The future of data science: Where are we headed? – Digital Transformation News
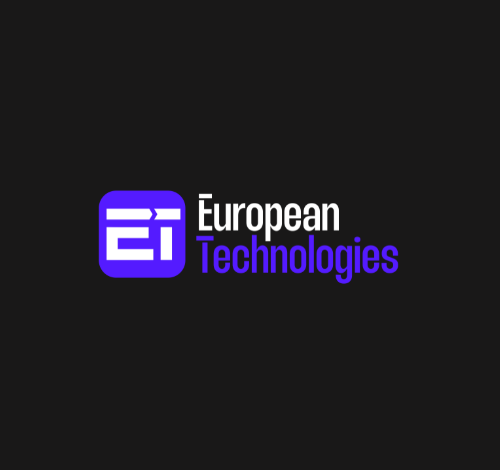
By Sunil Sharma
Advancements in data analysis technology
Tasks that once took years on classical computers can now be completed in minutes with quantum computing. This groundbreaking intersection of computer science and physics has substantial potential to overcome data analytics challenges. Key quantum computing concepts include superposition, entanglement, quantum gates, algorithms, supremacy, and error correction.
Global enterprises also leverage advanced machine learning techniques for more mature and nuanced visions to better serve their customers. Merging analytics with automated machine learning and AI, they prioritize safety, ethics, and evolving regulations. As machine learning grows beyond predictive models to faster, more profound, actionable, and compliant data-driven breakthroughs, several trends will shape the future of data science.
- Augmented analytics: Augmented analytics employs machine learning and AI to enhance data preparation, insight generation, and explanation in Business Intelligence (BI) systems. It automates various aspects of data science, machine learning, and AI model development, aiding both expert and citizen data scientists. With advancing AI and machine learning, integrating natural language processing (NLP) and automated insights facilitates improved data interaction. Amidst the latest trends in data science, augmented analytics is poised to streamline information extraction from datasets, expanding organizational knowledge for informed decision-making.
- Multimodal AI: Multimodal AI surpasses conventional single-mode data processing by incorporating various input types—text, images, and audio—to glean insights. This approach mimics human aptitude in handling diverse information, enabling businesses to harness generative AI effectively by integrating text, conversation, images, and videos. The practical applications of multimodal AI are extensive. For instance, it enhances diagnostics in healthcare by analyzing medical imagery in the context of a patient’s history and genetics. In tech companies, it boosts productivity by extending design and coding capabilities to employees lacking significant expertise in those domains.
- Agentic AI: An emerging trend in data science, agentic AI features advanced autonomous agents that proactively act rather than merely responding to user inputs or adhering to predetermined programming. These agents comprehend their environment, establish objectives, and attain them without direct human intervention. In the BFSI sector, financial agentic AI can actively manage investment portfolios using adaptive strategies that respond to real-time market
changes. Likewise, an AI agent can gather data, analyze patterns, and initiate preventive actions in environmental monitoring against potential hazards like forest fires. - Streaming analytics : Streaming analytics empowers data science experts to analyze information in real time, departing from the retrospective nature of batch processing. This real-time approach allows for a profound understanding of events as they unfold, enabling users to identify and address threats proactively. Unlike traditional methods, organizations employing streaming analytics can act preemptively, enhancing operational management. A prime example is its application in weather forecasting, where scientists analyze radar images in real-time to predict localized weather patterns. Retail companies also leverage streaming analytics to foresee customer behavior, strategically sending timely discount coupons and predicting high-selling items on specific days. This dynamic technology transforms data analysis from reactive to proactive decision-making.
- Edge analytics : With billions of connected devices amassing data, the value of edge analytics is rapidly increasing as it makes data processing possible at the source, reducing latency. While establishing infrastructure for edge analytics initially poses a considerable financial outlay, these costs diminish over time. Processing data at the edge not only provides real-time insights and uncovers novel patterns but also ensures compliance with data privacy regulations. Faster data analysis facilitates quicker decision-making to improve customer experience with greater personalization and streamlined buyer journey. Gartner predicts that by 2025, over 55% of deep neural network data analysis will occur at the edge upon capture, a substantial rise from less than 10% in 2021.
- Python and Pandas: Python, a widely recognized programming language, boasts a robust ecosystem of open-source libraries and tools that empower scientists to construct sophisticated applications. Particularly advantageous for data science, Python facilitates intricate analyses across diverse datasets. Pandas, a Python library, furnishes data structures and operations tailored for manipulating numerical tables and two-dimensional arrays. Pandas prove versatile, whether summarizing entire tables, computing statistics, conducting linear regressions, or generating histograms on subsets. Its technology bolsters data science through an intuitive toolkit for handling vast datasets in exploratory analyses, providing faster access to subsets than traditional languages like R.
Also read: Unleashing the power of quantum computing: A transformative frontier for India
Navigating the Challenges
Balancing Innovation and Accountability is essential. As data science technologies continually evolve, supporting business transformation, enterprises face the dual challenge of balancing innovation and accountability. Utilizing data to enhance operational efficiency and customer experience requires deciphering information patterns in vast datasets and prioritizing the management of AI risks. Ensuring data accuracy, privacy, and responsible analysis becomes paramount.
Staying Ahead of the Curve
Embracing these emerging trends and technologies positions organizations at the forefront of data science. This knowledge is critical for navigating the ever-changing landscape of this dynamic field and leveraging data’s full potential.
(The author is Global Head of Data Science and Digital Engineering – CriticalRiver. Views expressed are personal and not necessarily those of financialexpress.com).