The Potential Impact of Generative AI on Government CIOs
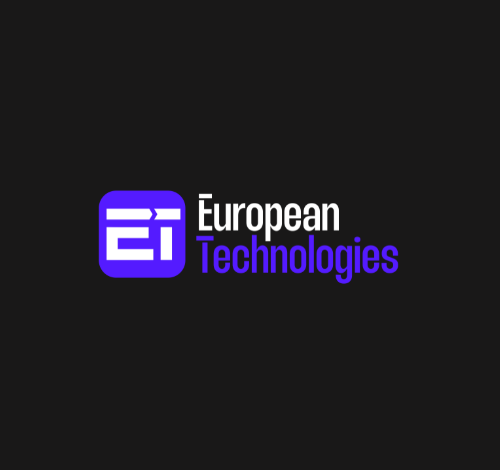
By Ben Kaner
Generative AI applications such as ChatGPT, GPT-4, Gemini and others have entered into the mainstream conversation of government executives wanting to understand the technology, its opportunities and its risks.
Generative AI applications can be used to generate value across a wide range of government use cases, both internal and facing citizens. However, delivering value through these applications relies on gaining and maintaining the trust of the community and the government workforce alike. This requires strong governance and risk management, and a comprehensive understanding and management of the inherent limitations of the technology.
Potential Significant Value for Government Service Delivery and Operations
Properly trained generative AI systems, when deployed alongside other automation tools, have the potential to greatly enhance government service delivery and operations. Here are some areas where generative AI can be helpful:
- Text generation: The ability to compose various types of communications for different target audiences, such as young people, marginalized communities, or those who speak different languages, presents opportunities for increased personalization in government services.
- Text summarization: Summarizing long or complex related cases for case managers to support improved decision making. Similarly, summarizing complex or abstruse documents for laypeople or policymakers could improve productivity.
- Text classification: Large language models (LLMs) enable classification and collation of the large volume of unstructured text, improving the quality of the data used to support decision intelligence and policy development.
- Sentiment extraction: Text classification could also be used for sentiment analysis of citizen engagement and communication.
Limitations and Risks That Currently Exist
Many government policies require transparency regarding the use of GenAI, so the Chief Information Officer (CIO) must understand this. There are five main sources of risk:
- Accuracy: LLMs are not cognitive models, but statistical ones. As the response is very sensitive to the prompt and is often returned in a manner that appears articulate, sometimes significant errors can be hard to spot. While some LLMs can now access the internet or organizational data to provide current results, new updates may unpredictably shift the output of the model, which means that answers may be significantly inconsistent over time.
- Bias: The data used to train the LLM may be incomplete or poor quality, or contain inherent biases. These biases will impede the accuracy of the model’s output.
- Copyright: There is a potential for copyright violations, as the legal status of copyright in the data used to train large language models and in the use of their results is still uncertain in most jurisdictions.
- Privacy: Generative AI systems available directly to the public are often not secure, because they may use your input for further training. They are, therefore, unlikely to meet privacy legislative obligations or community expectations. Government CIOs must take immediate steps to ensure no sensitive or private information is exposed.
- Sensitive/Confidential: Not just the direct information, but the metadata surrounding information submitted to a generative AI system and the response can compromise sensitive data; in the public sector, this includes defense and critical national infrastructure. As such, this is a category of data that needs specific policy attention.
Establishing a Roadmap LLM in Government
Generative AI uses significant quantities of data to create, in essence, a statistically based model of what is likely to be a usable or effective response to a prompt. In government, these models will require to a wide range of government data, much of which may be sensitive. It is therefore important that sensitive data remains controlled — not only from inappropriate public access, but also inappropriate internal access. More constrained models and architectures are needed to retain control over sensitive data.
CIOs should advocate for a policy that minimizes the risk of sensitive information being exposed. This policy should limit the use of open environments, except for low-impact experimentation, while also allowing for careful exploration of technology’s capabilities and identifying use cases that provide greater value than the residual risk. The CIO should prioritize services that have enterprise security and compliance controls, where data is stored within the customer’s infrastructure, over those that do not.
It is important to ensure that acceptable use guidelines require humans to review the output to detect incorrect, misinformed, or biased results until the systems are mature enough to consistently produce accurate results. Furthermore, it is necessary to document and plan for ways to mitigate the risks posed by malicious activities that exploit LLM and generative capability, such as administrative flooding or deepfake attacks, in government processes.
Additional analysis on implication of generative AI applications will be presented at the Gartner IT Symposium 2024 in Kochi, November 11-13 .
(The author is Ben Kaner, Senior Director Analyst at Gartner, and the views expressed in this article are his own)