NIST Drafts Profile on Generative Artificial Intelligence Risks
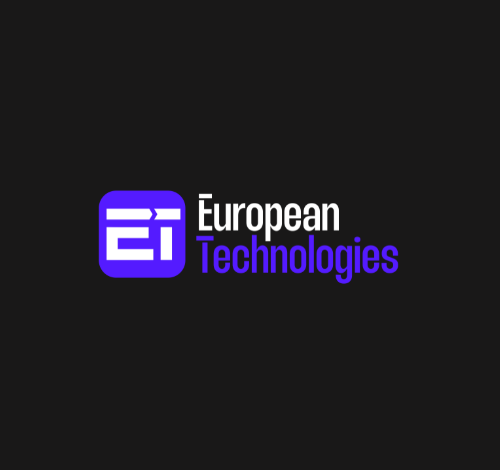
A year ago, we highlighted the National Institute of Standards and Technology’s (“NIST”) release of a framework designed to address AI risks (the “AI RMF”). We noted how it is abstract, like its central subject, and is expected to evolve and change substantially over time, and how NIST frameworks have a relatively short but significant history that shapes industry standards.
As support for the AI RMF, last month NIST released in draft form the Generative Artificial Intelligence Profile (the “Profile”).The Profile identifies twelve risks posed by Generative AI (“GAI”) including several that are novel or expected to be exacerbated by GAI. Some of the risks are exotic and new, such as confabulation, toxicity, and homogenization.
The Profile also identifies risks that are familiar, such as those for data privacy and cybersecurity. For the latter, the Profile details two types of cybersecurity risks: (1) those with the potential to discover or enable the lowering of barriers for offensive capabilities, and (2) those that can expand the overall attack surface by exploiting vulnerabilities as novel attacks.
For offensive capabilities and novel attack risks, the Profile includes these examples:
- Large language models (a subset of GAI) that discover vulnerabilities in data and write code to exploit them.
- GAI-powered co-pilots that proactively inform threat actors on how to evade detection.
- Prompt-injections that steal data and run code remotely on a machine.
- Compromised datasets that have been ‘poisoned’ to undermine the integrity of outputs.
In the past, the Federal Trade Commission (“FTC”) has referred to NIST when investigating companies’ data breaches. In settlement agreements, the FTC has required organizations to implement security measures through the NIST Cybersecurity Framework. It is reasonable to assume then, that NIST guidance on GAI will also be recommended or eventually required.
But it’s not all bad news – despite the risks when in the wrong hands, GAI will also improve cybersecurity defenses. As recently noted by Microsoft’s recent report on the GDPR & GAI, GAI can already: (1) support cybersecurity teams and protect organizations from threats, (2) train models to review applications and code for weaknesses, and (3) review and deploy new code more quickly by automating vulnerability detection.
Before ‘using AI to fight AI’ becomes legally required, just as multi-factor authentication, encryption, and training have become legally required for cybersecurity, the Profile should be considered to mitigate GAI risks. From pages 11-52, the Profile examines four hundred ways to use the Profile for GAI risks. Grouping them together, some of the recommendations include:
- Refine existing incident response plans and risk assessments if acquiring, embedding, incorporating, or using open-source or proprietary GAI systems.
- Implement regular adversary testing of the GAI, along with regular tabletop exercises with stakeholders and the incident response team to better inform improvements.
- Carefully review and revise contracts and service level agreements to identify who is liable for a breach and responsible for handling an incident in case one is identified.
- Document everything throughout the GAI lifecycle, including changes to any third parties’ GAI systems, and where audited data is stored.
“Cybersecurity is the mother of all problems. If you don’t solve it, all the other technology stuff just doesn’t happen” said Charlie Bell, Microsoft’s Chief of Security, in 2022. To that end, the AM RMF and now the Profile provide useful and early guidance on how to manage GAI Risks. The Profile is open for public comment until June 2, 2024.