New dbt Labs and Databricks Report Highlights the Evolving Roles of Data Practitioners
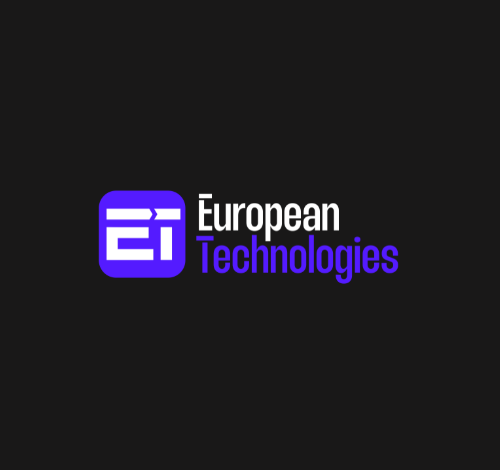
PHILADELPHIA, June 11, 2024 — dbt Labs, a pioneer in analytics engineering, and Databricks, the Data and AI company, unveiled “The Data Practitioner for the AI Era” report. Produced by MIT Technology Review and revealed at the Databricks Data + AI Summit, the findings shed new light on the critical role data practitioners play in managing data pipelines and processes, supporting business strategy and operations, and improving the quality of AI outputs.
“Data is the foundation of large language models and therefore data professionals have the power to make or break AI applications,” said Roger Murff, VP of Technology Partners at Databricks. “The role of data teams – and the groundwork they set – is foundational to building AI models. That’s why it’s important that we solve the challenges posed to data teams so they can focus on creating reliable, transparent, and high-quality data.”
Key takeaways from the “The Data Practitioner for the AI Era” report include:
- Data jobs are quickly evolving as practitioners transition from traditional, siloed roles to more integrated, strategic positions within an organization. Data teams are integrating data intelligence platforms that enhance data visibility and transparency, bridging the gap between technical and business units.
- Data is the cornerstone of AI strategy and organizations are increasingly investing in it to remain competitive. Data practitioners are central to this effort, responsible for ensuring data quality, governance, and the effective deployment of AI technologies.
- The concept of “Data as a Product” is emerging as it requires practitioners to adopt a product management mindset, prioritizing tasks that have significant business impact. The result is better data practices and teams deeply embedded into business strategy and operations.
- Data challenges remain before organizations can realize their AI aspirations, including data silos, processing speed, data sufficiency, and monitoring lineage. Companies need to have a unified way to query and understand all their data across these silos.
- Retrieval-augmented generation (RAG) is a key technique to improve data quality for AI outputs, as organizations seek to mitigate issues like bias and hallucinations. By grounding AI models with verifiable external knowledge sources, organizations can ensure the production of higher-quality, more specialized, and verifiable AI outputs.
“AI is a gamechanger, and data roles, responsibilities, and workflows are transforming as it takes shape and becomes embedded in how teams work,” said Drew Banin, Cofounder of dbt Labs. “Analytics engineers for example, a new breed of data practitioners, are bridging the gap between data and business needs in a way that we haven’t seen before. They play a vital role in translating business requirements into effective data transformations, making data more accessible, actionable, and essential to business strategy and operations.”
To learn more, download the “The Data Practitioner for the AI Era” report.
About dbt Labs
Since 2016, dbt Labs has been on a mission to help analysts create and disseminate organizational knowledge. dbt Labs pioneered the practice of analytics engineering, built the primary tool in the analytics engineering toolbox, and has been fortunate enough to see a fantastic community coalesce to help push the boundaries of the analytics engineering workflow. Today there are 40,000 companies using dbt every week.
Source: dbt Labs