New algorithm helps robots rapidly and reliably learn complex skills
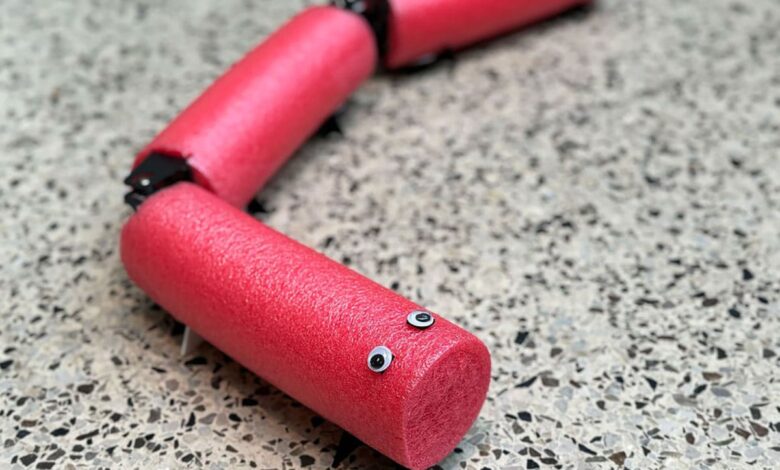
The use of robots has undeniably revolutionized numerous industries, enhancing their efficiency. However, while these machines excel in controlled environments such as manufacturing facilities, they encounter significant challenges in unstructured settings like household spaces.
Unlike in fixed environments, robots operating in unfamiliar or dynamic surroundings must rely on learning algorithms to autonomously complete tasks. Although these algorithms have shown great progress across various applications, they struggle to effectively guide embodied agents such as robots.
A key limitation lies in their assumption of independent data points, which does not hold true as robots interact with their environment over time and space. Consequently, existing robots often prove unreliable when dealing with unstructured environments, highlighting the need for further advancement.
Recently, Northwestern University engineers developed a new artificial intelligence (AI) algorithm called Maximum Diffusion Reinforcement Learning (MaxDiff RL), designed specifically for smart robotics. This algorithm can help robots rapidly and reliably learn complex skills and could significantly improve the practicality and safety of robots for a range of applications.
MaxDiff RL algorithm encourages robots to explore their environments as randomly as possible to gain diverse experiences, improving the quality of data collected. Simulated robots using this algorithm demonstrated faster learning and improved performance.
In tests, robots using MaxDiff RL consistently outperformed state-of-the-art models and were able to learn and successfully perform new tasks within a single attempt. This stands in contrast to current AI models, which rely on slower trial and error learning.
Thomas Berrueta, the lead researcher at Northwestern, shared, “Our robots demonstrated enhanced speed and agility, effectively applying their learning to new situations. This is a significant advantage for real-world applications where robots cannot afford prolonged trial and error. The MaxDiff RL algorithm offers a substantial benefit in these scenarios.”
“This doesn’t have to be used only for robotic vehicles that move around,” said Allison Pinosky, also a Ph.D. candidate. “It also could be used for stationary robots – such as a robotic arm in a kitchen that learns how to load the dishwasher. As tasks and physical environments become more complicated, the role of embodiment becomes even more crucial to consider during the learning process. This is an important step toward real systems that do more complicated, more interesting tasks.”
The adaptability of the MaxDiff RL algorithm makes it suitable for a variety of critical applications, including self-driving cars, delivery drones, household assistants, and automation. However, it is crucial to validate its performance in real-world settings, where it must navigate complex physics and imperfect sensor data. While the researchers have only tested their new algorithm on simulated robots, they built NoodleBot for future testing in the real world.
Journal reference:
- Thomas A. Berrueta, Allison Pinosky & Todd D. Murphey. Maximum diffusion reinforcement learning. Nature Machine Intelligence, 2024; DOI: 10.1038/s42256-024-00829-3