Leveraging edge artificial intelligence for sustainable agriculture
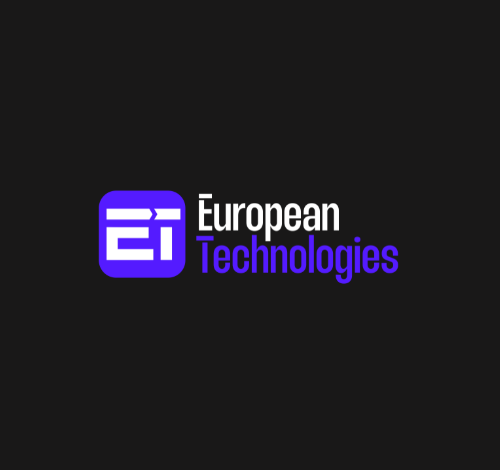
Tilman, D., Balzer, C., Hill, J. & Befort, B. L. Global food demand and the sustainable intensification of agriculture. Proc. Natl Acad. Sci. USA 108, 20260–20264 (2011).
The State of Food and Agriculture 2019. Moving Forward on Food Loss and Waste Reduction (FAO, 2019).
Chhogyel, N. & Kumar, L. Climate change and potential impacts on agriculture in Bhutan: a discussion of pertinent issues. Agric. Food Secur. 7, 79 (2018).
Davis, K. F. et al. Tropical forest loss enhanced by large-scale land acquisitions. Nat. Geosci. 13, 482–488 (2020).
Muluneh, M. G. Impact of climate change on biodiversity and food security: a global perspective—a review article. Agric. Food Secur. 10, 36 (2021).
What is agrobiodiversity? FAO https://www.fao.org/fileadmin/templates/soilbiodiversity/Downloadable_files/agrobiodivesity.pdf (2004).
Byerlee, D., Stevenson, J. & Villoria, N. Does intensification slow crop land expansion or encourage deforestation? Glob. Food Secur. 3, 92–98 (2014).
UN-Water Blueprint for Acceleration: Sustainable Development Goal 6 Synthesis Report on Water and Sanitation 2023 (United Nations Publications, 2023).
Rosa, L. et al. Energy implications of the 21st century agrarian transition. Nat. Commun. 12, 2319 (2021).
Giller, K. E. et al. The future of farming: who will produce our food? Food Secur. 13, 1073–1099 (2021).
Raymond, C. et al. Increasing spatiotemporal proximity of heat and precipitation extremes in a warming world quantified by a large model ensemble. Environ. Res. Lett. 17, 035005 (2022).
White, K., Habib, R. & Hardisty, D. J. How to SHIFT consumer behaviors to be more sustainable: a literature review and guiding framework. J. Mark. 83, 22–49 (2019).
Hallak, R., Onur, I. & Lee, C. Consumer demand for healthy beverages in the hospitality industry: examining willingness to pay a premium, and barriers to purchase. PLoS ONE 17, e0267726 (2022).
Hughner, R. S., McDonagh, P., Prothero, A., Shultz, C. J. II & Stanton, J. Who are organic food consumers? A compilation and review of why people purchase organic food. J. Consum. Behav. 6, 94–110 (2007).
Rockström, J. et al. Sustainable intensification of agriculture for human prosperity and global sustainability. Ambio 46, 4–17 (2017).
Vial, G. Understanding digital transformation: a review and a research agenda. J. Strat. Inf. Syst. 28, 118–144 (2019).
Sharma, R., Kamble, S. S., Gunasekaran, A., Kumar, V. & Kumar, A. A systematic literature review on machine learning applications for sustainable agriculture supply chain performance. Comput. Oper. Res. 119, 104926 (2020).
Yamakami, T. An experimental implementation of an edge-based AI engine with edge-cloud coordination. In 2018 18th International Symposium on Communications and Information Technologies (ISCIT) 442–446 (IEEE, 2018).
McCarthy, J. Programs with common sense. In Proc. Teddington Conference on the Mechanization of Thought Processes 75–91 (Her Majesty’s Stationary Office, 1959).
Samuel, A. L. Some studies in machine learning using the game of checkers. IBM J. Res. Dev. 3, 210–229 (1959).
Dechter, R. Learning while searching in constraint-satisfaction-problems. In Proc. Fifth AAAI National Conference on Artificial Intelligence 178–183 (AAAI Press, 1986).
Liu, X. et al. RENO: a high-efficient reconfigurable neuromorphic computing accelerator design. In Proc. 52nd ACM/EDAC/IEEE Design Automation Conference (DAC) 1–6 (2015).
Moradi, S., Qiao, N., Stefanini, F. & Indiveri, G. A scalable multicore architecture with heterogeneous memory structures for dynamic neuromorphic asynchronous processors (DYNAPs). IEEE Trans. Biomed. Circuits Syst. 12, 106–122 (2018).
Akopyan, F. et al. TrueNorth: design and tool flow of a 65 mW 1 million neuron programmable neurosynaptic chip. IEEE Trans. Comput. Aided Des. Integr. Circuits Syst. 34, 1537–1557 (2015).
Davies, M. E. et al. Loihi: a neuromorphic manycore processor with on-chip learning. IEEE Micro 38, 82–99 (2018).
Frenkel, C., Legat, J. D. & Bol, D. MorphIC: a 65-nm 738k-synapse/mm2 quad-core binary-weight digital neuromorphic processor with stochastic spike-driven online learning. IEEE Trans. Biomed. Circuits Syst. 13, 999–1010 (2019).
Gebregiorgis, A. et al. Tutorial on memristor-based computing for smart edge applications. Memories 4, 100025 (2023).
Brown, T. B. et al. Language models are few-shot learners. In Proc. 34th International Conference on Neural Information Processing Systems (eds Larochelle, H. et al.) 1877–1901 (Curran Associates, 2020).
Patterson, D. et al. The carbon footprint of machine learning training will plateau, then shrink. In Computer Vol. 55, 18–28 (IEEE Computer Society, 2022).
Hamdioui, S. et al. Memristor for computing: myth or reality? In Design, Automation & Test in Europe Conference & Exhibition (DATE) 722–731 (IEEE, 2017).
Singh, A. et al. Low-power memristor-based computing for edge-AI applications. In Proc. IEEE International Symposium on Circuits and Systems (ISCAS) 1–5 (IEEE 2021).
Singh, A. et al. CIM-based robust logic accelerator using 28 nm STT-MRAM characterization chip tape-out. In Proc. IEEE 4th International Conference on Artificial Intelligence Circuits and Systems (AICAS) 1–5 (IEEE, 2022).
Xu, Y. et al. Artificial intelligence: a powerful paradigm for scientific research. Innovation https://doi.org/10.1016/j.xinn.2021.100179 (2021).
Leroux, S., Simoens, P., Lootus, M., Thakore, K. & Sharma, A. TinyMLOps: operational challenges for widespread edge AI adoption. In Proc. IEEE International Parallel and Distributed Processing Symposium Workshops (IPDPSW) 1003–1010 (IEEE, 2022).
Kakani, V., Nguyen, V. H., Kumar, B. P., Kim, H. & Pasupuleti, V. R. A critical review on computer vision and artificial intelligence in food industry. J. Agric. Food Res. 2, 100033 (2020).
Ninomiya, S. High-throughput field crop phenotyping: current status and challenges. Breed. Sci. 72, 3–18 (2022).
Cisternas, I., Velásquez, I., Caro, A. & Rodríguez, A. Systematic literature review of implementations of precision agriculture. Comput. Electron. Agric. 176, 105626 (2020).
Zarco-Tejada, P. J. et al. Previsual symptoms of Xylella fastidiosa infection revealed in spectral plant-trait alterations. Nat. Plants 4, 432–439 (2018).
Pérez-Ruíz, M., Slaughter, D. C., Fathallah, F. A., Gliever, C. J. & Miller, B. J. Co-robotic intra-row weed control system. Biosyst. Eng. 126, 45–55 (2014).
Gázquez, J. A., Castellano, N. N. & Manzano-Agugliaro, F. Intelligent low cost telecontrol system for agricultural vehicles in harmful environments. J. Clean. Prod. 113, 204–215 (2016).
Akhavizadegan, F., Ansarifar, J., Wang, L., Huber, I. & Archontoulis, S. V. A time-dependent parameter estimation framework for crop modeling. Sci. Rep. 11, 11437 (2021).
van Klompenburg, T., Kassahun, A. & Catal, C. Crop yield prediction using machine learning: a systematic literature review. Comput. Electron. Agric. 177, 105709 (2020).
Bock, C. H., Barbedo, J. G. A., Del Ponte, E. M., Bohnenkamp, D. & Mahlein, A.-K. From visual estimates to fully automated sensor-based measurements of plant disease severity: status and challenges for improving accuracy. Phytopathol. Res. 2, 9 (2020).
Dandrifosse, S., Bouvry, A., Leemans, V., Dumont, B. & Mercatoris, B. Imaging wheat canopy through stereo vision: overcoming the challenges of the laboratory to field transition for morphological features extraction. Front. Plant Sci. https://doi.org/10.3389/fpls.2020.00096 (2020).
Ye, Y., Bruzzone, L., Shan, J., Bovolo, F. & Zhu, Q. Fast and robust matching for multimodal remote sensing image registration. IEEE Trans. Geosci. Remote Sens. 57, 9059–9070 (2019).
Carlier, A., Dandrifosse, S., Dumont, B. & Mercatoris, B. Comparing CNNs and PLSr for estimating wheat organs biophysical variables using proximal sensing. Front. Plant Sci. https://doi.org/10.3389/fpls.2023.1204791 (2023).
Raj, M. et al. A survey on the role of Internet of Things for adopting and promoting Agriculture 4.0. J. Netw. Comput. Appl. 187, 103107 (2021).
Tardaguila, J., Stoll, M., Gutiérrez, S., Proffitt, T. & Diago, M. P. Smart applications and digital technologies in viticulture: a review. Smart Agric. Technol. 1, 100005 (2021).
Khanna, A. & Kaur, S. Evolution of Internet of Things (IoT) and its significant impact in the field of precision agriculture. Comput. Electron. Agric. 157, 218–231 (2019).
Bao, J. & Xie, Q. Artificial intelligence in animal farming: a systematic literature review. J. Clean. Prod. 331, 129956 (2022).
Yépez-Ponce, D. F., Salcedo, J. V., Rosero-Montalvo, P. D. & Sanchis, J. Mobile robotics in smart farming: current trends and applications. Front. Artif. Intell. https://doi.org/10.3389/frai.2023.1213330 (2023).
Duong, L. N. K. et al. A review of robotics and autonomous systems in the food industry: from the supply chains perspective. Trends Food Sci. Technol. 106, 355–364 (2020).
Food robotics: global market unit volume 2020–2030. Statista https://www.statista.com/statistics/1290022/food-robotics-global-market-unit-volume/ (2022).
Zhou, Z. et al. Edge intelligence: paving the last mile of artificial intelligence with edge computing. Proc. IEEE 107, 1738–1762 (2019).
Lin, Y. H. et al. Performance impacts of inalog ReRAM non-ideality on neuromorphic computing. IEEE Trans. Electron Devices 66, 1289–1295 (2019).
Mohanan, V., Budiarto, R. & Aldmour, I. Powering the Internet of Things with 5G Networks (IGI Global, 2017).
Tzachor, A., Devare, M., King, B., Avin, S. & Ó hÉigeartaigh, S. Responsible artificial intelligence in agriculture requires systemic understanding of risks and externalities. Nat. Mach. Intell. 4, 104–109 (2022).
Wilkinson, M. D. et al. The FAIR guiding principles for scientific data management and stewardship. Sci. Data 3, 160018 (2016).
Silvestro, D., Goria, S., Sterner, T. & Antonelli, A. Improving biodiversity protection through artificial intelligence. Nat. Sustain. 5, 415–424 (2022).
Deichmann, U., Goyal, A. & Mishra, D. Will digital technologies transform agriculture in developing countries? Agr. Econ. 47, 21–33 (2016).
Blount-Dorn, K., Detroit Food Policy Council and Lindsey Scalera, Ecology Center Modeling an Equitable Michigan Food System (Michigan State Univ., 2018); https://www.canr.msu.edu/news/modeling-an-equitable-michigan-food-system