Superposition Guy’s Podcast — Catherine Vollgraff Heidweiller, Product Manager, Google Quantum AI The Superposition Guy’s Podcast: Workforce Development
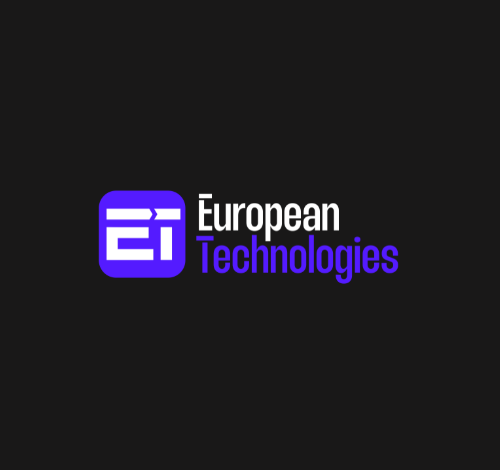
“The Superposition Guy’s Podcast”, hosted by Yuval Boger, CMO at QuEra Computing
Catherine Vollgraff Heidweiller, product manager at Google Quantum AI, is interviewed by Yuval Boger. Catherine describes the development of full-stack quantum computing, the importance of their 2019 quantum supremacy milestone, Google’s product focus, and the early customers they work with. We discuss the evaluation of quantum usefulness, their error correction roadmap, the intersection of quantum computing and AI, the societal responsibilities of quantum development, and much more.
Listen on Spotify — here
Full Transcript
Catherine Vollgraff Heidweiller, product manager at Google Quantum AI, is interviewed by Yuval Boger. Catherine describes the development of full-stack quantum computing, the importance of their 2019 quantum supremacy milestone, Google’s product focus, and the early customers they work with. We discuss the evaluation of quantum usefulness, their error correction roadmap, the intersection of quantum computing and AI, the societal responsibilities of quantum development, and much more.
Full Transcript
Yuval Boger: Hello Catherine, and thank you for joining me today.
Catherine: Hi.
Yuval: So who are you and what do you do?
Catherine: I’m Catherine. I work for Google Quantum AI, which is a big R&D team under Google Research. We build quantum computers, and we develop quantum algorithms and applications. So really, it’s a full stack effort, and we aim to develop fully scaled, fully error-corrected quantum computers and make them useful and available to the world. Personally, I’m a mathematical physicist by training. My first encounter with quantum computing was at university where I worked for a little while on the hidden subgroup problem. I have been with Google for six years now, and I currently lead the product management effort across hardware and software, which is really all about kind of closing the gap between invention and us delivering a useful new compute capability to the world, hopefully.
Yuval: If you’re a product manager, that means there may be products coming. Are there Google quantum products?
Catherine: Yeah, there are. Our core product goal is to develop a full-stack quantum hardware and software offering with fault-tolerant, scaled quantum hardware and software, which allows users to solve commercially and societally-relevant problems. Of course, this is a long-term goal, which we hope to reach in lockstep with the developments in hardware and applications research.
We’re currently in what I would call the early product iterations. Our full-stack product currently serves many of our internal users, as well as a small circle of external expert users.
Today’s quantum computers are a very scarce resource unless you choose to invest a lot in scaling them horizontally. We decide to invest more in improving them rapidly toward the commercial era and co-develop with some users where it makes sense.
We also have a portfolio of open-source products focused on R&D progress. We invest in these products, believing they will help drive the field forward. In the applications and algorithms space, we have OpenFermion for quantum simulation. We also have Qualtran, a new tool currently under heavy development, which allows you to estimate the quantum compute costs associated with an algorithm, enabling rapid iteration on quantum algorithms and a quick assessment of their usefulness.
We also have some research tools for error correction research. Part of our full-stack product is open source, particularly the top of the stack and some simulators.
Yuval: You mentioned useful applications. How do you measure usefulness? Google made lots of headlines many years ago, or not that many years ago, with their quantum supremacy experiment, which many people thought wonderful demo, useless algorithm. How do you measure usefulness?
Catherine: So I think there’s probably two questions there. Maybe there’s a question around how we think about that 2019 milestone, and maybe another question of how we define usefulness more broadly. Let’s address the first one first.
The 2019 milestone was really a first-flight moment, demonstrating that quantum computers can indeed outperform classical computers on benchmark tasks. It was a field-defining moment that showed the world it is worthwhile to invest time in building quantum computers. This was the first step on a trajectory. As predicted, researchers have since found methods to run this benchmark faster on classical computers, but at the same time, quantum computers have been continuing to improve.
You can see that if you compare the trajectory of classical compute power with that of quantum compute power, classical computers essentially can’t catch up anymore since we reached that first benchmark. But this was just a benchmarking task, a basic way of measuring raw compute performance. I don’t know if that answers the question about the 2019 experiment.
More broadly, when considering the usefulness of a quantum computer, I think there are two important lenses to consider. One is how useful the quantum hardware is—how powerful it is as a generally programmable computer. The second is how useful the application you’re running on the quantum computer is. These are two separate questions, both critical to address as you evolve your research.
Shall I go into these two a little bit more?
Yuval: Absolutely.
Catherine: Cool. So, yeah, let’s start with this compute power piece. To determine how powerful the quantum computer is, we’ve done a lot of thinking about how to assess this in a comprehensive way. Ultimately, we concluded that we should look at the algorithmic complexity a processor can handle well. The metric that best represents this is called effective quantum volume. There’s a paper and blog post on this for anyone interested in the details.
Effective quantum volume measures the size of the program that can run on your quantum computer without noise degrading the quantum state of the output. It measures the number of quantum operations or gates that contribute to a measurement outcome. It’s interesting that coverage of quantum computing hardware often focuses on the number of qubits in a processor. I personally think this is misleading because a large number of low-quality qubits isn’t helpful for any task.
We try to have a more comprehensive view and evaluate not just the physical qubit count but also the physical qubit qualities, coherence, gate fidelity, logical qubit error rate, speed (operations per unit of time), and connectivity—how the qubits interact and how you can map an algorithm onto the chip.
That’s the raw compute power of the quantum computer. Next comes the question of how useful the application is that you’re running. There are a few things to consider here. You want to assess the real-world significance of the application. What question does it help you answer? Is that question significant commercially, scientifically, societally, or otherwise? Is it an interesting question that your computation helps answer?
The second piece is how this algorithm’s performance compares to known classical algorithms that attempt to compute the same thing. Does the quantum algorithm, when run on a quantum computer, provide a super-quadratic speedup over known classical algorithms, even when run on the world’s best classical computers? There’ve been a lot of interesting results in the algorithm space showing there’s a big overhead when you run something on a quantum computer. You really need a super-quadratic speedup for the algorithm to be practically useful.
Regarding real-world significance and algorithm performance, I believe independent validation is important. Peer reviews can help the community confirm claims about the usefulness of the application running on your quantum computer.
Yuval: When you think about these early customers that you work with, do they skew towards a particular application, or do they come from one particular vertical?
Catherine: So I would say that they do have a similar profile in a sense. And that is because of what can be done on current-day hardware.
So if you think about early users of the full-stack offering, by definition, they should be working on projects that are feasible on current-day hardware. Quantum computers have been used so far for many interesting scientific applications. We believe it’s likely that various useful applications will emerge with current-day hardware.
However, based on the state of applications research, I expect that the major commercial opportunities will be in the fault-tolerant regime. These early users tend to be people working on scientific applications, at least for the moment. They could be exploring new physics or doing something that is a useful application of the current-day hardware.
One thing to add is that there are many sensational headlines, but in reality, we haven’t yet seen the publication of a result where someone did a commercially relevant computation on a quantum computer that couldn’t be simulated on supercomputers. It’s a very exciting area of progress. I think we’ll cross that frontier as an industry at some point, but we’re not quite there yet.
Personally, I think pretending to have all these customers solving high-value problems on your quantum computer seems a little unrealistic.
Yuval: You mentioned logical qubits and error correction, and also an error correction framework or an open-source project that you make available. Where is Google on error correction?
Catherine: We are currently working on building a long-lived logical qubit. So specifically, what we’re trying to achieve is having one error fewer in one million algorithmic steps. So we’re working very hard to make progress in many areas like, you know, reducing the physical errors with improved qubit design, fabrication, gate control, readout, reset times, you know, qubit stability, faster real time decoding, you know, scaling up processor size. There are tons of areas of development to get to this, you know, long-lived logical qubit milestone. I don’t know if it would be helpful to talk a little bit about our most recent big result, which we are currently building on.
Yuval: Please.
Catherine: Cool. Yeah. So in 2022, we demonstrated that a that basically error correction can work and, hence that quantum computers can scale. So what we showed is that you can increase the size of the chip, but reduce the errors using error correction. So we compared a 17 physical qubit logical qubit to a 49 physical qubit logical qubit and demonstrated that the 49 one had lower errors. So this is kind of a prototype of a logical qubit, and we’re currently really improving on every front and scaling it up to get to a long-lived logical qubit.
Yuval: Long live the logical qubit. That sounds very British. Many companies are investing in AI these days, sometimes to the detriment of quantum computing. We certainly see budgets shifting from quantum to AI sometimes. How much do you feel that quantum and AI are intermingled and codependent?
Catherine: Yeah, it’s an interesting question. Of course, our team is called Quantum AI for a reason. We believe that some of the most important applications of quantum computers will sit in the quantum machine learning space.
There is an interlocking of timelines in the sense that timelines for developing quantum computers are loosely coupled to timelines for what quantum AI applications will be possible. As we scale error-corrected quantum computers, we will gradually unlock new areas of quantum machine learning applications that will become feasible.
To illustrate this, current applications research suggests that quantum machine learning on quantum data is expected to require about 100,000 to 500,000 physical qubits. Quantum machine learning on classical data is expected to require 500,000 to 5 million physical qubits. In that sense, the two are tightly coupled.
But I think people often confuse quantum machine learning on quantum or classical data with classical machine learning on classical data. The two are not tightly coupled, and their timelines are probably about 20 years apart.
Yuval: Speaking of AI, AI was sort of lurking in the shadows for decades. And then chatGPT came along sort of, and I sometimes say an overnight sensation 30 years in the making, maybe more than 30. What do you see as the chatGPT moment of quantum, and how soon would you expect it to be?
Catherine: Yeah, so that’s a very interesting one. You know, what will be the big moment in quantum?
I would imagine the big moment would be us using a quantum computer to do something really meaningful with societal impact. Quantum computers have a great deal of promise in delivering societal impact. There are many promising early results in the applications research space, particularly in areas like energy generation and storage, as well as drug discovery.
However, on the other hand, it could also be something much simpler—an “aha” moment where we apply the technology in a practically impactful way. It makes me think of the example from 2012 in AI, when Google Research published the “cat paper.” I don’t know if you’ve heard about it. Shall I tell you about it?
Yuval: Please go ahead.
Catherine: Yeah. So in 2012, we had this kind of important applied breakthrough in AI, where a neural network actually learned to recognize common objects from unlabeled data. And that really massively pushed forward the state of the art in image classification.
The question was, if we show a neural network YouTube videos for a week, what would it learn? The hypothesis was that it would learn to recognize common objects. And indeed, it turned out that one of the artificial neurons actually learned to recognize cats. This was impressive because the network had never been told what a cat is or been given even a single image labeled as a cat. Instead, it really learned it. It discovered what a cat looked like by itself, just from unlabeled YouTube stills.
In that way, the world suddenly had programs that could do self-taught learning. So, maybe in quantum, we will have this kind of “cat moment” as well, like a Schrödinger’s cat paper. Something that suddenly shows something really basic but of very high importance. So, yeah, let’s see. Time will tell. I have faith in the algorithms community.
Yuval: How do you feel about the social responsibility of companies building quantum computers? Is there a responsibility to make them equally accessible to all corners of the world? Is there a responsibility to use them only for beneficial applications as opposed to cracking the financial system? What do you think about that?
Catherine: I think there are a few lenses to look at this through. As a product manager, I naturally think about it through the product lens. If you build a very powerful tool, a generally programmable computer, you have to do that responsibly. It’s through our products that we bring new innovations to the world, and we need to think about the impact of doing that.
At Google, there’s quite a formalized process around this. We have a set of AI principles for building new technologies responsibly, which actually carry over well to quantum computing. Some examples include ensuring that the product should be socially beneficial, designed and tested for safety, and subject to appropriate human direction and control.
We also consciously avoid certain application areas, such as weapons and surveillance that violate internationally accepted norms. From a product perspective, it’s very much about what tools you offer the world, how you build safeguards, and how you ensure proper testing to bring something constructive, safe, and helpful to the world.
But there’s also a broader question around preventing parts of society from being left behind. How do we avoid worsening existing inequalities by ensuring people are educated about quantum computing and have access to it? We must ensure the “left behind by technology” issue isn’t exacerbated in the quantum space. Additionally, how do we ensure we hire diverse groups so that the people who build these quantum computers come from different parts of society? This way, we can reinforce forces toward greater equality.
Yuval: As we get close to the end of our conversation, I’m curious, professionally speaking, what keeps you up at night?
Catherine: That is a very good question as well. What keeps me up at night? I think it’s this societal impact, this question of building responsibly. In these early product iterations, it’s tough to figure out what practical safeguards to put in place.
With every product launch, you must think carefully: If I were the most evil person in the world, what’s the worst possible thing I could do with this? It’s not how my brain naturally works, so I have to make a real effort to consider these more negative scenarios.
Maybe that’s the most esoteric question that can really keep you up at night.
Yuval: And last, a hypothetical: if you could have dinner with one of the quantum greats dead or alive, who would that be?
Catherine: Oh, yeah. It doesn’t have to be one person. Can I make a dinner party?
Yuval: You can make a dinner, you can make whatever you want. This is a hypothetical.
Catherine: OK, cool. Well, at the head of the table, of course, you have Richard Feynman. But I can’t limit myself to just him because it’s too standard. Frankly, I think it would be nice to have Richard Feynman as a grandfather, maybe together with David Attenborough as my other grandfather. But anyway, he’ll be one person there.
To add to that, I think I would also have to include Ada Lovelace. Although she wasn’t a quantum computer scientist, she was a computer scientist, and without the concept of computers, we wouldn’t have the idea of quantum computers. Hopefully, that’s allowed. She would have been fascinating—this lady computer scientist, daughter of a romantic poet, friends with Michael Faraday and Charles Dickens. It would have been fantastic to have dinner with her.
Another personal addition would be Stephen Hawking, even though I don’t know if this one’s “legal.” I initially started life much more interested in astrophysics and only later learned about quantum computing. It’s impossible not to be fascinated by quantum gravity, a field of theoretical physics that seeks to describe gravity according to the principles of quantum mechanics.
When I was in the math department at Cambridge, Stephen Hawking was this elusive hero—omnipresent but rarely seen, cruising down the hallways occasionally. Students would excitedly whisper, “Oh, I saw Stephen Hawking!” He was such a fascinating person, and I would have loved to have dinner with him around the end of his PhD, probably in the mid-1960s. I could chat about singularities, the geometry of space-time, and just learn something from him.
Yuval: Catherine, thank you so much for joining me today.
Catherine: It was great to see you. Bye!
To subscribe to the audio podcast, please Spotify here
If you found this article to be informative, you can explore more current quantum news here, exclusives, interviews, and podcasts.