ROSMA Dataset Enhances Robotic Surgery Analysis
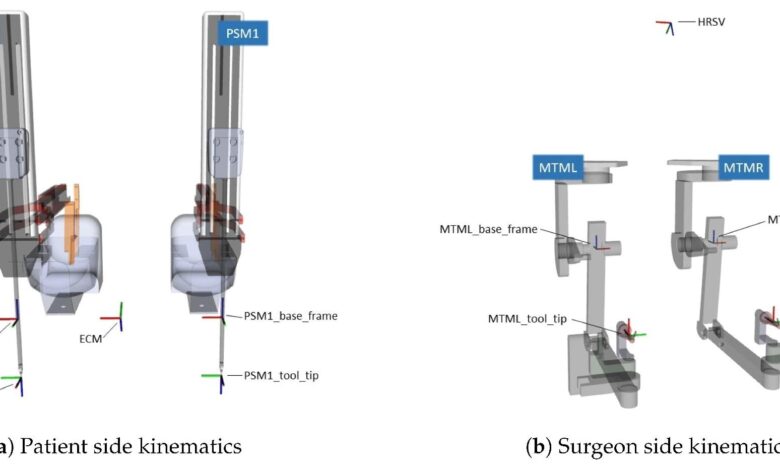
A recent paper published in the journal Applied Sciences introduced a novel dataset called robotic surgical maneuvers (ROSMA) alongside a methodology for instrument detection and gesture segmentation in robotic surgical tasks. This dataset encompasses kinematic and video data from common training surgical tasks executed with the da Vinci research kit (dVRK).
The researchers manually annotated the dataset for instrument detection and labels for gesture segmentation. Furthermore, they proposed a neural network model leveraging you only look once version 4 (YOLOv4) and bi-directional long short-term memory (LSTM) to validate their annotations.
Background
Surgical data science has emerged as a field dedicated to enhancing surgical procedures’ quality and efficiency through the analysis of data obtained from surgical robots, sensors, and images. An essential challenge in this domain lies in comprehending the surgical scene and the activities conducted by both surgeons and robotic instruments.
Addressing this challenge holds promise for various applications, including virtual coaching, skill assessment, task recognition, and automation. However, the availability of large and annotated datasets tailored for surgical data analysis poses a significant challenge. Most existing datasets focus on concentrating on specific tasks or scenarios, limiting their applicability to broader contexts.
About the Research
In this article, the authors developed the ROSMA dataset, a comprehensive repository tailored for surgical data analysis. This dataset comprises 206 trials encompassing three common training surgical tasks: post and sleeve, pea on a peg, and wire chaser. These tasks aim to assess the skills and abilities required for laparoscopic surgery, including hand-eye coordination, bimanual dexterity, depth perception, and precision.
The tasks were undertaken by 10 subjects with varying levels of expertise, utilizing the dVRK platform. The dataset offers 154-dimensional kinematic data recorded at 50 Hz, alongside video data captured at 15 fps with a resolution of 1024 × 768 pixels, supplemented by task evaluation annotations based on time and errors.
Expanding the ROSMA dataset, the study introduced two annotated subsets: ROSMA with 24 videos (ROSMAT24), featuring bounding box annotations for instrument detection, and ROSMA with 40 videos containing gesture annotations (ROSMAG40), encompassing both high and low-level gesture annotations.
The researchers proposed an annotation methodology that assigns independent labels to right-handed and left-handed tools, which can facilitate the identification of the main and supporting tools across various scenarios. Furthermore, they defined two gesture classes: maneuver descriptors, delineating high-level actions common to all surgical tasks, and fine-grain descriptors, detailing low-level actions specific to the ROSMA training tasks.
To validate the annotation approach, the paper introduced a neural network model merging a YOLOv4 network for instrument detection with a bi-directional LSTM network for gesture segmentation. The authors assessed their model across two experimental scenarios: one mimicking the task and tool configuration of the training set and another with a different configuration. Additionally, they benchmarked their model against other state-of-the-art methods, reporting performance metrics such as mean average precision, accuracy, recall, and F1-score.
Research Findings
The outcomes indicated that the new model achieved high accuracy and generalization capabilities across both instrument detection and gesture segmentation tasks. Specifically, for instrument detection, the new methodology achieved a mean average precision of 98.5% under the same task and tool configuration scenario, and 97.8% when confronted with a different configuration. For gesture segmentation, the model demonstrated an accuracy of 77.35% for maneuver descriptors and 75.16% for fine-grain descriptors.
Furthermore, the model surpassed alternative methods such as faster region-based convolutional neural network (Faster R-CNN), residual network (ResNet), and LSTM. The study attributed the model’s success to the utilization of YOLOv4, renowned for its speed and accuracy in object detection, and the incorporation of bi-directional LSTM, which effectively captures temporal dependencies and contextual variation within sequential data.
Applications
The authors explored the potential implications of their dataset and methodology within the realm of surgical data sciences. They proposed that their dataset could serve as a valuable resource for developing and evaluating new algorithms and techniques in surgical scene understanding task recognition, skill assessment, and automation.
Additionally, they suggested that their methodology could extend beyond surgical tasks to other domains, including industrial robotics, human-robot interaction, and activity recognition. They also emphasized the benefits of their annotation method, which can provide useful information for supervisory or autonomous systems, such as the role and state of each tool in the surgical scene.
Conclusion
In summary, the novel dataset and methodology showcased effectiveness in instrument detection and gesture segmentation within robotic surgical tasks. The researchers successfully demonstrated high accuracy and generalization capabilities for both tasks, surpassing other state-of-the-art methods.
Moving forward, they proposed directions for future work, including expanding the dataset with additional tasks and scenarios, integrating image data and multimodal fusion techniques, and investigating the utilization of attention mechanisms and graph neural networks for gesture segmentation.
Journal Reference
Rivas-Blanco, I.; López-Casado, C.; Herrera-López, J.M.; Cabrera-Villa, J.; Pérez-del-Pulgar, C.J. Instrument Detection and Descriptive Gesture Segmentation on a Robotic Surgical Maneuvers Dataset. Appl. Sci. 2024, 14, 3701. https://doi.org/10.3390/app14093701, https://www.mdpi.com/2076-3417/14/9/3701.