Reimagining Search: How Google’s Generative AI is Transforming User Experience | by Oluwafemidiakhoa | May, 2024
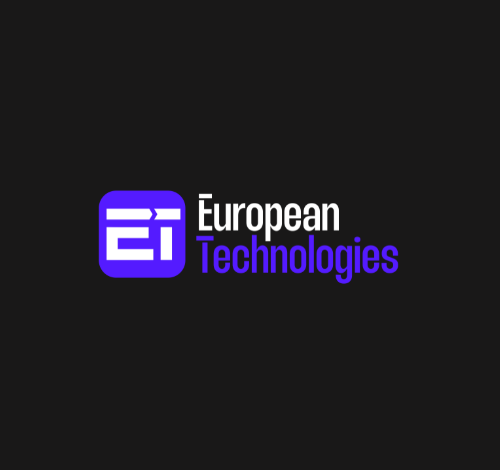
In the digital age, the demand for quick and accurate information is at an all-time high. Google, as the leading search engine, constantly seeks to enhance its user experience by integrating cutting-edge technologies. The latest breakthrough in this endeavor is the introduction of generative AI into Google Search. This innovation is powered by the Gemini model, a state-of-the-art AI that revolutionizes the way users interact with search results. By providing AI Overviews — quick summaries accompanied by detailed information links — Google aims to transform the search experience, making it more efficient, relevant, and user-friendly.
Generative AI marks a significant shift from traditional keyword-based search approaches to more intuitive and context-aware methods. It can understand and generate human-like text, offering users a comprehensive overview of their search queries instantly. This article explores the profound impact of generative AI on Google’s search capabilities. We will delve into the evolution of Google Search, the workings of generative AI and the Gemini model, the benefits of AI Overviews, and the potential challenges and ethical considerations of this technology. Through this exploration, we aim to understand how Google’s generative AI is set to redefine the future of information retrieval and user experience.
Since its inception in 1998, Google Search has undergone a remarkable evolution, transforming from a basic search engine into a sophisticated tool that leverages advanced algorithms and machine learning techniques. Understanding this evolution provides context for the significance of integrating generative AI into Google Search.
The Early Days: Keyword-Based Search
In its early days, Google Search relied heavily on keyword-based search algorithms. The foundational algorithm, PageRank, evaluated the relevance of web pages based on the number and quality of links pointing to them. This approach allowed Google to deliver more accurate search results compared to its competitors at the time. Users would input keywords, and Google would return a list of web pages ranked by relevance.
Advancements in Search Algorithms
As the internet grew, so did the complexity of search queries. Users began to seek more specific information, prompting Google to enhance its search algorithms. The introduction of the Knowledge Graph in 2012 marked a significant advancement. The Knowledge Graph enabled Google to understand the relationships between different pieces of information, providing users with more contextually relevant results.
The Hummingbird update in 2013 further refined search capabilities by focusing on the meaning behind search queries rather than just matching keywords. This update allowed Google to better understand conversational queries and deliver results that were more aligned with user intent.
Machine Learning and Artificial Intelligence
The incorporation of machine learning and AI into Google Search brought about a new era of search technology. Algorithms like RankBrain, introduced in 2015, leveraged machine learning to handle complex and ambiguous queries more effectively. RankBrain helped Google to better understand the context of queries and improve the relevance of search results.
Another significant milestone was the introduction of BERT (Bidirectional Encoder Representations from Transformers) in 2019. BERT enabled Google to understand the nuances of language by analyzing the context of words in a sentence. This deep learning model allowed Google to provide more accurate answers to complex queries and improve the overall search experience.
The Rise of Generative AI
The integration of generative AI into Google Search represents the latest chapter in this evolution. Generative AI models, such as the Gemini model, have the capability to create new content based on existing data. Unlike traditional AI, which primarily focuses on classification and prediction, generative AI can generate novel outputs, making it an ideal tool for enhancing search capabilities.
The Gemini Model: A New Era
The Gemini model, developed by Google, leverages deep learning techniques to analyze vast amounts of data and generate meaningful content. This model is capable of producing high-quality text summaries, answering complex questions, and even generating creative content. By incorporating the Gemini model into Google Search, Google aims to provide users with more informative and engaging search results.
The Gemini model is designed to understand and generate human-like text, making it an ideal tool for enhancing search capabilities. It leverages transformer architectures, similar to those used in BERT, to process and generate text. This allows the model to analyze context and generate coherent summaries that are both accurate and relevant.
The Impact of Generative AI on Search
The integration of generative AI in Google Search has several key impacts. First, it enhances the relevance and accuracy of search results by providing users with quick summaries of search topics. These AI Overviews offer a concise overview of the information, saving users time and effort. Additionally, the detailed information links included in the AI Overviews provide users with access to more in-depth content, ensuring they have comprehensive information at their fingertips.
Second, generative AI improves the accessibility of information. By generating easy-to-understand summaries, it makes complex topics more approachable for users with varying levels of knowledge. This democratizes access to information, allowing a broader audience to benefit from Google’s search capabilities.
Finally, generative AI enhances the overall user experience by making search more interactive and engaging. The ability to generate creative and contextually relevant content adds a new dimension to the search experience, making it more dynamic and user-friendly.
The evolution of Google Search from a simple keyword-based tool to a sophisticated AI-driven platform highlights the company’s commitment to enhancing the user experience. The integration of generative AI, powered by the Gemini model, represents a significant leap forward in this journey. By providing quick summaries and detailed information links, generative AI is set to transform the way users interact with search results, making information retrieval more efficient, accessible, and engaging. As we delve deeper into the workings of generative AI and its impact on search technology, it becomes clear that the future of Google Search is both promising and exciting.
What is Generative AI?
Generative AI is a branch of artificial intelligence focused on creating new content based on existing data. Unlike traditional AI, which primarily focuses on tasks such as classification, prediction, and optimization, generative AI can generate novel outputs. This includes producing text, images, music, and even entire virtual environments. The core idea behind generative AI is to learn patterns and structures from the input data and use this knowledge to create new, similar content.
How Does Generative AI Work?
Generative AI models are typically built using deep learning techniques, particularly neural networks. One of the most common architectures used in generative AI is the transformer model. Transformers are designed to handle sequential data and are particularly well-suited for tasks involving natural language processing (NLP).
Transformers consist of an encoder and a decoder. The encoder processes the input data and converts it into a series of hidden states, which capture the contextual information of the input. The decoder then takes these hidden states and generates the output data. This architecture allows transformers to generate coherent and contextually relevant content.
The Gemini model, developed by Google, is an advanced generative AI model based on the transformer architecture. It leverages deep learning techniques to analyze vast amounts of data and generate meaningful content. The Gemini model is designed to understand and generate human-like text, making it an ideal tool for enhancing search capabilities.
The Gemini Model
The Gemini model represents a significant advancement in generative AI technology. It builds on the success of previous models, such as GPT-3 and BERT, but introduces several innovations that make it particularly powerful for search applications.
One of the key features of the Gemini model is its ability to generate high-quality text summaries. By analyzing large datasets and identifying key patterns, the model can produce concise and coherent summaries of complex topics. This capability is particularly useful in the context of Google Search, where users often seek quick and accurate information.
Another important aspect of the Gemini model is its ability to answer complex questions. By leveraging its deep learning capabilities, the model can understand the context of a query and generate relevant and informative answers. This makes it an invaluable tool for enhancing the search experience, as it allows users to obtain precise information quickly and easily.
The Gemini model is also capable of generating creative content. This includes writing articles, creating stories, and even generating poetry. This capability adds a new dimension to the search experience, making it more engaging and interactive.
Training the Gemini Model
Training the Gemini model involves feeding it large amounts of data and allowing it to learn patterns and structures from this data. The training process is computationally intensive and requires powerful hardware, such as GPUs and TPUs. During training, the model is exposed to a diverse range of texts, which helps it develop a deep understanding of language and context.
One of the challenges in training generative AI models is ensuring that they produce high-quality and reliable outputs. To address this, the training process involves several steps, including data preprocessing, model optimization, and validation. Data preprocessing involves cleaning and organizing the input data to ensure it is suitable for training. Model optimization involves fine-tuning the model’s parameters to improve its performance. Validation involves testing the model on unseen data to ensure it generalizes well and produces accurate outputs.
Applications of the Gemini Model in Google Search
The integration of the Gemini model into Google Search has several practical applications. One of the most notable applications is the AI Overview feature. This feature provides users with quick summaries of search topics, allowing them to grasp essential information at a glance. By generating AI Overviews, the Gemini model enhances the relevance and accuracy of search results, making it easier for users to find what they need.
Another application of the Gemini model is in answering complex questions. The model’s ability to understand context and generate informative answers makes it an invaluable tool for improving the search experience. Users can ask detailed questions and receive precise answers, reducing the time and effort required to find information.
The Gemini model also has the potential to enhance other aspects of Google Search, such as content creation and personalization. By generating creative and contextually relevant content, the model can make the search experience more engaging and interactive. Additionally, by understanding user preferences and generating personalized content, the model can provide a more tailored search experience.
Generative AI represents a significant advancement in artificial intelligence technology, with the potential to transform various industries, including search. The Gemini model, developed by Google, leverages deep learning techniques to generate high-quality text summaries, answer complex questions, and create engaging content. By integrating the Gemini model into Google Search, Google aims to enhance the user experience, making information retrieval more efficient, relevant, and engaging.
As we continue to explore the capabilities and applications of generative AI, it becomes clear that this technology has the potential to redefine the future of search and information retrieval. The Gemini model represents a significant step forward in this journey, offering a glimpse into the exciting possibilities that lie ahead.
Introduction to AI Overviews
One of the most transformative features introduced by the Gemini model in Google Search is the AI Overview. AI Overviews are designed to provide users with concise, coherent summaries of their search topics, along with detailed information links. This dual approach enhances the search experience by offering both a quick grasp of the subject and the option to delve deeper into more comprehensive content.
How AI Overviews Work
AI Overviews are generated using the advanced capabilities of the Gemini model. When a user inputs a search query, the model analyzes vast amounts of relevant data, extracts the most pertinent information, and compiles it into a summary. This summary captures the essence of the topic, making it easy for users to understand the core points without having to navigate through multiple sources.
In addition to the summary, AI Overviews include detailed information links. These links direct users to sources that provide more in-depth information on the topic. By clicking on these links, users can explore the subject further, accessing comprehensive articles, studies, and other resources.
Benefits of AI Overviews
Time Efficiency
One of the primary benefits of AI Overviews is the significant time savings they offer. In the traditional search model, users often need to sift through numerous search results, scan through pages of content, and extract the relevant information themselves. AI Overviews streamline this process by presenting the most crucial information upfront. This allows users to quickly grasp the main points of their query and decide if they need to explore further.
Improved Relevance
AI Overviews enhance the relevance of search results. By analyzing and summarizing data from various sources, the Gemini model ensures that the information presented is comprehensive and contextually appropriate. This reduces the likelihood of encountering irrelevant or redundant content, thereby improving the overall search experience.
Enhanced Accessibility
Another significant advantage of AI Overviews is their ability to make information more accessible. Summarizing complex topics into easily digestible content helps users with varying levels of knowledge understand the subject matter. This is particularly beneficial for users who are unfamiliar with the topic or are looking for a quick answer without needing to delve into detailed articles.
Comprehensive Understanding
While AI Overviews provide concise summaries, they also offer detailed information links that cater to users who seek a deeper understanding of the topic. This dual approach ensures that users have access to both high-level overviews and in-depth content, allowing them to tailor their search experience according to their needs.
Examples of AI Overviews in Action
To illustrate the impact of AI Overviews, consider a user searching for information on climate change. Upon entering the query, the Gemini model generates an AI Overview that summarizes the key points: the causes of climate change, its effects on the environment, and potential mitigation strategies. Alongside the summary, the AI Overview provides links to detailed articles, scientific studies, and authoritative resources that offer a deeper exploration of the topic.
Another example is a user searching for information on a medical condition, such as diabetes. The AI Overview might include a brief summary of the condition, its symptoms, treatment options, and lifestyle recommendations. Detailed information links could direct the user to reputable medical websites, research papers, and patient support forums.
Challenges and Limitations of AI Overviews
While AI Overviews offer numerous benefits, they also present certain challenges and limitations. One of the primary challenges is ensuring the accuracy and reliability of the generated summaries. As the model relies on existing data to create summaries, it is crucial to ensure that the sources of this data are credible and up-to-date.
Another challenge is addressing the potential for bias in the AI-generated content. The training data for the Gemini model may contain biases, which can be reflected in the summaries. Ensuring fairness and neutrality in the AI Overviews is essential to provide unbiased and accurate information to users.
Moreover, there is the risk of information overload. While detailed information links are beneficial, presenting too many options can overwhelm users. Striking a balance between providing comprehensive information and maintaining user-friendliness is crucial.
Future Prospects of AI Overviews
The future of AI Overviews looks promising, with continuous advancements in generative AI technology. As the Gemini model evolves, we can expect improvements in the accuracy, relevance, and comprehensiveness of the summaries. Additionally, advancements in natural language processing and machine learning will enhance the model’s ability to understand and generate human-like text, further improving the user experience.
One potential area of development is the personalization of AI Overviews. By leveraging user data and preferences, the Gemini model could generate customized summaries that cater to individual needs and interests. This would further enhance the relevance and utility of the search results.
Another exciting prospect is the integration of AI Overviews with other Google services and platforms. For example, AI Overviews could be incorporated into Google Assistant, providing users with concise information on voice queries. They could also be integrated into educational platforms, offering students quick summaries and detailed resources for their research.
AI Overviews, powered by the Gemini model, represent a significant advancement in Google Search technology. By providing quick summaries and detailed information links, they enhance the efficiency, relevance, and accessibility of search results. While there are challenges to address, the potential benefits of AI Overviews are immense. As generative AI technology continues to evolve, we can look forward to even more sophisticated and personalized search experiences. AI Overviews are set to play a central role in this transformation, making information retrieval more efficient, engaging, and user-friendly.
Introduction to User Experience Transformation
The integration of generative AI, particularly through the Gemini model and AI Overviews, has the potential to revolutionize how users interact with search engines. This transformation goes beyond mere improvements in search results; it fundamentally changes the user journey, making information retrieval more intuitive, personalized, and efficient. In this chapter, we explore the various ways in which generative AI is transforming the user experience in Google Search.
Efficiency and Speed
Quick Access to Information
One of the most immediate and noticeable impacts of AI Overviews is the significant reduction in the time users spend searching for information. Traditional search methods often involve navigating through multiple web pages and extracting relevant details manually. AI Overviews, however, provide quick summaries of search topics, allowing users to access essential information instantly. This efficiency is particularly valuable for users seeking quick answers to straightforward questions or needing to gather information rapidly in time-sensitive situations.
Streamlined Decision-Making
By presenting concise and relevant summaries, AI Overviews aid users in making informed decisions quickly. Whether it’s finding the best product to purchase, understanding the basics of a complex topic, or getting the latest updates on a news story, AI Overviews help users cut through the noise and get straight to the significant part. This streamlined approach enhances user satisfaction and reduces the cognitive load associated with traditional search methods.
Enhanced Relevance and Contextual Understanding
Context-Aware Responses
Generative AI’s ability to understand the context of a query significantly enhances the relevance of search results. The Gemini model’s deep learning capabilities allow it to process complex and nuanced queries, providing answers that are contextually appropriate. This is particularly beneficial for queries that involve multiple layers of meaning or require a deep understanding of the subject matter. Users receive results that are not only accurate but also tailored to their specific needs and contexts.
Comprehensive Overviews
AI Overviews bring together information from various sources, providing a comprehensive understanding of a topic. This holistic approach ensures that users are presented with a well-rounded perspective, incorporating different viewpoints and data points. For instance, a search query about climate change might include scientific data, policy implications, and practical mitigation strategies, all summarized in a single overview. This comprehensive approach enriches the user experience by offering a broader and more informed view of the topic.
Personalization and Customization
Tailored Search Experiences
Generative AI opens new possibilities for personalized search experiences. By leveraging user data and preferences, AI Overviews can be customized to reflect individual interests and needs. For example, a user frequently searching for medical information might receive AI Overviews that prioritize reputable medical sources and detailed health information. Personalization ensures that users receive the most relevant and useful content, enhancing the overall search experience.
Adaptive Learning
As users interact with Google Search, the system can learn from their behavior and preferences, continually refining and improving the relevance of AI Overviews. This adaptive learning capability allows the search engine to evolve alongside the user, providing increasingly accurate and personalized results over time. The more a user engages with the search engine, the better it becomes at delivering content that aligns with their specific needs and interests.
Interactive and Engaging Content
Dynamic Interactions
The generative capabilities of the Gemini model enable the creation of interactive and engaging content. AI Overviews can include multimedia elements such as images, videos, and interactive charts, making the search experience more dynamic and visually appealing. This interactive approach not only enhances user engagement but also aids in better comprehension and retention of information.
Creative Content Generation
Beyond factual summaries, generative AI can produce creative content that enhances the user experience. This includes generating personalized recommendations, writing summaries of long articles, and even creating original content based on user queries. For example, a user searching for writing inspiration might receive AI-generated story prompts or creative writing tips. This creative potential adds a new dimension to the search experience, making it more enjoyable and inspirational.
Accessibility and Inclusivity
Simplifying Complex Information
AI Overviews make complex information more accessible by breaking it down into simple, digestible summaries. This is particularly beneficial for users with limited expertise in a subject or those who may find certain topics difficult to understand. By presenting information in a clear and concise manner, AI Overviews democratize access to knowledge, making it easier for everyone to benefit from Google Search.
Supporting Diverse User Needs
Generative AI can cater to diverse user needs, providing customized content for different demographics. This includes offering multilingual support, accessibility features for users with disabilities, and culturally relevant information. By considering the diverse backgrounds and needs of its users, Google Search can create a more inclusive and supportive search environment.
The integration of generative AI into Google Search, exemplified by the Gemini model and AI Overviews, represents a transformative leap in enhancing the user experience. By providing quick, relevant, and personalized information, generative AI not only streamlines the search process but also makes it more engaging and accessible. The ability to generate interactive and creative content further enriches the user journey, transforming how people interact with information.
As generative AI continues to evolve, the potential for further enhancements in user experience is immense. Personalization, adaptive learning, and inclusivity will remain key focus areas, ensuring that Google Search meets the diverse needs of its global user base. The future of search is not just about finding information; it’s about creating an intuitive, engaging, and personalized experience that empowers users to access knowledge effortlessly and effectively.
Introduction to Challenges and Ethical Considerations
While the integration of generative AI into Google Search offers numerous benefits, it also presents several challenges and ethical considerations that must be addressed. These include ensuring the accuracy and reliability of AI-generated content, mitigating biases, protecting user privacy, and maintaining transparency. Addressing these issues is crucial to harnessing the full potential of generative AI while safeguarding user trust and upholding ethical standards.
Ensuring Accuracy and Reliability
Risk of Misinformation
One of the primary challenges associated with generative AI is the risk of misinformation. AI models like Gemini generate content based on patterns learned from vast datasets, which may contain inaccuracies or outdated information. This can lead to the propagation of incorrect or misleading summaries in AI Overviews, potentially causing harm to users who rely on these overviews for critical information.
To mitigate this risk, it is essential to continuously update the training data and ensure it comes from reliable and reputable sources. Implementing rigorous validation and verification processes can also help maintain the accuracy of AI-generated content. Furthermore, integrating mechanisms for cross-referencing information against multiple sources can enhance the reliability of the summaries provided.
Quality Control Measures
Implementing robust quality control measures is vital to maintaining the integrity of AI Overviews. This includes regular audits of the generated content, leveraging human oversight to review and correct inaccuracies, and employing feedback loops where users can report errors or discrepancies. By incorporating these measures, Google can ensure that the AI-generated summaries remain accurate and trustworthy.
Addressing Bias and Fairness
Bias in Training Data
AI models are trained on large datasets that may contain inherent biases, reflecting societal prejudices and inequalities. If not addressed, these biases can be perpetuated in the AI-generated content, leading to unfair or discriminatory outcomes. For instance, biased data can result in skewed representations of certain groups or topics, affecting the objectivity and fairness of search results.
To tackle this issue, it is crucial to employ diverse and representative training datasets that minimize biases. Additionally, implementing bias detection and mitigation techniques during the model training phase can help identify and reduce biases. Continuous monitoring and refinement of the model are also necessary to ensure that it generates fair and impartial content.
Ethical AI Development
Developing ethical AI requires a commitment to fairness, accountability, and transparency. This involves establishing clear guidelines and ethical standards for AI development and deployment. Engaging with diverse stakeholders, including ethicists, legal experts, and affected communities, can provide valuable insights and help shape ethical AI practices. By fostering an ethical AI ecosystem, Google can ensure that its generative AI technologies align with societal values and promote fairness.
Protecting User Privacy
Data Privacy Concerns
The use of generative AI in search engines raises important questions about data privacy. AI models rely on vast amounts of data to generate content, and there is a need to ensure that this data is collected, stored, and used in a manner that respects user privacy. Unauthorized access or misuse of user data can lead to significant privacy breaches and erode user trust.
Implementing robust data protection measures, such as encryption, anonymization, and secure data storage, is essential to safeguard user privacy. Additionally, adhering to stringent data governance policies and complying with legal and regulatory frameworks can further enhance data privacy protections.
Transparency and Consent
Ensuring transparency and obtaining user consent are fundamental to ethical AI practices. Users should be informed about how their data is being used and have the option to opt-out if they choose. Providing clear and concise information about data collection, usage, and storage practices can empower users to make informed decisions about their privacy. Additionally, offering granular privacy controls can give users greater control over their data and how it is utilized.
Maintaining Transparency and Accountability
Explainability of AI Decisions
One of the key ethical considerations in AI development is ensuring the explainability of AI decisions. Users should be able to understand how AI models generate content and the rationale behind specific search results. This is particularly important in cases where AI Overviews influence critical decisions, such as medical diagnoses or financial recommendations.
Implementing explainability techniques, such as providing insights into the model’s decision-making process and highlighting the sources of information, can enhance transparency. This can help users trust the AI-generated content and make informed decisions based on the provided summaries.
Accountability Mechanisms
Establishing accountability mechanisms is crucial to ensure that AI systems operate responsibly. This includes defining clear roles and responsibilities for AI developers, operators, and stakeholders. Implementing robust audit trails and monitoring systems can help track the performance and impact of AI models. In cases where AI systems fail or cause harm, having accountability mechanisms in place ensures that responsible parties can be identified, and corrective actions can be taken.
The integration of generative AI into Google Search, while transformative, brings forth several challenges and ethical considerations that must be addressed to ensure a positive user experience. Ensuring the accuracy and reliability of AI-generated content, addressing biases, protecting user privacy, and maintaining transparency are critical to the responsible deployment of generative AI.
By implementing rigorous quality control measures, employing diverse and representative training datasets, and adhering to ethical AI practices, Google can mitigate these challenges and uphold user trust. Engaging with stakeholders and fostering an ethical AI ecosystem are also essential to aligning AI technologies with societal values and promoting fairness.
As generative AI continues to evolve, ongoing efforts to address these challenges and uphold ethical standards will be crucial to realizing its full potential. By prioritizing accuracy, fairness, privacy, and transparency, Google can ensure that its generative AI technologies enhance the user experience while respecting ethical principles and promoting societal well-being.
The advent of generative AI in Google Search, exemplified by the Gemini model and AI Overviews, marks a significant milestone in the evolution of search technology. By providing quick summaries and detailed information links, generative AI enhances the efficiency, relevance, and accessibility of search results, fundamentally transforming the user experience.
Summarizing the Impact of Generative AI
Efficiency and Relevance
Generative AI significantly reduces the time users spend searching for information by presenting concise and relevant summaries. This efficiency is particularly valuable in today’s fast-paced digital world, where users demand quick and accurate answers. By understanding the context of queries, the Gemini model ensures that search results are not only accurate but also contextually appropriate, enhancing the relevance of the information provided.
Personalization and Engagement
The ability to personalize search experiences based on user preferences and behavior further enhances the user journey. Adaptive learning capabilities allow the search engine to evolve alongside the user, delivering increasingly accurate and personalized results over time. The generation of interactive and engaging content adds a dynamic dimension to the search experience, making it more enjoyable and informative.
Accessibility and Inclusivity
AI Overviews make complex information more accessible by breaking it down into simple, digestible summaries. This democratizes access to knowledge, allowing users with varying levels of expertise to benefit from Google Search. By supporting diverse user needs and offering multilingual and accessible features, generative AI promotes inclusivity and ensures that information is available to a broader audience.
Addressing Challenges and Ethical Considerations
Ensuring Accuracy and Mitigating Bias
Maintaining the accuracy and reliability of AI-generated content is critical to preserving user trust. Implementing rigorous quality control measures and continuously updating training data can help mitigate the risk of misinformation. Addressing biases in training data and ensuring fairness in AI-generated content are also essential to promoting ethical AI practices.
Protecting User Privacy
Robust data protection measures and transparent data usage policies are crucial to safeguarding user privacy. By providing clear information about data collection and usage, and offering granular privacy controls, Google can empower users to make informed decisions about their data.
Maintaining Transparency and Accountability
Ensuring the explainability of AI decisions and establishing accountability mechanisms are key to maintaining transparency and user trust. By providing insights into the model’s decision-making process and implementing robust monitoring systems, Google can ensure that its AI systems operate responsibly and ethically.
The Future of Search with Generative AI
The integration of generative AI into Google Search is just the beginning. As AI technologies continue to evolve, the potential for further enhancements in user experience is immense. Personalization, adaptive learning, and inclusivity will remain key focus areas, ensuring that Google Search meets the diverse needs of its global user base.
Future developments in generative AI could include more sophisticated personalization features, deeper integration with other Google services, and the ability to generate even more complex and creative content. By continuously innovating and addressing ethical considerations, Google can ensure that its search technologies remain at the forefront of the digital information landscape.
Conclusion
Generative AI, powered by the Gemini model, is set to redefine the future of search and information retrieval. By providing quick, relevant, and personalized information, it enhances the efficiency, accessibility, and engagement of search results. Addressing the challenges and ethical considerations associated with this technology is crucial to ensuring a positive and responsible user experience.
As we look to the future, the potential for generative AI to transform the way we search for and interact with information is both exciting and profound. By prioritizing ethical AI practices and continuously innovating, Google can harness the full potential of generative AI, creating a search experience that is not only efficient and relevant but also fair, transparent, and inclusive. The future of search is here, and it is powered by generative AI.