The Role of Big Data Analytics in Insurance | by Jen Roberts | Mar, 2024
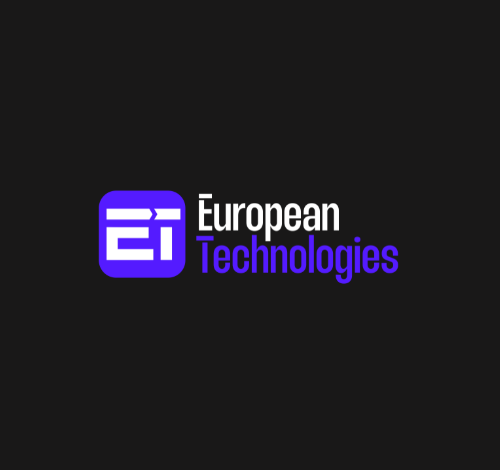
In the quickly changing world of risk analysis and management, the exponential growth of data and computing power has ushered in changes, bringing challenges to traditional actuarial foundations. Data science has emerged as a multidisciplinary field leveraging advanced statistics, machine learning, and enormous datasets to gain insights and find patterns hidden by today’s highly complex data environments.
With information accumulating at unprecedented speeds, time-tested actuarial techniques in isolation may fail to show the complete story on their own anymore. While mathematical and statistical rigor remain important, integrating data science principles ensures actuaries can better comprehend our data-rich world.
Both fields aim to anticipate outcomes and reduce uncertainty. By mutually learning from each other, they can build on strengths to manage evolving risks and help communities around the world.
However, caution is needed.
While data science helps us understand risks, we must acknowledge data alone does not fully portray reality. Various perspectives influence data collection and preparation. Features deemed relevant and proxies used to depict complex social issues inherently impact results. Moreover, machine learning opacity raises accountability concerns versus traditional models grounded in interpretable statistics.
As various data impacts multiply, responsible practices require us to consider different data viewpoints and algorithm transparency. Actuaries and data scientists hoping to improve techniques and further important work must elevate the appropriate discussion.
Data science opens new doors for analyzing larger, more varied information. While mathematical and statistical rigor must remain, integrating data science principles will let actuaries better understand today’s data-rich world.
Both fields aim to predict outcomes and minimize uncertainty.
The coming together of actuarial science and data science presents both opportunities and challenges. On one hand, data-driven methods offer the exciting potential of more accurate risk modeling and predictive power. By utilizing the wealth of data generated by our digital lives, data scientists may be able to discover intricate connections and subtle patterns that evaded traditional actuarial techniques.
However, this potential comes with serious ethical and philosophical questions. As we entrust increasingly sophisticated algorithms to assess risk, we must have full control and understanding of the assumptions, biases, and power structures that underlie these systems.
Firstly, the very notion of “data” itself is complex with many perspectives. The data fueling these models does not simply reflect reality but is shaped by the priorities, views, and blind spots of those who collect and process it. The risk models we build are inherently influenced by the data included or excluded, features deemed relevant, and proxies used to quantify complicated social issues.
Moreover, a lack of transparency in many machine learning models raises issues of accountability. While traditional actuarial models rooted in statistics offer some interpretability, the inner workings of complex neural networks often remain vague, making them similar to “black boxes.” This lack of transparency poses challenges for auditing, validating, and critically evaluating the decisions and risk assessments created by these systems.
As data and algorithms impact more lives, professionals must ensure system analyses consider diverse data perspectives and address algorithmic opacity concerns. With open discussion, the fields can capitalize on each other’s strengths to further important work, while building understanding and trustthrough responsive dialogue. Together, progress depends on both upholding rigorous methodology and respecting the humansexperience.
We must thoughtfully consider the ethical implications of data-driven risk assessment, especially in areas that can greatly impact people and communities. Using indirect variables and perpetuating past biases within training data have these risks:
- increase and worsen unequal treatment,
- further entrench existing power structures, and
- continue to marginalize vulnerable groups.
I hope you agree with me by now, we must take an interdisciplinary approach that looks beyond any single field. We need insights from diverse areas like ethics, social sciences, data studies, and science technology studies to build a nuanced understanding of the technical systems we create.
Actuarial science and data science do not need to be seen as opposing sides in a contest with a sole winner. Instead, they should engage in productive discussion, with each field challenging and enriching the other.
Actuaries, with their in-depth knowledge of risk theory and field experience, can offer valuable perspectives on the interpretability, validity and real-world effects of data models. Conversely, data scientists can contribute novel techniques and computational methods to enhance predictive power and scalability in actuarial models.
Ultimately, realizing the true potential of their alignment relies on cultivating a thoughtful, ethical practice that questions assumptions and power dynamics within our models and methodologies. We must stay attentive in questioning the narratives and worldviews encoded in our data and algorithms, striving to create systems that prioritize fairness, accountability and well-being for all people.
As we stand at the precipice of a data-driven revolution, it is imperative that we approach this frontier with a critical lens, one that acknowledges the complexities and contradictions inherent in our pursuit of understanding and managing risk. Only through a holistic, interdisciplinary approach can we harness the power of data science to create more equitable, accountable, and just systems of risk assessment and management.