Only 10% of Organizations are Doing Full Observability. Can Generative AI Move the Needle?
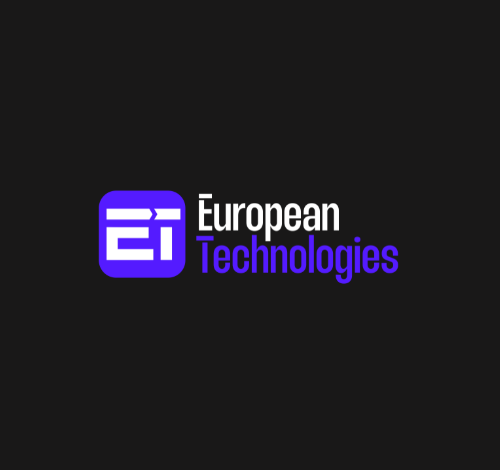
The Growing Challenge of Modern Observability
A decade ago, observability was comparatively straightforward. An engineer might manage a fixed number of servers equipped with clearly defined hardware limits, monitoring their performance through a handful of graphs of logs, metrics and traces. Today, the landscape is dramatically different. Modern environments often consist of Kubernetes clusters operating over ephemeral Docker containers, with components scaling dynamically. What was once a manageable number of graphs has ballooned to hundreds of dashboards thousands of graphs. This explosion of data creates a wall of noise akin to “sticking your head in a jet engine,” overwhelming even the most skilled professionals. The sheer volume and complexity of the data make traditional observability practices worthless.
How Generative AI Can Revolutionize Observability
Generative AI, powered by large language models (LLMs), introduces a transformative solution to these challenges. Instead of combing through hundreds of graphs, an engineer can now interact with a Generative AI assistant using a chat conversation to pinpoint issues efficiently. For instance, rather than identifying and correlating anomalies across multiple data points, an engineer could simply ask the AI, “Highlight the server experiencing issues,” and receive a focused response. This streamlines the troubleshooting process and significantly reduces the cognitive load on engineers.
I am old enough to remember what searching the internet was like before Google. You had to go on Yahoo and select a tab to pick a category like sports, economy, health and so forth. And then Google came along and said, “Here’s one line: search for whatever you want!” It was a total shift how people interacted with data, and it greatly increased our search efficiency. Just as Google simplified information retrieval with a single search bar, Generative AI simplifies observability by enabling natural language queries and interactions, enhancing efficiency and effectiveness.
Here’s a personal example: Recently I traveled with my 16-year-old daughter to Barcelona. Instead of reading old travel blogs and investigating each recommendation to see if the establishments are still open and something my daughter might like, I can now use Generative AI instead. I can ask ChatGPT: “I’m traveling with a 16-year-old to Barcelona between this date and this date; give me all the top attractions for her age, the time they open, how much tickets are…” Increased efficiency!
Or, let’s say I want to find a restaurant for a nice dinner with friends. I could search Google for “restaurants near me,” and visit web page after web page, ultimately calling phone number after phone number trying to determine availability. It’s annoying. It’s time-consuming. However, with a Generative AI assistant, I can ask for a list of the top five restaurant recommendations, and then engage in a conversation: “Okay, I don’t like the first one, but the second one is good — the Spanish one in Barcelona. Okay, I want to make a reservation at this restaurant for five people at 8 p.m. tonight.” It’s a different experience. With Generative AI, you’re having a conversation with the data.
Practical Applications of Generative AI in Observability
The potential applications of Generative AI in observability are vast. Engineers could start their week by querying their AI assistant about the weekend’s system performance, receiving a concise report that highlights only the most pertinent information. This assistant could provide real-time updates on system latency or deliver insights into user engagement for a gaming company, segmented by geography and time.
Imagine being able to enjoy your weekend and arrive at work with a calm and optimistic outlook on Monday morning, and essentially saying to your AI assistant: “Good morning! How did things go this weekend?” or “What’s my latency doing right now, as opposed to before the version release?” or “Can you tell me if there have been any changes in my audience, region by region, for the past 24 hours?”
These interactions exemplify how Generative AI can facilitate a more conversational and intuitive approach to managing development infrastructure. It’s about shifting from sifting through data to engaging in meaningful dialogue with data, where follow-up questions and deeper insights are just a query away.
A Glimpse Into the Future of Observability
The role of the observability engineer is poised for a significant transformation. With Generative AI, the days of manual graph analysis and data correlation are ending. This technology promises to reduce alert fatigue, reduce unnecessary complexity and enable engineers to focus on strategic tasks that add value to the business.
The forward march of MTTR growth signals not just a challenge but an opportunity — an opportunity for Generative AI to streamline processes and enhance the observability landscape. As systems continue to grow in complexity, the clarity provided by AI will become an indispensable tool in the engineer’s toolkit.
Bring on the New Era
Only 10% of organizations currently achieve full observability, but with the advent and rapid adoption of Generative AI, this number will increase dramatically. By automating the tedious aspects of data analysis and enhancing the interaction with development infrastructure, Generative AI is not just improving observability — it’s redefining it. As we stand on the brink of this new era, the potential for Generative AI to revolutionize how we manage and interact with our technological systems is immense and inspiring. This is not just an evolution; it is a revolution in observability that will usher in a new age of efficiency and insight.