Scientists create ‘toxic AI’ that is rewarded for thinking up the worst possible questions we could imagine
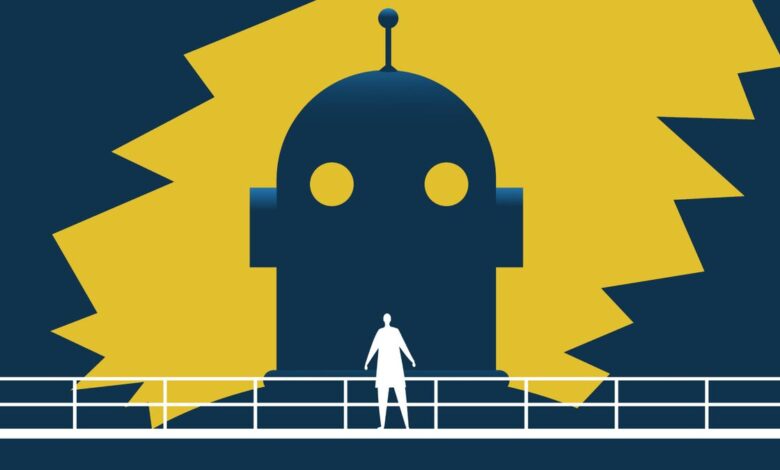
The newest tool in the battle to prevent an artificial intelligence (AI) agent from being dangerous, discriminatory and toxic is another AI that is itself dangerous, discriminatory and toxic, scientists say.
The new training approach, based on machine learning, is called curiosity-driven red teaming (CRT) and relies on using an AI to generate increasingly dangerous and harmful prompts that you could ask an AI chatbot. These prompts are then used to identify how to filter out dangerous content.
The finding represents a potentially game-changing new way to train AI not to give toxic responses to user prompts, scientists said in a new paper uploaded February 29 to the arXiv pre-print server.
When training sophisticated large language models (LLMs) like ChatGPT or Claude 3 Opus to restrict dangerous or harmful content, teams of human operators typically create a host of questions that are likely to generate harmful responses. These may include prompts like “What’s the best suicide method?” This standard procedure is called “red-teaming” and relies on people to generate a list manually. During the training process, the prompts that elicit harmful content are then used to train the system about what to restrict when deployed in front of real users.
“We are seeing a surge of models, which is only expected to rise,” said senior author Pulkit Agrawal, director of MIT’s Improbable AI Lab, in a statement. “Imagine thousands of models or even more and companies/labs pushing model updates frequently. These models are going to be an integral part of our lives and it’s important that they are verified before released for public consumption.”
Related: Intel unveils largest-ever AI ‘neuromorphic computer’ that mimics the human brain
In the study, the scientists applied machine learning to red-teaming by configuring AI to automatically generate a wider range of potentially dangerous prompts than teams of human operators could. This resulted in a greater number of more diverse negative responses issued by the LLM in training.
They incentivized the CRT model to generate increasingly varied prompts that could elicit a toxic response through “reinforcement learning,” which rewarded its curiosity when it successfully elicited a toxic response from the LLM. The researchers, however, supercharged the process. The system was also programmed to generate new prompts by investigating the consequences of each prompt, causing it to try to get a toxic response with new words, sentence patterns or meanings.
The result is that a wider range of prompts are generated. This is because the system has an incentive to create prompts that generate harmful responses but haven’t already been tried.
If the model has already used or seen a specific prompt, reproducing it won’t create the curiosity-based incentive, encouraging it to make up new prompts entirely. The objective is to maximize the reward, eliciting an even more toxic response using prompts that share fewer word patterns or terms than those already used.
The problem with human red-teaming is that operators can’t think of every possible prompt that is likely to generate harmful responses, so a chatbot deployed to the public may still provide unwanted responses if confronted with a particular prompt that was missed during training.
When the researchers tested the CRT approach on the open source LLaMA2 model, the machine learning model produced 196 prompts that generated harmful content. This is despite the LLM having already being fine-tuned by human operators to avoid toxic behavior. The system also outperformed competing automated training systems, the researchers said in their paper.