The Promise of Artificial Intelligence in Clinical Practice
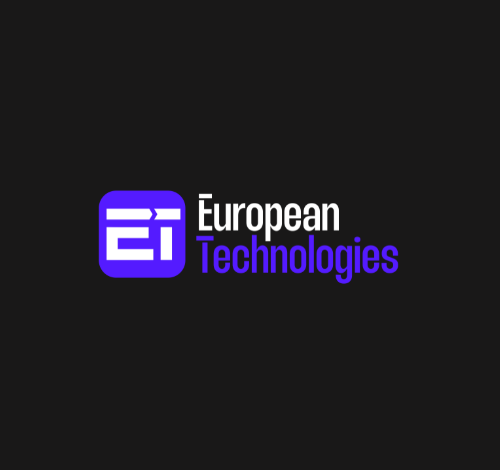
Instead of adding even more burdens to medical practices, artificial intelligence (AI) technology promises to become a tool for a host of clinical needs.
For example, a new report published in JAMA Network Open suggests that AI technology can significantly change medical practice. Physician investigators at Beth Israel Deaconess Medical Center in Boston, Massachusetts, compared a chatbot’s probabilistic reasoning to that of human clinicians. The findings suggest that AI could serve as a useful clinical decision support tool for physicians.
Lead author Adam Rodman, MD, an internist at Beth Israel, said clinicians struggle with probabilistic reasoning, the practice of making decisions based on calculating odds. He and his colleagues evaluated probabilistic reasoning in isolation. They used a previously published national survey of more than 550 practitioners performing probabilistic reasoning on 5 medical cases. Dr Rodman’s team fed the publicly available large language model (LLM) Chat GPT-4 the same series of cases and ran an identical prompt 100 times to generate a range of responses.
The chatbot was tasked with estimating the likelihood of a given diagnosis based on patients’ presentations. Then the chatbot program updated its estimates after given test results, such as chest radiography for pneumonia, mammography for breast cancer, stress test for coronary artery disease, and a urine culture for urinary tract infection.
When test results were positive, it was almost a draw. The chatbot was more accurate in making diagnoses than human clinicians in 2 cases, similarly accurate in 2 cases, and less accurate in 1 case. When tests came back negative, however, the chatbot demonstrated greater accuracy in making diagnoses than human clinicians in all 5 cases.
Probabilistic Reasoning
“I was surprised by these findings,” Dr Rodman said. “Humans do a very poor job at probabilistic reasoning. Whether it’s playing poker, being more scared of flying on an airplane than driving on the highway, filling out an NCAA tournament bracket, or for my purposes predicting whether or not a patient has a pneumonia. We have known about innumeracy for a very long time.”
LLMs are effectively word or token prediction engines built off massive amounts of human text. But they also have emergent properties and may help with differential diagnoses. “In terms of routine use, I think physicians will first interact with LLMs in so-called ‘clerical’ tasks, serving as an AI scribe while in the clinic, assisting with discharge summaries, assisting with many of the other rote tasks that can take up so much time in the day,” Dr Rodman said.
Over the next several years, Dr Rodman said, he expects AI to start making a significant impact in clinical care in the form of clinical decision support. “I imagine LLMs that serve as a co-pilot, looking for early signs of medical errors, making suggestions on additional information to collect or additional diagnoses to consider or even taking the first pass at collecting a history,” he said.
During graduate school at Stanford University in Palo Alto, California, Sarah Hooper, PhD, developed ways to improve the automated processing of cardiac MRIs. Now, as a research scientist at the National Heart, Lung, and Blood Institute’s Imaging AI program, she is working to integrate machine learning across a variety of imaging platforms.
Dr Hooper said machine learning can be inserted into any point of the imaging pipeline to make things more efficient. One program might make it easier to automate parts of the analysis workflow so that a radiologist can spend 5 minutes looking at an image instead of 30. That all translates into patients getting results faster, according to Dr Hooper.
Predicting Cancer Outcomes
Researchers at UT Southwestern Medical Center in Dallas have developed an AI model that analyzes the spatial arrangement of cells in tissue samples. This approach, detailed in Nature Communications, accurately predicted outcomes for cancer patients, marking a significant advancement in using AI for cancer prognosis and personalized treatment strategies.
Tissue samples analyses by pathologists is time-consuming, and interpretations can vary among pathologists. In addition, the human brain can miss subtle features present in pathology images that might provide important clues to a patient’s condition. The new AI model mimics how pathologists read tissue slides, starting with detecting cells in images and their positions. From there, it identifies cell types as well as their morphology and spatial distribution, creating a map in which the arrangement, distribution, and interactions of cells can be analyzed.
The investigators successfully applied this tool to 3 clinical scenarios using pathology slides. They now hope to adopt this model to streamline targeted preventive measures for high-risk populations and optimize treatment selection for individual patients.
Investigators at Arizona State University School of Biological and Health Systems Engineering in Tempe are using AI to sort through decades of medical data to identify treatments for neurological disorders. They are also applying AI models to learn how cancer cells replicate and find solutions to keep older adults safe from life-threatening falls.
One of the investigators, associate professor Bradley Greger, PhD, said that for individual patient care, AI will be useful in digesting large amounts of data from multiple tests, physical exams, and imaging to aid clinical decisions. “Just like any technology, MRI, X-ray, ECG, blood tests, etc., AI can help to improve outcomes for patients through reducing morbidity and mortality,” Dr Greger said.
Dr Rodman agrees and said there are many examples where AI may help improve outcomes and possibly quality of life. “I can imagine a few ways language models can help with morbidity and mortality in the short-to-medium run. The first is in medical errors,” he said. “The second is in minimizing misdiagnosis and delayed diagnosis. We already have good experimental data that LLMs can make diagnoses better than humans and I’m running studies now showing how the use of LLMs actually changes human behavior,” said Dr. Rodman.
Patient Triage
Within the next several years, he envisions there will be human trials with AI providing a “second opinion.” AI is perfectly suited for triaging patients and patient navigation. Health systems have a vested interest in both triaging patients to the right place for safety, and navigating them to the right place for efficiency. These systems currently rely on a considerable amount of manpower.
Triage decisions are high stakes, so there would need to be a high bar for evidence to show that LLMs can perform in this domain, Dr Rodman said. “But that’s what is exciting. Even if imperfect, their ease of use and ability to be integrated into clinical workflows could theoretically make humans make better decisions.”
References
Rodman A, Buckley TA, Manrai AK, et al. Artificial intelligence vs clinician performance in estimating probabilities of diagnoses before and after testing. JAMA Netw Open, 2023;6 (12):e2347075 doi:10.1001/jamanetworkopen.2023.47075
Wang S, Rong R, Zhou Q, et al. Deep learning of cell spatial organizations identifies clinically relevant insights in tissue images. Nat Commun 14, 7872 (2023). doi:10.1038/s41467-023-43172-8
This article originally appeared on Renal and Urology News