The Role of Computer Vision in Cybersecurity Automation
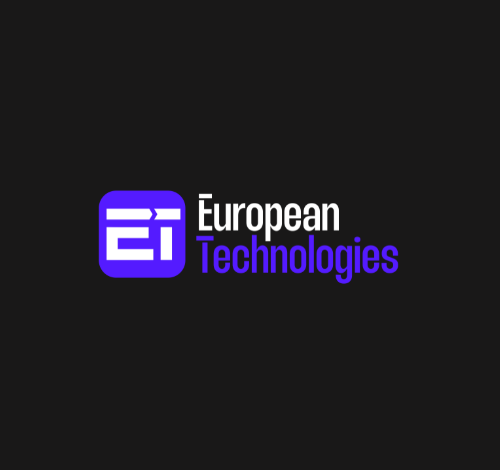
Summary
Computer vision is crucial in cybersecurity for enhancing threat detection through pattern recognition and anomaly identification.
Computer vision is crucial in cybersecurity for enhancing threat detection through pattern recognition and anomaly identification. Its ability to quickly and accurately analyze visual data empowers security systems to identify potential threats—like unusual user behavior or malicious software—that could compromise networks and sensitive information.
Automation is crucial in a landscape where cyberthreats continuously adapt and multiply. Organizations can swiftly detect and respond to risks by harnessing computer vision for automated monitoring. It significantly reduces their vulnerability to increasingly sophisticated attacks.
Computer vision in cybersecurity automation
Computer vision empowers cybersecurity systems to identify patterns and anomalies with remarkable precision, making it invaluable for threat detection. Analyzing visual data—like user activity, file structures and network traffic—can uncover unusual behaviors indicative of cyberattacks or suspicious software.
Organizations leveraging security AI and automation can save up to $1.76 million, thanks to the speed and accuracy with which these systems detect and neutralize threats. Automated threat detection with computer vision enables rapid, data-driven responses that minimize damage and prevent breaches. It provides significant protection in an increasingly digital world.
Applications in automated cybersecurity
Computer vision’s application in automated cybersecurity allows swift and accurate identification of threats across various domains. Here’s how it enhances detection, response and overall threat prevention.
Surveillance and physical security
Computer vision automates video surveillance by analyzing footage from security cameras to identify suspicious behavior in real time. It leverages advanced algorithms to detect unusual movements, patterns or objects. Data from security cameras makes up 40% of the world’s IoT-based data, so this vast visual information becomes a critical resource for pinpointing potential threats.
Automated systems quickly sift through hours of footage to recognize signs of trespassing, loitering or unauthorized access. They can also promptly generate alerts when security breaches occur to enable immediate response and minimize potential damage.
Biometric security systems
Facial recognition and other biometric systems automate user authentication with unmatched precision and speed. These systems can instantly verify identities by analyzing unique physical features, such as facial patterns, fingerprints and iris scans. This ensures that only authorized individuals gain access to sensitive information or secure locations.
This high accuracy and efficiency make computer vision crucial in preventing unauthorized access. It eliminates the vulnerabilities associated with traditional methods like passwords. Biometric authentication strengthens security protocols and streamlines access to provide a seamless, secure user experience.
Phishing detection
Algorithms identify phishing attempts in emails and websites by meticulously analyzing visual cues—like logos, branding and webpage layouts—that often mimic legitimate sources. Comparing these elements to known databases of authentic sites can spot inconsistencies that signal a phishing scam.
Phishing was the most effective cyberthreat in 2021—responsible for 90% of data breaches—making this automated detection critical. These intelligent systems flag suspicious links and messages to enable immediate response to potential attacks. They also reduce the risk of employees accidentally exposing sensitive information to malicious actors.
Document and file analysis
Computer vision techniques automate analyzing suspicious files and documents using sophisticated pattern recognition and data analysis. These algorithms examine document structures, embedded images and visual signatures to identify characteristics that may indicate malware or phishing attempts.
Classifying file types and recognizing potential threats like harmful attachments flags risky documents to prevent their download or execution. This automated classification streamlines threat detection, helps cybersecurity teams stay ahead of attackers and prevents malicious files from infiltrating corporate networks.
Anomaly detection and intrusion detection systems
Computer vision identifies unusual activities within network traffic or user behavior by translating data into visual patterns that algorithms can quickly analyze. Research has demonstrated that 2D visualizations of malicious and benign files can effectively train machine learning-based classifiers to detect malware in new entities.
These classifiers distinguish between normal and suspicious patterns by processing network traffic and user activity logs as visual representations. It helps uncover subtle indicators of potential threats. Moreover, image processing can highlight anomalies in network traffic, detect unusual log patterns and identify distinctive malware signatures. It ensures organizations flag emerging threats before causing significant damage.
Challenges and future directions
Computer vision systems face challenges like false positives and adversarial attacks due to their reliance on mathematical algorithms trained on specific datasets. Malicious actors can exploit these vulnerabilities by subtly altering an object’s appearance or manipulating an aspect of the computer vision software. This practice tricks the system into misclassifying legitimate activities as threats or vice versa.
This vulnerability creates a risk of costly false alarms or undetected breaches. However, integrating computer vision with other AI systems—like anomaly detection and behavior analysis—can create a multilayered security framework that minimizes these risks. This comprehensive approach leverages complementary AI techniques to bolster accuracy, making it more challenging for adversaries to exploit a single point of failure.
Advancing toward more accurate automated security
Thanks to advancements in computer vision and AI integration, the future of automated security holds the potential for increasingly refined and accurate protection. As cybersecurity technologies continue to evolve, organizations can anticipate systems that will better detect and neutralize sophisticated threats with unparalleled precision.
About The Author
Zac Amos is the features editor at ReHack, where he covers trending tech news in cybersecurity and artificial intelligence. For more of his work, follow him on Twitter or LinkedIn.
Did you enjoy this great article?
Check out our free e-newsletters to read more great articles..