Navigating Your Data Science Career: From Learning to Earning
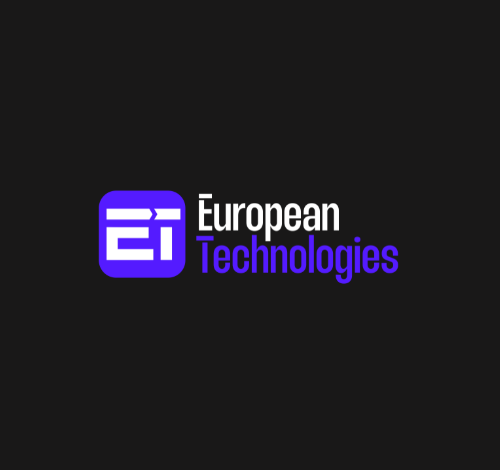
Image by author
With 281 tech companies that laid off 80,628 people, why would you be interested in starting a data science career?
It might seem this is not a good moment, with companies downsizing. Yes, there are layoffs, but the chart below shows recent layoffs are nothing compared to the end of 2022 and the beginning of 2023. So, it’s not that bad!
Source: layoffs.fyi
Another perspective makes it even more positive: companies are still employing data scientists. In fact, in the last month, there have been almost 5,500 job ads on Glassdoor only in the US.
There’s a rather vibrant job market for data scientists. Only now are the companies more demanding. They are looking more for data science specialists than generalists. On top of that, embracing AI tools is what’s now required from data scientists. Here’s how you can approach the challenges and still come on top in the job market.
1. Educational Pathways
There are always two distinct approaches when it comes to learning data science:
- Academic education
- Self-learning
Ideally, you would combine both.
Academic education
Academic education is not necessary to become a data scientist, but it does give you broad and structured knowledge. It’s much easier to build on this knowledge later than to become a data scientist from scratch.
Data scientists usually have a Bachelor’s degree in quantitative fields, such as computer science, statistics, mathematics, or even economics.
Having a master’s degree is an excellent idea to boost your chances of getting a job. With it, you can specialize. Some examples of specializations are machine learning, data analysis, business intelligence, etc.
Going for a PhD is usually unnecessary, except if you’re interested in research-oriented roles in companies or academia.
Self-Learning
You can become a data scientist by creating a curriculum for yourself. This can include anything from the (non-exhaustive) list:
- Certifications
- Online courses
- Bootcamps
- YT videos
- Books
- Blog articles
- Community forums
If time and finances allow, I recommend you focus on certifications, online courses, and bootcamps. Then, complement them with other resources.
Some of the certifications, courses, and bootcamps I suggest are:
2. Skills
A data scientist’s skills can be categorized into technical and soft skills.
Technical Skills
They stem from the main data scientist’s tasks: extracting and manipulating data, building, testing, and deploying ML models.
Data scientists must use various programming languages and tools to put all this knowledge into practice.
Here’s an overview.
This should be your starting point for further specialization. For example, you can specialize in BI tools or focus on data engineering tools, such as Apache Kafka, Apache Spark, Talend, Airflow, etc.
Soft Skills
The technical skills have to be complemented by the soft skills given below.
Communication Skills
These include both listening to others’ thoughts and communicating your own.
Your work as a data scientist starts by listening to other people’s problems. You’re the kind of psychotherapist that helps others solve their problems using data. Data therapist? By understanding business problems, you can shape your technical solution to the users’ needs.
Data scientists also must be able to translate the technical complexity of their work to non-technical audiences. They help themselves with visualization tools, meaning effectively visualizing and presenting your work is mandatory.
Analytical Thinking
Business problems that you need to solve will often be explained to you in a very non-technical way: “Oh, God, our customer retention is bombing! Heeeelp! You, the data science guy, come up with something. ”
This calls for the ability to break down the problem into logical blocks and solve it systematically. Also, creativity needs to be sprinkled around, as many problems require finding novel solutions.
Collaboration Skills
Data scientists’ ideal work day would be to be left alone, work on their models, and talk softly to it (in Gollum’s voice): It is mine, I tell you. My own. My precious. Yes, my precious.
Unfortunately, data scientists very often have to collaborate with other colleagues from data team. Projects also include cross-departmental teams.
Being adaptable and flexible, creating a good working atmosphere, and solving conflicts effectively and respectfully? Yes, my precious!
Project Management
Working on a data science project requires project management ability, including prioritizing tasks, coordinating a project team, and tracking project progress and deadlines.
Add to that mentoring junior staff and juggling between several projects, and this skill becomes crucial.
Business Acumen
All data projects are designed to solve business problems. To make them so, you need to have a solid understanding of your company’s business and industry. This makes it easier to understand the business problem and design a solution considering dependencies that may not have been explicitly mentioned.
3. Career Path and Salary
The data science career usually starts with landing a junior data analyst or junior data scientist job.
From there, I suggest you go into one of the specialization roles. Some of the examples are data engineers, ML engineers, business analysts, data analysts, or BI engineers. The data scientist position today is also increasingly a specialist role – more focused on using statistics in data exploration and initial model development rather than doing end-to-end projects.
Depending on the number of years you spend in a specific specialistic position and your interests, you could go into two distinct directions: management roles or advanced specialization roles.
For example, management roles can include a senior manager or director in any of the specializations mentioned earlier. This path takes you away from the technical part of your job, and managing people and departments becomes your focal point.
The other option is to remain an individual contributor and go even deeper into your specialization. These are advanced specialization roles. For any of the specializations mentioned, the titles are usually Staff, Principal, Distinguished, and Fellow.
4. Salary
Data science is still a very well-paying profession. This shouldn’t be overlooked when choosing your career path. Here’s the overview of the salaries for the previously mentioned roles.
Image by author, source of salary data: Glassdoor
5. Getting a Job
Now, the question is how to transition from learning data science to earning all this money, otherwise known as getting a job.
I wouldn’t say anything new if I said: find the job ads you like, apply, kick ass on the interview, get a job. There you go, you’re welcome!
There are, however, two things that can distinguish you from other applicants:
- An outstanding portfolio
- Experience of the job interviews
An outstanding portfolio means having a solid number of data projects relevant to the job. Data projects are the best way to comprehensively build up and showcase your data science skills, as doing them requires a high level of each skill. Of course, you can also work on specialized projects focusing on specific skills, e.g., machine learning, data engineering, etc.
Experience of the job interviews can be gained in two ways. The first is to fail a lot of interviews before you get a job. This is a legitimate way many of us have experienced. I’m not joking; gaining experience makes you more used to the interview process, approaches, topics tested, and, especially, coding under time pressure.
However, there’s also a less painful way to achieve the same: solving the actual coding and other technical interview questions on the platforms that provide them.
Conclusion
While it might not seem like it, now is the ideal time to get into data science. Two reasons. First, if you’re thinking about starting your data science education, go for it. It will take some time. By the time you finish, data science might again be booming.
Second, if you already have all the requirements, apply for the jobs, as there are plenty of them, despite the layoffs.
Let’s remember that data science is still one of the most attractive jobs there, despite all the shake-ups.
Nate Rosidi is a data scientist and in product strategy. He’s also an adjunct professor teaching analytics, and is the founder of StrataScratch, a platform helping data scientists prepare for their interviews with real interview questions from top companies. Nate writes on the latest trends in the career market, gives interview advice, shares data science projects, and covers everything SQL.