The No. 1 risk companies see in gen AI usage isn’t hallucinations
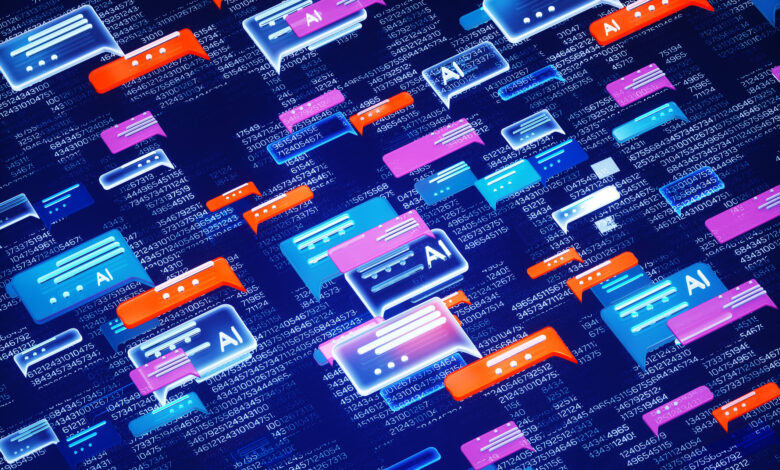
Andriy Onufriyenko | Moment | Getty Images
The perks of generative artificial intelligence have a flip side, including hallucinations, code errors, copyright infringement, perpetuated bias and — what organizations worry about most — data leaks.
Most companies (77%) report successful gen AI pilots, according to a recent survey from Alteryx, but 80% cite data privacy and security concerns as the top challenges in scaling AI. Meanwhile, 45% of organizations encountered unintended data exposure when implementing AI solutions, according to AvePoint’s 2024 AI and Information Management Report. Microsoft AI’s leak of 38 terabytes of data late last year is just one example of how big this problem can get.
“AI has certainly amplified and accelerated some of the challenges that we see around data management,” said Dana Simberkoff, chief risk, privacy and information security officer at AvePoint, which provides technology to help organizations manage, migrate and protect their data in the cloud and on premise.
Simberkoff explains that much of this leaked information is unstructured data that’s sitting in collaboration spaces, unprotected but previously undiscovered due to the difficulty around finding it. “It’s often what we call dark data,” Simberkoff said.
Arvind Jain, CEO and cofounder of enterprise search platform Glean, which creates gen AI-powered enterprise-wide search tools and was named this week to the 2024 CNBC Disruptor 50 list, says there’s immense pressure on chief information officers and related roles to deploy AI, leaving a lot of room for error in the race to modernity. “It was so hard to find anything. Nobody knows where to look,” said Jain. “That’s the thing that AI fundamentally changes. We don’t have to go and look anywhere anymore. You just have to ask a question.”
Jain says most enterprise data has some level of privacy to it, and that permissions that aren’t shored up leave crucial information untethered. While his own search platform operates with organizational permissions in mind, it’s up to leaders to manage their data before augmenting it with AI.
Shining a light on unprotected ‘dark data’
It’s not just customer and employee personal identifiable information escaping beyond the organization’s walls to worry about. From a former employee’s termination letter to confidential discussions about mergers and acquisitions, there are myriad kinds of sensitive documents that can cause trouble if accessed by the wrong parties within an organization. Whether it’s employee dissatisfaction, insider training or something in between, the risks are tangible.
Even without AI, that information that would leak out is still unprotected. “Not knowing is never better,” said Simberkoff. “Shining a light on that dark data, all of a sudden, there it is, and you can’t ignore it anymore.”
Simberkoff lives by the mantra, “We protect what we treasure, and we improve what we measure.”
So how do leaders improve data permissions and protections in light of or, ideally, before AI implementation?
“It’s not turning on AI. It’s the six steps beforehand of understanding your data,” said Jason Hardy, chief technology officer for AI at data infrastructure company Hitachi Vantara. He said this includes logging data, using vendor-provided tools to feed that data through structuring and search protocols, and consistently vetting your information over time.
Hardy adds that both ends of the spectrum matter: policies to prevent leaks and enforcement to manage information if it does get out.
“It does come down to a lot of training,” he said. “It’s making your end users aware of the information you’re responsible for. We have approved tools to use, but also as we bring them into our systems, let’s have those safeguards.”
Simberkoff says it’s crucial to prioritize high-risk information in your organization’s ecosystem and practice data labeling, classification and tagging.
An anti-rushing approach to AI implementation
One thing Simberkoff says many leaders forget is that it’s okay to pause in the AI adoption journey. “Organizations may sort of rush to adopt AI and then have to pause. That’s okay,” she said. “One of the things that we’ve seen that can be very effective is thinking about this in incremental steps, which is that you can start off with something like an acceptable use policy and a strategy, but it’s always good to test the waters with a pilot.”
Plus, Simberkoff says regulation and laws are changing, so having a good understanding of your data over time just makes sense.
Here, Hardy believes an ounce of prevention is equal to a pound of cure. “Do the right thing up front and you’re not going to make the front page of pick-your-popular-news-vendor.”
Simberkoff reminds leaders that AI is an imperfect technology. “We know that these algorithms hallucinate, that they make mistakes, that they’re only as good as the data entered into them,” she said. “When you’re using AI, it’s really important to make sure that you’re checking it and that you’re using it for its purpose.”
That means user education is a must. After all, she likens AI to a valuable intern. “You can give them assignments, but you always want to check, make sure that you know what they’re doing is correct and that they’re not off going down a tangent,” Simberkoff said.
Jain recommends all companies, especially large enterprises, have a centralized AI strategy to vet tools and determine what content they’re going to connect to the data set. However, limited information provides limited value, so connecting as much information as possible while maintaining appropriate permissions makes the most sense, he says. In addition, a soft rollout is a good idea to test the waters of a new program before embracing it company-wide.
Even with AI uncovering poor data hygiene, Simberkoff says the juice is well worth the squeeze. “AI is our best friend,” she said. “It’s going to really push the organization to take those steps that they should have been taking all along.”