Multimodal Generative AI Guide: Unlocking Creativity!
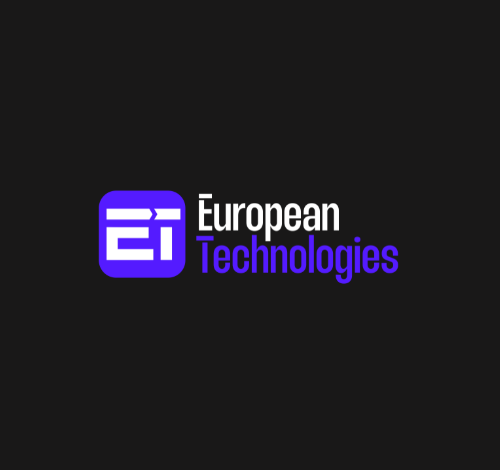
Multimodal generative AI is a cutting-edge frontier where technology meets creativity. As we delve into artificial intelligence, which not only understands but also integrates and generates content across multiple forms of data, we uncover limitless possibilities for innovation. This guide introduces multimodal generative AI’s capabilities, applications, and transformative potential. Whether you’re a creative professional looking to push the boundaries of art and design, a developer eager to build more intuitive and engaging AI systems, or simply a tech enthusiast curious about the next big thing in AI technology, this article will provide you with a comprehensive overview of how multimodal generative AI is reshaping the landscape of creativity.
What Is Multimodal Generative AI?
Multimodal generative AI refers to artificial intelligence systems that can understand and generate outputs across multiple types of data or modes, such as text, images, audio, and video. These systems are designed to process and integrate information from these different modalities to perform tasks requiring a holistic understanding of multiple input forms. Here are some key points about multimodal generative AI:
- Cross-Modal Understanding: Multimodal AI can interpret and relate information across different modalities. For example, it can understand a written description and generate a corresponding image, or vice versa.
- Generative Capabilities: Generative Capabilities: Unlike models that only analyze or classify data, multimodal generative AI can create or generate new content. For example, it can compose music based on a series of images, synthesize speech from text, or generate video from a script.
- Applications: This technology has many applications, including content creation, educational tools, accessibility technologies, entertainment, and more. For instance, it can generate realistic virtual reality simulations, provide automatic dubbing of videos into different languages, or create personalized learning experiences in educational software.
- Complex Interactions: These systems often require sophisticated architectures to manage and translate between different data types. Techniques such as transformers and neural networks are commonly used to handle the complexity of learning patterns and relationships within and between different modalities.
- Challenges: Multimodal generative AI faces challenges like ensuring coherence between modalities, maintaining accuracy and relevancy in generated outputs, and addressing ethical considerations such as content authenticity and privacy concerns.
Multimodal generative AI represents a significant advancement in the ability of machines to interact with the world in a human-like way, recognizing and synthesizing varied types of information to perform complex tasks.
Dive into the world of AI with our Applied Generative AI Specialization course. Whether aspiring to become a prompt engineer or seeking to harness the power of AI in your field, this course offers the knowledge and hands-on experience you need.
Difference Between Generative AI and Multimodal AI
The distinction between generative AI and multimodal AI centers around their capabilities and application areas, even though they often overlap in practice. Here’s a breakdown of the differences:
Generative AI
- Definition: Generative AI refers to artificial intelligence systems capable of creating new content or data that resemble original human-generated data. This includes text, images, music, video, and more.
- Focus: The primary focus is generating novel, realistic, or useful outputs based on learned patterns and data structures from a given dataset.
- Examples: Technologies like GPT (for text generation), DALL-E (for image generation), and StyleGAN (for advanced image manipulations) are typical examples of generative AI.
- Applications: It is used in creative fields to generate art, literature, or music, in business to generate marketing content, in software development to generate code, and in many other domains.
Multimodal AI
- Definition: Multimodal AI refers to AI systems that can process and understand multiple types of input data (modalities) such as text, images, audio, and video. It can integrate information from these different modalities to perform tasks.
- Focus: The focus is on interaction between different data types, understanding cross-modal relationships, and performing integrative tasks that require a holistic view of multiple inputs.
- Examples: Multimodal AI systems could include an AI tutor that interprets a student’s spoken questions and provides relevant visual aids and textual explanations or a system that can analyze video content and generate a descriptive summary in text.
- Applications: Particularly useful in environments where multiple data formats need to be understood or synthesized, such as in automated customer support, educational technology, and content moderation.
Overlap
- Generative Aspect: Multimodal AI can also be generative. For example, it might generate a text description based on a combination of image and audio inputs.
- Usage of AI Techniques: Both multimodal and generative AI may utilize similar underlying AI techniques such as deep learning, neural networks, and transformers to achieve their tasks.
Benefits of Multimodal Generative AI
Multimodal generative AI combines the advantages of generative models with the ability to process and integrate multiple types of data, leading to a range of powerful applications and benefits:
- Enhanced Understanding and Interpretation: By integrating multiple data types (like text, images, and sound), these models can achieve a deeper understanding of content and context than single-mode AI systems. This can lead to more accurate and relevant responses in AI applications, such as virtual assistants, that can understand a query’s verbal and non-verbal components.
- Richer Content Creation: Multimodal generative AI can create more complex and nuanced content by blending elements from different modalities. For example, it can generate a video from a text script, complete with appropriate imagery and sound, or create educational materials that combine visual, textual, and auditory elements to enhance learning.
- Improved Accessibility: These systems can automatically generate alternative forms of content to improve accessibility. For example, they can convert spoken language into text for the hearing impaired or transform written content into audio for the visually impaired.
- Better User Experience: In user interfaces, multimodal generative AI can adapt outputs to suit the user’s needs or the context of use. For example, it could automatically provide visual explanations in a mobile app where audio output is not feasible or enhance interactive gaming experiences by generating realistic characters and dialogues that respond to user inputs in multiple forms.
- Cross-Domain Applications: Multimodal generative AI is versatile and finds applications across many fields. In healthcare, it can analyze medical images and patient histories to assist in diagnosis. In the automotive industry, it can interpret visual and sensor data to enhance the capabilities of autonomous driving systems.
- Automated Content Moderation: By understanding content across multiple modalities, these AI systems can better identify and handle inappropriate or harmful content across platforms that use diverse media forms, such as social media networks featuring videos, images, and text.
- Innovative Marketing and Advertising: Multimodal generative AI can help create personalized advertising content that combines customer data across different modalities. For instance, it can analyze customer interactions through text and images to generate targeted promotional content that resonates on multiple sensory levels.
- Efficient Data Fusion: These systems can effectively merge information from different sources to provide a more comprehensive view of complex situations, which is particularly useful in areas like security, where combining visual, audio, and textual analysis can lead to more effective surveillance and threat detection.
Multimodal Generative AI Adoption
The adoption of multimodal generative AI across various sectors is accelerating due to its ability to integrate and generate content across multiple data types, enhancing operational efficiencies and user experiences. Here are some key sectors and how they are adopting this technology:
1. Healthcare
- Clinical Diagnosis: AI systems that analyze medical imaging data, patient histories, and other modalities can help diagnose diseases more accurately and quickly than traditional methods.
- Patient Interaction: Multimodal systems can interact with patients using verbal and non-verbal cues, improving the quality of virtual healthcare services.
2. Education
- Interactive Learning Tools: These AI systems can create dynamic learning materials that combine text, images, and video to adapt to different learning styles and needs.
- Accessibility Enhancements: They help generate accessible content for students with disabilities, such as converting textbooks into audiobooks or providing sign language interpretations of spoken content.
3. Automotive
- Autonomous Vehicles: Multimodal AI integrates sensor data, visual inputs, and auditory signals to improve the safety and efficiency of autonomous driving systems.
- Enhanced User Interfaces: In-car systems that respond to voice, touch, and visual inputs create a more seamless and intuitive user experience.
4. Retail and E-commerce
- Customer Service: AI chatbots and virtual assistants that understand and generate responses across text, images, and voice improve customer interaction and service.
- Product Recommendations: These systems analyze customer interactions across multiple channels to provide personalized shopping recommendations.
5. Entertainment and Media
- Content Creation: From generating music based on mood and visual themes to creating movie scenes from scripts, multimodal AI is revolutionizing content production.
- Personalized Experiences: Streaming services use multimodal data to tailor content recommendations and user interfaces to individual preferences.
6. Security and Surveillance
- Threat Detection: AI systems that analyze video, audio, and data feeds can identify potential threats more quickly and accurately than human monitors.
- Forensic Analysis: Integrating various data types helps reconstruct events more effectively for investigative purposes.
- Marketing and Advertising
- Ad Creation: Create ads that combine text, images, and video and are tailored to the user’s current engagement context.
- Consumer Insights: Analyze consumer behavior across different platforms to design more effective marketing strategies.
Challenges and Considerations
While the adoption of multimodal generative AI is growing, it also presents several challenges:
- Data Privacy and Security: Handling multiple types of personal data increases the risk and complexity of data privacy issues.
- Bias and Fairness: AI systems can inherit or amplify biases in training data across different modalities.
- Integration Complexity: It is technically challenging to combine data from multiple sources and ensure the system interprets it correctly.
- Regulatory Compliance: Ensuring compliance with evolving regulations concerning AI and data usage across countries and industries.
Future of Multimodal AI
The future of multimodal AI looks highly promising, with continuous advancements likely to expand its capabilities, applications, and impact across various industries. Here are several key trends and developments that might shape the future of multimodal AI:
1. Enhanced Integration and Fusion Techniques
- Improved Data Fusion: Future developments in neural architectures and learning algorithms will enable more sophisticated data integration from different modalities, leading to richer and more accurate interpretations and outputs.
- Cross-Modal Translation: Advances in AI will enhance the ability to translate one modality to another, such as converting images directly into sound or text into video, increasing the accessibility and usability of information.
2. Greater Emphasis on Contextual Understanding
- Context-Aware AI: Multimodal AI systems will become more adept at understanding the context surrounding data inputs, such as recognizing social cues in a conversation or adapting to environmental changes in real time, enhancing interaction quality and decision-making precision.
3. Expansion in Healthcare Applications
- Personalized Medicine: With its ability to analyze diverse data types, multimodal AI could personalize treatment plans based on a deeper understanding of patient records, genetic information, and lifestyle data.
- Robotic Surgery: AI enhancement might lead to more sophisticated robotic systems that use multimodal data to perform complex surgeries with greater precision and adaptability.
4. Improved User Interfaces and Experiences
- Voice and Visual AI Interfaces: As natural language processing and computer vision continue to advance, AI interfaces will become more intuitive and capable of understanding and responding to users with human-like awareness, making technology more accessible and enjoyable.
5. Proliferation in Autonomous Systems
- Smarter Autonomous Vehicles: Multimodal AI could lead to safer and more efficient autonomous vehicles by integrating and interpreting vast amounts of sensor, visual, and auditory data in real time.
- Advanced Drones and Robotics: These systems will increasingly use multimodal AI to navigate complex environments and perform tasks that require a sophisticated understanding of multiple data inputs.
6. Ethical AI Development and Regulation
- Focus on Ethics: As AI systems become more capable and widespread, ethical considerations, particularly concerning privacy, consent, and transparency, will drive the development of more secure and responsible AI technologies.
- Regulatory Frameworks: We can expect more comprehensive regulations focusing on developing and deploying multimodal AI systems to ensure their safe and fair use.
7. AI in Creative Industries
- Film and Music Production: AI could assist in creating music scores, editing films, and even scripting, leveraging its understanding of emotional cues and audience preferences.
- Art and Design: Multimodal AI will further empower artists and designers with tools that enhance creativity and enable new forms of expression by blending different artistic modalities.
8. Global Accessibility and Education
- Language Translation and Learning: Advances in multimodal AI will help break down language barriers more effectively and create educational tools that adapt to the learning preferences of individuals, making education more accessible worldwide.
How Multimodal Generative AI Works?
Multimodal generative AI is a sophisticated technology that combines the capabilities of understanding and generating content across multiple data types, such as text, images, audio, and video. Here’s a breakdown of how these systems typically work, from data input to output generation:
1. Data Input and Preprocessing
- Multimodal Data Collection: The AI system collects data from different modalities. For example, it might gather text descriptions, images, videos, and audio recordings.
- Preprocessing: Each type of data is processed differently. Text may be tokenized into words or sentences, images are resized and normalized, audio is converted into spectrograms or waveforms, and videos are broken into frames.
2. Feature Extraction
- Modality-Specific Processing: Specialized neural networks process each modality:
- Text: Transformer models or recurrent neural networks analyze and encode text.
- Images: Convolutional neural networks are used to extract features from images.
- Audio: Networks like CNNs or RNNs analyze audio frequencies and patterns.
- Video: Video data is handled using a combination of CNNs for spatial processing and RNNs or 3D CNNs for temporal processing.
- Feature Extraction: These networks convert raw data into a high-dimensional space where similar features are represented in ways that the models can easily process.
3. Fusion and Integration
- Early Fusion: In some models, data from all modalities is combined at the beginning of the process. This approach is useful when integrating all data types from the start, as it can enhance learning.
- Late Fusion: In other models, data from each modality is processed separately through its network, and the features are only combined later, usually before the final decision or output layer.
- Hybrid Approaches: Some systems use a mix of early and late fusion, integrating some modalities early and others later, depending on the task requirements.
4. Generative Modeling
- Training Generative Models: The system trains on the integrated multimodal data using generative models like Generative Adversarial Networks (GANs), Variational Autoencoders (VAEs), or autoregressive models like Transformers.
- Learning to Generate: These models learn to generate new content that mimics the training data in style, structure, and modality. They might learn to produce a coherent output that combines multiple input types, such as generating a video from a script or creating a sound that matches a picture.
5. Output Generation
- Decoding and Synthesis: The generative model outputs new data in the desired form, converting the learned representations into readable or perceptible formats, such as text, images, or sounds.
- Cross-modal Outputs: Depending on the application, the output might involve translating or transforming content from one modality to another (e.g., text to image).
6. Evaluation and Refinement
- Performance Evaluation: Outputs are evaluated against benchmarks or through user feedback to measure the system’s accuracy, relevance, and realism.
- Model Refinement: Based on feedback and performance, the model undergoes further training and tuning to improve its outputs and handle a broader range of scenarios.
Elevate your career and harness the power of AI with our Generative AI for Business Transformation course. Don’t miss this opportunity to transform your understanding of generative AI and its applications in the business world.
Challenges in Multimodal Generative AI
- Data Integration Complexity: Effectively integrating data from different modalities can be technically challenging, requiring sophisticated algorithms to ensure the data is combined in a meaningful way that preserves context.
- Quality and Consistency of Generated Content: Ensuring the quality and consistency of content generated from diverse input types is difficult, as the system must maintain coherence and relevance across modalities.
- Scalability: Processing and analyzing large datasets from multiple modalities demands extensive computational resources, making scalability a critical issue.
- Bias and Fairness: AI models can inadvertently learn and perpetuate biases present in training data, which can be particularly problematic when handling diverse forms of data.
- Data Scarcity and Annotation: Obtaining sufficient annotated multimodal data for training can be expensive and time-consuming. The lack of labeled data for certain modalities limits the effectiveness of these AI systems.
- Privacy and Security: Handling sensitive data across multiple modalities increases the risk of privacy breaches and poses significant security challenges.
- Ethical and Societal Implications: As with other AI technologies, there are concerns about the ethical use of multimodal generative AI, including issues related to misinformation, deepfakes, and the potential replacement of human jobs.
- Interpretability and Explainability: Multimodal models, often based on complex neural networks, can be opaque, making it difficult to understand decisions, which is crucial for trust and accountability.
- Regulatory Compliance: Adhering to diverse and evolving regulations concerning data protection and AI across different jurisdictions can be complicated, especially when multiple data types are involved.
- Technical Heterogeneity: Different data types require different processing techniques, leading to inconsistencies in how data is handled and integrated within the system.
Conclusion
Multimodal generative AI represents a frontier in technological advancement that promises to reshape how we interact with and harness technology across various sectors. By understanding and utilizing this powerful tool, professionals and creatives alike can unlock unprecedented levels of innovation and efficiency. For those looking to delve deeper into the capabilities of generative AI and explore its transformative potential within the business landscape, the Generative AI for Business Transformation course offered by Simplilearn is an excellent resource. This course provides comprehensive insights and practical skills to leverage generative AI effectively in your organization. Embrace the future of AI and enhance your professional toolkit by enrolling today at Generative AI for Business Transformation. Unlock your creative potential and lead the charge in the AI-driven business revolution!
FAQs
1. What is multimodal in machine learning?
Multimodal machine learning involves systems that can process and interpret data from multiple sources or types (modalities), such as text, images, audio, and video. These systems integrate and analyze information across these modalities to enhance understanding and improve decision-making.
2. What are some real-world applications of Multimodal Generative AI?
Real-world applications include healthcare (diagnosing diseases from medical images and patient histories), education (interactive learning materials), entertainment (creating music or films), customer service (AI chatbots that process text and voice), and security (surveillance systems analyzing visual and audio data).
3. Can Multimodal Generative AI improve content creation processes?
Yes, multimodal generative AI can significantly enhance content creation by automating process aspects, ensuring consistency, and generating novel ideas. It can create comprehensive and engaging multimedia content by integrating text, images, and videos, streamlining workflows and boosting creativity.
4. What industries could benefit most from Multimodal Generative AI?
Industries like healthcare, entertainment, automotive, education, and marketing could benefit greatly. These sectors can leverage AI to integrate various data types for better diagnostics, personalized content creation, safer autonomous vehicles, dynamic educational tools, and more targeted marketing strategies.
5. How does Multimodal Generative AI impact the future of machine learning?
Multimodal Generative AI is pushing the boundaries of machine learning by facilitating the development of more sophisticated, adaptive, and intelligent systems. It promotes a deeper integration of AI into daily life and industry, enhancing AI’s ability to understand and interact with the world in a more human-like manner.