Star Trek’s Holodeck Recreated As Virtual Training Ground For Next-Gen Robots
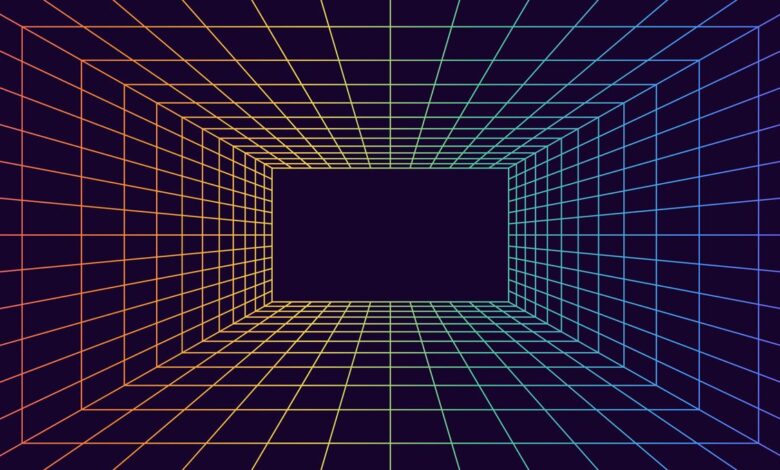
Generations of Star Trek fans have wondered what it might be like to make first contact with a new species, escape an awkward social interaction with an emergency beam-out, or experience warp speed on a starship (just don’t go past warp 10, whatever you do!). While a lot of the incredible tech depicted in the franchise remains in the realms of fiction for now, some Trek-inspired innovations have become a reality. Thanks to a team of engineers at the University of Pennsylvania, we can now add a holodeck to that list.
To be clear, and because we were just as disappointed as you, we’re not talking about a holographic environment where humans can explore and interact with characters – we’re still a way off that. But the Holodeck system (yep, that’s what it’s called), created by a team at Penn Engineering and their collaborators, can build pretty much any 3D environment you can think up. You just have to ask.
“We can use language to control it,” explained co-creator Yue Yang in a statement. “You can easily describe whatever environments you want and train the embodied AI [artificial intelligence] agents.”
The holodeck system depicted in Star Trek series like The Next Generation and Voyager is an infinitely customizable virtual environment, capable of turning quite basic verbal commands into complete, simulated worlds. In reality, these types of environments – albeit on a smaller scale – have important applications in training robots.
Creating a virtual world, however, is a time-consuming process. “Artists manually create these environments [and] could spend a week building a single environment,” Yang said. The problem with this is that to train a robot to navigate real life, you need to trial it in a variety of different environments. Generative AI, which has exploded in recent months, seemed the clear solution.
“Generative AI systems like ChatGPT are trained on trillions of words, and image generators like Midjourney and DALLE are trained on billions of images,” said Chris Callison-Burch, Associate Professor in Computer and Information Science at the University of Pennsylvania.
Holodeck essentially engages a large language model (LLM) – the system that powers chatbots like ChatGPT – in a conversation that allows it to pick out the parameters of the environment the user wants. The system accesses a digital library of millions of premade objects called Objaverse, from which it can select suitable furnishings, and a layout design module constrains the spatial configuration so that the objects end up in logical places in the room.
How Holodeck builds up an environment piece by piece, by essentially having a conversation with an LLM to discern all the necessary parameters.
In practice, that means if you ask it for the apartment of a person who owns a cat, Holodeck will ensure the finished room contains all the pieces of furniture you’d expect, including a cat tree.
The team compared Holodeck to an earlier tool called ProcTHOR, generating 120 scenes and asking students to choose their preferences in a blinded test. Holodeck outperformed its competitor in every way. The system also coped well when asked to create more unusual spaces, from science labs to wine cellars.
But, “The ultimate test of Holodeck,” according to co-creator Assistant Professor Mark Yatskar, “is using it to help robots interact with their environment more safely by preparing them to inhabit places they’ve never been before.”
Virtual training is often limited to mostly residential spaces, but there are lots of strange new worlds out there that a robot may need to navigate. Using Holodeck to produce the environments, rather than the predecessor tool, had a marked positive effect – for example, a robot pre-trained on 100 virtual music rooms created by Holodeck was able to locate a piano 30 percent of the time, versus just 6 percent after training with ProcTHOR.
You might not be able to use it to run a Dixon Hill holonovel, but this Holodeck could soon be making a big impact in the world of robotics.
The study will be presented at the 2024 IEEE/CVF Computer Vision and Pattern Recognition Conference. A preprint paper, which has not been peer-reviewed, is available via arXiv.