Money talks in the competition of large language models
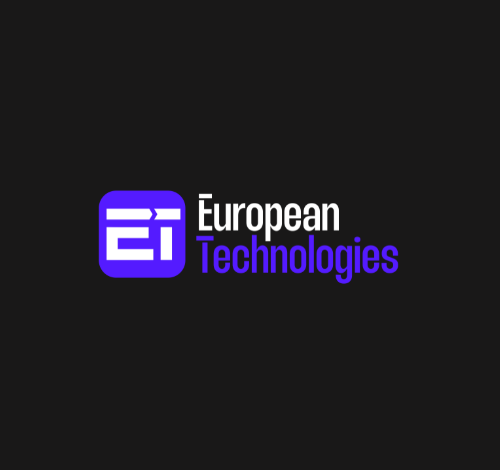
Amid the fierce jostling among large language models and generative AI platforms in terms of applications and functions, the real battle is far more grounded: funding. Zaobao journalist Li Yaning speaks to industry experts to find out more.
Recently, huge waves have been sweeping through the world of generative AI.
On 13 May, OpenAI launched GPT-4o, a large multimodal model (LMM) that can understand and generate text, voice and images; the next day, Google released its own model, Gemini 1.5 Pro, which gives Google Search the capability of complex reasoning. By 15 May, China’s ByteDance announced the low-cost Doubao AI model, which it touted as being 99% cheaper than other models on the market.
According to forecasts by think tank Bloomberg Intelligence, the global GenAI market will reach US$1.3 trillion by 2032. As GenAI relies on large models for its generative capability, the battle of GenAI is in fact a battle between large models.
The most widely used among these is Large Language Models (LLMs).
Funds are coming in to put a stake on the future of LLMs. To date, Microsoft has invested more than US$13 billion on OpenAI; Amazon upped its investments in OpenAI rival Anthropic by US$2.75 billion; Meta announced in its first quarter earnings report that it plans to spend billions more on AI; and Google announced in April that it will invest US$3 billion in building a data centre.
GenAI megatrend is likely to be the greatest megatrend of the decade and it will prove to be structural – Tan De Jun, research and portfolio manager, FSMOne.com
All of the tech giants have seen their share prices grow by 10% to 40% this year, while leading chipmaker Nvidia, whose product forms the basis of AI, saw its stock price soar by nearly 90% recently.
Tan De Jun, research and portfolio manager at FSMOne.com, said companies like Amazon, Meta, Microsoft, and Google have all raised their capital spending plans on AI substantially, a trend that will likely carry into the years ahead. He also said the GenAI megatrend is likely to be the greatest megatrend of the decade and it will prove to be structural.
Outside of the tech giants, the birth of ChatGPT has also caused a wave of ventures into AI. According to data from CrunchBase, AI start-up companies around the world have raised nearly US$50 billion in 2023. Among these, 70 funding rounds for AI-related companies raised a total of more than US$100 million.
But just one year on, the market is very different. Faced with the high cost of computing power, the question of profitability, and the withdrawal of investment, worries are mounting: will the LLM competition become a game for the biggest players only?
Burning through money is almost ingrained in the DNA of LLMs. The performance of the model is largely dependent on the number of parameters; the more parameters there are, the higher the computing cost.
Yang You, a presidential young professor at the School of Computing at National University of Singapore (NUS), observed that the barriers of entry for entrepreneurship in large models were extremely high. “If you don’t have US$200 to 300 million, it’s very hard to start,” he said. “If you look at the companies doing large models in the US, Europe and China, this is always the case.”
Burning through money is almost ingrained in the DNA of LLMs. The performance of the model is largely dependent on the number of parameters; the more parameters there are, the higher the computing cost.
… the cost of electricity is a mere fraction of the cost of processing chips.
ChatGPT searches use 15 times more power than Google
According to an exposé last year by US-based SemiAnalysis, ChatGPT has 1.8 trillion parameters, and costs US$63 million to train. The sum includes only computer costs and does not include testing and failed training, as well as labour and other costs.
Daily operations also require a huge amount of computing power. You Yang told Lianhe Zaobao that a single ChatGPT search uses 15 times more computing power than a Google search. The New Yorker also previously reported that ChatGPT used more than 500,000 kw of electricity per hour — the equivalent of 17,000 American households.
However, the cost of electricity is a mere fraction of the cost of processing chips. Lu Jianfeng, chairman and co-founder of Singapore-based AI company WIZ.AI, said, “[Mark] Zuckerberg said Meta requires 600,000 H100 of computing power, for OpenAI this could be 1 million pieces. Outside of these top companies, it’s very hard for anyone else to start from nothing to produce a general-use large model.”
H100 refers to the high performance graphic processing unit (GPU) Nvidia launched in 2022 designed for AI and high-performance computation tasks. There is no official retail price for the H100, but they are going for nearly US$40,000 each on eBay.
High costs naturally bring about questions of profitability. Figures show that the economic benefits generated by large models are currently far below investments.
According to a report by Grand View Research, the global LLM market reached US$4.35 billion in 2023. In contrast, data from CrunchBase showed that AI start-ups worldwide raised a total of nearly US$50 billion in financing last year, of which some US$18 billion went to just three US companies: OpenAI, Anthropic, and Inflection AI.
You Yang pointed out that with the high cost of computing power, with existing architecture and hardware technology, even if companies started charging users, general-use LLMs such as ChatGPT would still be vastly unprofitable. This might also be the reason OpenAI wants to produce its own chip – to lower costs.
Several media outlets had previously reported that OpenAI was planning to invest billions to build a chip fabrication plant.
But You remains confident of the profitability prospects of LLMs over the long term. “Although they won’t be profitable within the next few years, as technology progresses, over the long term the top LLMs will stop losing money at the very least, because there are indeed so many uses for it.”
Yeap Jun Rong, market strategist at IG, said GenAI may still have a way to go before “actual monetisation”, but stable tech companies who have abundant resources and huge access to data may emerge as winners.
Clearly, short-term profit is not what the tech titans are after. What they are betting on is the longer-term future — artificial general intelligence (AGI), which is AI that will truly reach or even surpass human-level intelligence. Mark Zuckerberg has also said that AGI is the company’s “long term vision”.
Yann LeCun, chief AI scientist at Meta, once said, “If you think AGI is in, the more GPUs you have to buy.” He likened the AI competition to a war, and GPU to weapons. And Nvidia CEO Jensen Huang is the one supplying the weapons.
More financing challenges facing AI start-ups
While the tech giants have a full armoury, most start-ups do not have the luxury of overlooking commercial challenges. As the initial wave of enthusiasm, financing has becoming more difficult.
You Yang observed that compared to last year, funds are quite clearly more cautious this year. “Among the top venture capitalists in the US and China, many will only go in at the series A or B funding round, after that they stop,” he said. “Some large model companies become unicorns (start-ups that reach a valuation of US$1 billion) after the angel round, but are not able to commercialise or get listed, and investors have to take into account returns on investment.”
If 2023 was when a great tidal wave swept through the market, this year could herald rationality after the tide ebbs. Statistics show that a start-up needs either strong commercial ability or outstanding technology to survive, otherwise investors will quickly drop them.
LLMs that specialise in certain sectors or have specific capabilities are turning out to be the only viable path for the majority of small companies. Most of such LMs are used in enterprise services.
US-based media channel The Information reported that Jasper, a company which uses AI to generate ad copy, cut its internal valuation by 20%. Interest in Character.AI, CopyAI and other AI companies that base their business on packaging third-party models is also dwindling.
In February, Chinese AI company Emotibot announced it was restructuring due to cash flow issues, and halted some of its operations. The one-time market darling had managed to raise over RMB 1 billion (US$138 million) in funding, but the sum has proven inadequate for it to become a contender in the LLM field.
Specialised AI companies search out new paths
Dimitry Smirnov, founder of venture capital firm Flint Capital, wrote in his column that investments in AI start-ups have not completely died out, but it has become harder for ordinary start-ups to get a look-in.
Niko Bonatsos, managing director at General Catalyst, another venture capital firm, was also of the opinion that AI start-ups were facing funding challenges. However, AI is undergoing very rapid development and with technological advances, some costs will also decrease.
“I think the next set of algorithms should be more efficient, so you’ll need less computer power,” he said. “Also, there will be more open source. So the cost of starting a company will go down.”
Lu Jianfeng, of WIZ.AI, said with the arrival of ChatGPT, large model start-ups were at “peak potential”, and from the second half of last year to the present, they have come to a “valley of hopelessness”.
In his view, the hopelessness is due to having to face the crushing competition from leading tech companies. “For VCs looking at an AI company, they are all thinking, what if OpenAI starts making the same product, so the valuation of these companies has changed. Investments in consumer-facing businesses are trending towards rationality.”
However, he feels that AI companies targeting businesses and other verticals have it better: they are seeing more opportunities and it has not become an environment where the winner takes it all.
“If a large model is trained for specific scenarios, with a volume and cost that’s only 1% of OpenAI, you’ll see profits very quickly… For example, using a large model to accelerate R&D in pharmaceutical drugs, or helping oil companies to find oil.” — Presidential Young Professor You Yang, School of Computing, NUS
With computing resources as a limiting factor, LLMs that specialise in certain sectors or have specific capabilities are turning out to be the only viable path for the majority of small companies. Most of such language models are used in enterprise services.
You Yang pointed out that compared to general-use large models, vertical large models are able to turn a profit more quickly. “If a large model is trained for specific scenarios, with a volume and cost that’s only 1% of OpenAI, you’ll see profits very quickly,” he said. “For example, using a large model to accelerate R&D in pharmaceutical drugs, or helping oil companies to find oil.”
PatSnap, a Singaporean tech intelligence unicorn, is one such example. PatSnap co-founder Guan Dian said that compared with the general-use large models of OpenAI and Anthropic, PatSnap’s LLM goes deeper and is more precise in the patents sector.
She feels that this is a chance for the LLM era. “For the tech giants, vertical application markets are smaller, so they won’t come in to compete with you, and you will have the space to grow. If you really focus on understanding your customers and the scenarios, you can go further in-depth and build up entry barriers.”
WIZ.AI is based on open-source models, and the company has incorporated Indonesian languages and trained the first Indonesian LLM in Southeast Asia for use in customer service. Lu Jianfeng said small companies are not looking to rise to the zenith of technology; instead, they are looking at increasing efficiencies. “It’s more putting new wine into old bottles, and the new wine tastes a lot better too.”
The sensitivity of smaller companies to computing costs is also a pain point in the implementation of LLMs. Lu said the larger the model, the higher the cost of inference. If a scenario requires only basic knowledge, an overly large model would not be economic, so these factors need to be taken into consideration in actual operations.
… if you have a model that is best suited to the languages and industries of Southeast Asia that can offer value across different sectors and industries, it will also attract high-end talent, like Falcon, which was launched in the Middle East.” — You
The US is leading while Europe and China play catch up
In the competitive field, it is not just the differences between companies that matter — the gaps between countries have also become obvious. Both at the very top and at the level of start-ups, the US is the clear leader. Among the top ten AI companies in 2023 in terms of capital raised, eight were from the US, and the other two from Europe.
Geopolitics is also affecting this war. Back in 2022, the US government started to ban Nvidia from exporting its high-end A100 and H100 chips to China, hampering China’s competitiveness in the field of AI.
You Yang said China and Europe are currently playing catch up, which is vital. “Especially for countries like China, who are emphasising autonomy and control, they will definitely develop an independent large model, otherwise the risk is just too great.”
He said small countries like Singapore will also need to develop their own LLMs with tens of billions of parameters. “It’s not about competing with OpenAI; if you have a model that is best suited to the languages and industries of Southeast Asia that can offer value across different sectors and industries, it will also attract high-end talent, like Falcon, which was launched in the Middle East.”
In 2023, the United Arab Emirates launched its own LLM with 40 billion parameters which included a chatbot, customer service functions, virtual assistant, translation, content generation, emotion analysis and other uses. Falcon showed outstanding performance, even outperforming OpenAI and Google’s models in some aspects.
Southeast Asian LLM launched by Singapore last year
Last year, Singapore launched Sea-Lion, an LLM tailored for Southeast Asia, with two versions based on 3 billion and 7 billion parameters respectively. Last December, when Singapore announced its AI Strategy 2.0, it included an investment of $70 million over two years to build an LLM, based on Sea-Lion, with 30 billion to 50 billion parameters.
You Yang said Singapore should raise its computing capability, as it is a limiting factor in creating synergies.
In addition, Singaporean LLM start-ups are smaller, and small start-ups are known to achieve technological breakthroughs more efficiently than research institutions. At present, start-up companies in the field are concentrated in the US, China, and Europe, so Singapore should also look into developing the sector.
What is a Large Language Model?
A LLM is a language model made up of an artificial neural network with numerous parameters.
You Yang explained: “The human brain consists of many neurons, joined up with over 100 trillion links, enabling us to think and learn. The parameters in large models can be likened to the links between neurons, with a whole architecture resembling the neurons in a human brain, LLMs can start to learn all the knowledge that is on the internet.”
He said the underlying principle of LLM is predicting the probability of the next word or phrase based on existing script, to generate output.
The world “large” in LLM refers to the number of parameters. Starting from the large scale predictive training language model BERT launched by Google in 2018, to OpenAI’s GPT-2, GPT-3, and GPT-4 and Google’s PaLM and Gemini, to Anthropic’s Claude — in just a few short years, the scale of parameters has gone from billions to trillions, setting off this round of revolution in generative AI.
It has often been thought of as a numbers game: the greater the number of parameters, the better the generative capability of the model. Just as the more neural links there are, the more human-like AI will become, as its capacity for reason and inference becomes stronger. The ability to reason and infer cause and consequence is of vital importance to AI systems, and the arrival of LLM has enabled artificial intelligence to be put into general use.