NVIDIA’s JeDi: Joint-Image Diffusion models for fine-tuning generative AI
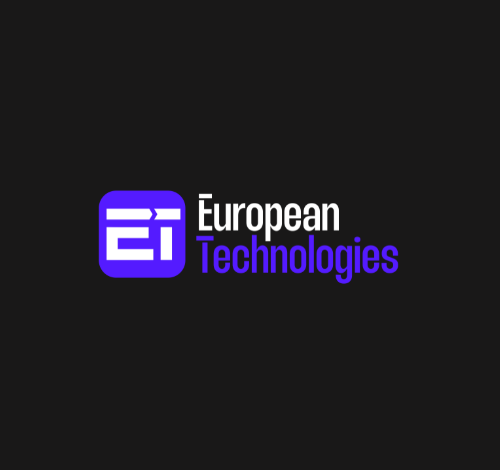

At the Computer Vision and Pattern Recognition (CVPR) Conference, NVIDIA has unveiled 57 papers and continues to break ground in the rapidly advancing field of visual generative AI (GenAI). One such paper, JeDi, proposes a new technique that allows users to easily personalize the output of a diffusion model within a couple of seconds using reference images, significantly outperforming existing methods.
The state-of-the-art in text-to-image generation has advanced significantly in the last two years, propelled by large-scale diffusion models and paired image-text datasets. One of the key problems with generative AI is using additional input to direct image generation (multi-modal input) and the related problem of maintaining consistency as you adjust the output. Oftentimes, one goes to refine a text-to-image generative AI, and the result is a completely different interpretation or inference.
NVIDIA’s research at CVPR includes the JeDi text-to-image model that can be easily customized to depict a specific object or character. To achieve the personalization capability, existing generative AI methods often rely on fine-tuning a text-to-image foundation model on a user’s custom dataset, which can be non-trivial for general users, plus resource-intensive and time-consuming. Despite attempts to develop fine-tuning the generation quality can be much worse.


The JeDi, approach is an effective technique fixing this. The researchers key idea is to learn the joint distribution of multiple related text-image pairs that share a common subject. To facilitate learning, they have a scalable synthetic dataset generation technique. Once trained, their model enables fast and easy personalization at test time by simply using reference images as input during the sampling process. Their approach does not require any expensive optimization process or additional modules and can faithfully preserve the identity or look represented by any number of reference images.

Despite generative AI diffusion models providing a great capability in generating high-quality images well aligned to the user’s text prompts, existing models cannot generate new images depicting specific custom objects or styles that are only available as few reference images.
The key challenge of personalized image generation is to produce distinct variations of a custom subject while preserving its visual appearance.

A limitation of JeDi is that it needs to process all the reference images at inference (run) time. This leads to an efficiency drop when the number of reference images increases. JeDi is thus more suitable for subject image generation given a few reference images, and its less efficient in adapting to a new large database of reference images.