Machine Learning AI Model Accurately Predicts HIV Incidence in Patients With STIs
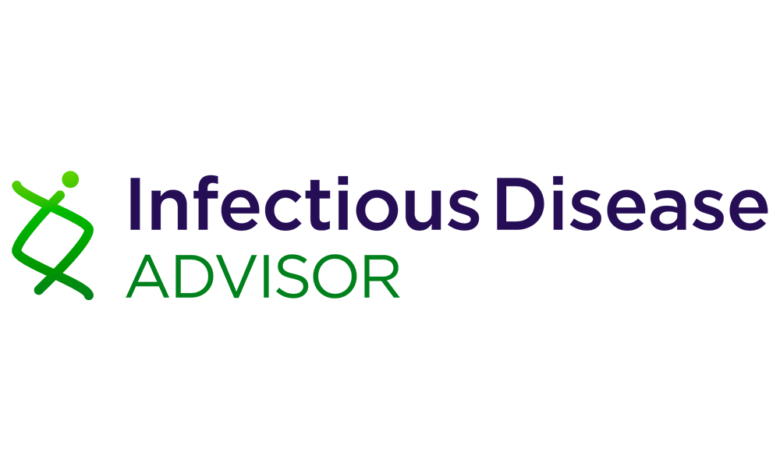
A machine learning model was found to predict incident HIV infection in patients with sexually transmitted infections (STIs) with a high degree of accuracy, indicating the potential use of such models toward the development of tailored interventions for HIV prevention. These findings were published in Clinical Infectious Diseases.
Researchers conducted a study to assess the accuracy of an artificial intelligence (AI)-based machine learning model for predicting the incidence of HIV infection. Data for this study were sourced from the State Electronic Notifiable Disease Surveillance System and Electronic HIV/AIDS Reporting System for Fulton County in Georgia. The analysis included patients aged 13 years and older who were evaluated for sexually transmitted infections (STIs) and HIV coinfection between 2010 and 2021. Patients with STIs were matched 1:1 on the basis of positive vs negative HIV status. The training and validation datasets used for the AI-based machine learning model comprised information from both groups.
A total of 127,169 STI episodes were included in the analysis, representing 85,224 unique patients (female sex, 54%). Among all patients, 2027 developed HIV infection during the study period, the majority of whom were men or boys (84%).
Of men and women with STIs, the mean age at diagnosis was 28 and 24 years, 63% and 57% were Black, and 71% and 72% had a single documented STI episode, respectively.
“
By accurately identifying individuals at heightened risk of HIV acquisition, these models could become valuable tools for developing strategies prioritizing individuals for HIV testing and prevention.
The machine learning model identified 203 true negative and 203 true positive HIV diagnoses among men and 38 true negative and 40 true positive HIV diagnoses among women. Overall, the model was associated with high rates of precision and recall in both men and women (80% and 78% vs 80% and 81%, respectively).
Further analysis showed that the most informative predictors of HIV infection among men were age at STI diagnosis, previous non-HIV STI episode, provider type, number of previous non-HIV STI episodes, reinfection interval, and housing and transportation status. For women, the most informative predictors of HIV infection were age at STI diagnosis, ethnicity, previous non-HIV STI, provider type, household composition, and minority group status.
Limitations of this study include potentially low generalizability among rural populations. Moreover, the use of AI-based machine learning models to predict HIV incidence relies on the accuracy and completeness of its datasets.
“By accurately identifying individuals at heightened risk of HIV acquisition, these models could become valuable tools for developing strategies prioritizing individuals for HIV testing and prevention,” the researchers noted.
Disclosures: One study author declared affiliations with biotech, pharmaceutical, and/or device companies. Please see the original reference for a full list of disclosures.