scientific empowers scientists and researchers to accelerate scientific discovery, enhance productivity, and drive advancements in healthcare and beyond.
www.thermofisher.com/us/en/home.html
In partnership with