Securing The Use Of Artificial Intelligence And Machine Learning In Legal Services
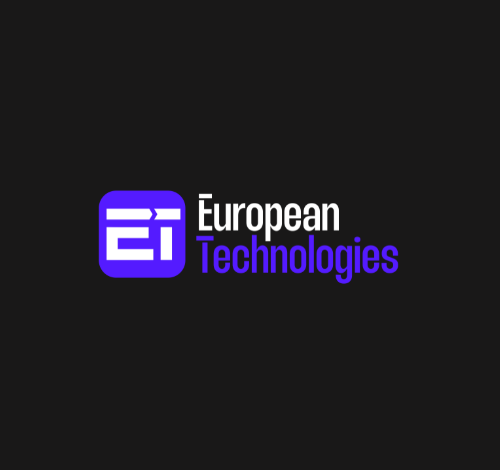
Ed. note: This article first appeared in an ILTA publication. For more, visit our ILTA on ATL channel here.
The emergence and rapid development of Artificial Intelligence (AI) and Machine Learning (ML) within legal services is creating extraordinary opportunities for legal professionals. Many law firms and legal entities eagerly embrace AI/ML technologies to assist with tasks like research, document analysis, and case prediction. While these advancements are revolutionizing branches of the industry and generating unparalleled excitement within various circles, other groups of legal professionals are reluctant to consider the potential benefits of incorporating AI/ML tools into their daily workflow. Mapping the multitude of causes driving this dichotomy between excitement and frigidity around AI/ML advancements among legal professionals exceeds the parameters of this project. However, we can identify and carefully consider one of the more subtle motivators generating reservations around normalizing AI/ML within legal services.
The fact that AI/ML tools exceed the limitations of human capabilities in several areas is quickly becoming common knowledge. Moreover, these technological advancements have reached a point where AI/ML directs machines to learn, adapt, and understand data in ways that mimic or surpass human intelligence. AI allows the computer to think, learn, and problem-solve like humans, while ML constructs algorithms to learn from the data. Although AI/ML has been around for decades, only now is the technology presenting responses typically indicative of self-aware beings; it wants to be acknowledged and understood. While this development has raised relevant concerns, some reluctance around AI/ML adaptation may stem from a sense of human vulnerability. When preconceived ideologies are set aside, it becomes clear that many fear-based responses to AI/ML are rooted in its levels of efficiency that surpass human capabilities. The reality is that the increased efficiency provided by AI/ML tools can reduce organizational expenses, minimize errors, and eliminate the need for extensive revision processes.
One of the most compelling aspects of AI/ML in the legal field is their ability to revolutionize traditionally time-intensive tasks like research and data analysis. They can sift through vast amounts of legal data in a fraction of the time it takes us humans, offering insights, precedents, and improved accuracy that could shape legal strategies and outcomes. AI can be leveraged to pinpoint relevant precedents and legal principles. It can then be coupled with custom ML algorithms to quickly identify patterns, correlations, and similarities between cases, assisting lawyers in uncovering key arguments and supporting authorities to improve their positions. Together, they can analyze historical case data and outcomes to predict the likelihood of success in similar cases and offer clients potentially more accurate assessments of their legal positions and potential risks.
AI/ML can be perceived as an “invisible” helper that supports better time management. With the power and ability to stay on top of deadlines and compliance requirements, AI/ML can be leveraged to track and manage timelines for legal tasks, such as court filings, document submissions, and client communications, or assist in compliance-related operations like license renewals and report submissions. This astounding ability to predict our desires can be utilized for delivering personalized and tailored services focused on clients’ unique needs and circumstances, leading to customized recommendations and strategies for their legal challenges.
AI/ML systems can capture potential inconsistencies or gaps in documents and contracts, increasing accuracy and reducing costly mistakes while automating manual tasks and streamlining processes to reduce time spent on routine work. These efficiencies can free up time to focus on more complex and strategic work, boosting productivity, optimizing resources, and enhancing overall performance. They can lead to lower billable hours and faster case resolutions, impersonating cost savings to law firms and their clients.
While embracing AI/ML technologies, we must also acknowledge and address potential risks associated with their use. What are some of the challenges that accompany AI/ML advancements? And how can we approach them with contemplative deliberation and responsible proactivity? The initial considerations for most law firms revolve around cybersecurity and confidentiality.
Some fundamental forms of confidentiality attacks on AI/ML systems that should be considered are:
- Model stealing is cloning or replicating an AI/ML model without permission. The attacker sends queries to the model and observes responses, parameters, structure, and logic to recreate them for their purposes. To minimize the risk of model stealing, consider limiting access and exposure to your model and utilizing encryption, obfuscation, and added noise on the model’s outputs.
- Model inversion is recovering information from an AI/ML model’s outputs. The attacker analyzes the model’s outputs for different inputs to determine the characteristics of the data used to train the model or reconstruct the data. To minimize the risk of model inversion, leverage data anonymization or encryption, limit the amount of information from model outputs, and apply applicable privacy controls.
- Backdoored ML embeds hidden functionality in an AI/ML model that can be leveraged as required. Modifying training data, code, or updates creates a backdoor that triggers the model to behave abnormally or maliciously on specific inputs or conditions. To minimize the risk of backdoor attacks, pay attention to the integrity and source of training data, code, and updates and apply anomaly detection and verification controls.
- Membership inference is similar to model inversion as it focuses on determining if an individual’s personal information has been used to train an AI/ML model to access that personal information. To minimize the risk of membership inference, look at techniques like differential privacy (adding noise to the data), adversarial training (training the model on regular and adversarial examples), and regularisation (preventing overfitting in the model).
Regarding integrity, ML algorithms are vulnerable to tampering, leading to unauthorized changes to data or systems. If the system’s integrity is altered, the data and firm guidance issued could be inaccurate, or the system could be non-compliant with client or regulatory requirements.
Some forms of integrity attacks on AI/ML systems that should be considered are:
- Data poisoning—This can compromise the quality or integrity of the data used to train or update an AI/ML model. The attacker manipulates the model’s behavior or performance by injecting malicious or misleading data into the training set. To minimize the risk of data poisoning, verify the source and validity of your data, use data cleaning and preprocessing techniques, and monitor the model’s accuracy and outputs.
- Input manipulation—The attacker deliberately alters input data to mislead the AI/ML model. To minimize risk, leverage input validation, such as checking the input data for anomalies (unexpected values or patterns) and rejecting inputs that are likely to be malicious.
- Adversarial attacks—The goal here is to cause the AI/ML model to make a mistake, a misclassification, or even perform a new task by including alterations in the input, leading the AI/ML model to make incorrect predictions. As the AI/ML model operates on previously seen data, this data quality significantly impacts the resulting models’ performance. To minimize risk, define your threat model, validate and sanitize your inputs, train your model with adversarial examples, and monitor and audit your outputs.
- Supply chain—Similar to software development, AI/ML model tech stacks rely on various third-party libraries that could have been compromised by malicious third parties or had their third-party repositories of AI/ML models compromised. To minimize risk, leverage your third-party risk management and secure software development practices, focusing on various supply chain stages, including data collection, model development, deployment, and maintenance.
Finally, legal entities employing AI/ML systems should reinforce their cybersecurity to protect against threats that may disrupt services or infrastructure by causing downtime, impacting firm operations, leveraging ransomware, or launching denial-of-service attacks. Securing an AI/ML system can be unsettling initially, much like securing any other legal software. The process will vary depending on the use case, but it typically follows a structure similar to technical and organizational security that defends against threats and vulnerabilities.
You can prepare by implementing AI governance and either modify or establish policies, processes, and controls to ensure your AI systems are developed, deployed, and used responsibly and ethically and are aligned with your organization’s expectations and risk tolerance. This includes defining roles and responsibilities for AI governance; implementing data governance practices to ensure accurate, reliable, and secure utilization of data; creating guidelines for developing and validating AI models (testing for bias, fairness, and accuracy); considering ethical and compliance requirements and updating risk management processes and training and awareness programs to possess AI needs.
Once your organization has identified a need for an AI/ML system and governance protocol is in place, it’s time to evaluate your risk. Conducting a risk assessment is critical as it allows you to understand the system’s business requirements, data types, and access requirements and then define your security requirements for the system, considering data sensitivity, regulatory requirements, and potential threats.
If the AI/ML system is Software as a Service (SaaS) or Commercial party Off-the-Shelf (COTS), you must invoke appropriate third-party risk management processes. Often, this involves:
- Ensuring the proper contractual clauses are in place to protect your organization and its information.
- Determining if a vendor can comply with organizational security policies.
- Investigating whether the AI/ML model was created using secure coding practices, validating inputs, and then tested for vulnerabilities to prevent attacks such as model poisoning or evasion.
Suppose you want to develop a unique set of AI/ML tools. In that case, you will want to consider the source of components you are utilizing carefully. Apply model attack prevention to the system as a part of the data science (add noise, make model smaller, hide parameters). Protect the AI/ML model with secure coding practices, validating inputs, and testing for vulnerabilities to prevent attacks such as model poisoning or evasion. Implement appropriate throttles and logging to monitor access to your model and ensure your code can detect abuse, recognize standard input manipulations, and limit the amount of data in rest and transit and the time it is stored.
When you are comfortable purchasing or developing a secure AI/ML system, it is time to ensure the technology is rolled out and supported securely. To do this, you will want to:
- Implement secure data storage practices, such as encryption, access controls, and regular data backups, to protect sensitive data use used by the AI/ML system.
- Use secure protocols (HTTPS) to encrypt data in transit and at rest and prevent unauthorized access, interception, and tampering.
- Anonymize sensitive data used in the AI/ML system to protect user privacy and comply with regulations.
- Apply the necessary role-based access controls (RBAC) to restrict access to the AI/ML system and its data based on the majority of minor privilege requirements.
- Configure monitoring and logging to track the AI/ML system’s behavior and detect suspicious activity.
- Quickly update and patch the AI/ML system and its components to protect against new vulnerabilities and exploits.
- Update security operation processes to include new AI/required controls.
- Conduct regular security audits and monitor the AI/ML system for unusual or suspicious activity.
As members of the legal profession begin to understand and embrace AI/ML technologies widely, we must remain intentional about addressing the legitimate fears and challenges they present. Accordingly, it would be wise for legal service communities to navigate the complexities of AI/ML with a nuanced approach that balances innovation and caution. If we manage it responsibly, exercise some faith, and remain vigilant about ensuring the proper controls and governance are in place, we should all be able to progress together.
David Whale is a Director of Information Security with a passion for enabling business innovation in a risk-managed environment. With over 20 years of experience in cybersecurity across professional services, construction and legal industries, he brings a wealth of knowledge and insights to his writing. You may recognize David from the podcast and panel discussions he has hosted with ILTA. He holds a degree in Business and his CISA and CRISC in security.