The year machines will run out of data
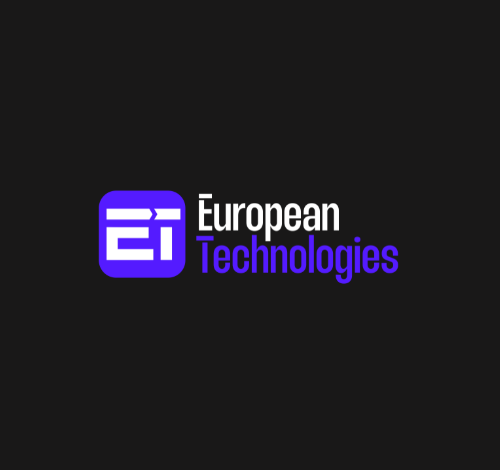
Hello Nature readers, would you like to get this Briefing in your inbox free every week? Sign up here.
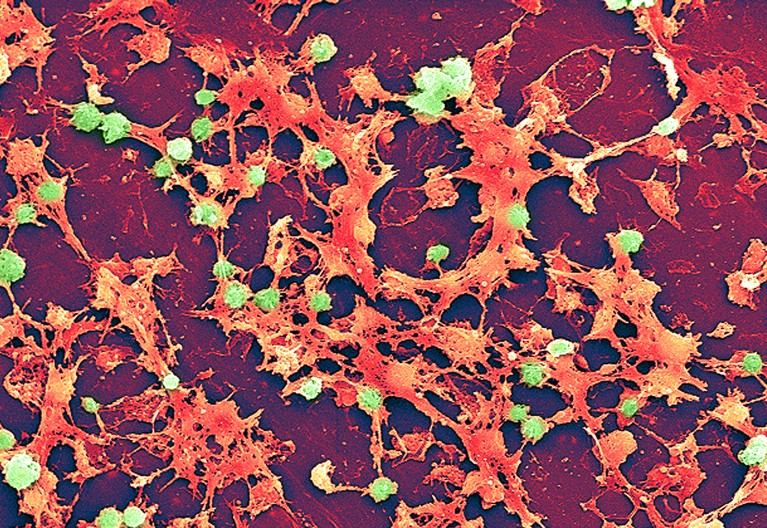
Staphylococcus aureus bacteria (green and orange; artificially coloured) were among those killed by one of the peptides identified by a machine-learning approach.Credit: Science Source/Science Photo Library
A machine-learning algorithm sifted through tens of thousands of microbial genomes and predicted more than 800,000 DNA snippets that encode for antimicrobial compounds. More than 90% of these potential new antibiotics had never been described before. Out of the 100 of these compounds the researchers synthesised, three were highly effective in mice against an infection of an often-resistant pathogen. “This indicates that their efficacy may be limited in vivo,” says biophysicist Seyed Majed Modaresi. “Still, this is a remarkable result, and the compounds might circumvent severe toxicity side effects of [other] last-resort antibiotics.”
DW | 4 min read & Nature Research Highlight | 3 min read (Nature paywall)
Microsoft’s AI model Aurora accurately predicts weather and, for the first time, air pollution for the whole world — and does so in less than a minute. Global air pollution forecasting is much more complex than weather forecasting, says machine-learning researcher Matthew Chantry: “That was the thing where I went: wow, that’s a really cool result.” The AI forecasts are as good as those from conventional mathematical models and have “orders of magnitude smaller computational cost”, say Microsoft researchers.
Reference: arXiv preprint (not peer reviewed)
AI systems could exhaust the supply of publicly available, human-crafted writing in the next two to eight years. So far, the amount of text data that large language models are trained on has more than doubled every year. “If you start hitting those constraints about how much data you have, then you can’t really scale up your models efficiently anymore,” says computing researcher and study co-author Tamay Besiroglu. Others are less worried about a bottleneck. “I think it’s important to keep in mind that we don’t necessarily need to train larger and larger models,” says computer engineer Nicolas Papernot.
Reference: arXiv preprint (not peer reviewed)
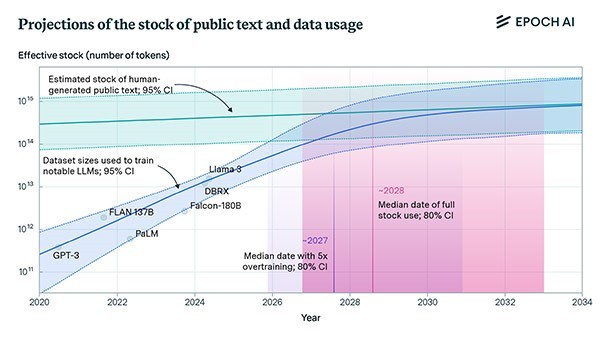
Sometime between 2026 and 2032, the amount of text needed to train the next big AI model (blue line) will approach the amount of all human-written, publicly available data (turquoise line). (P. Villalobos et al./arXiv preprint)
Features & opinion
Researchers are working towards 1-bit large language models (LLMs) that are cheaper, faster and less energy-intensive. In a 1-bit AI model, the complexity of its parameters is dramatically reduced so that information can be represented by -1 or 1 — 1 bit instead of 16, say. So far, starting with full-size models and paring them down has been more popular. But quantization-aware training, which starts from scratch with low-precision parameters, has the potential to do even better. One such model performed as well as a comparable full-precision model while consuming 94% less computing energy.
“The impact of generative AI on election misinformation might not yet be as extensive as feared,” write information researcher Kiran Garimella and political scientist Simon Chauchard. Ahead of India’s recent elections, they asked 500 people to donate their WhatsApp data. Among nearly 2,000 highly-shared messages, just 1% contained AI-created images — often futuristic infrastructure projects or projections of Hindu supremacy (the duo did not look at AI-generated text or audio). Despite this relatively low figure, it’s important to remain vigilant about AI-generated propaganda, they caution.
Computer chips are trying to keep pace with the ever-increasing computing demands of AI models. A key part of this has been the switch from central processing units (CPUs) to graphics processing units (GPUs), which can do the many parallel calculations needed for AI tasks much faster than can CPUs. As AI applications move into mobile devices, “I don’t think GPUs are enough any more”, says computer engineer Cristina Silvano. Engineers are starting to use various tricks, including more accessible memory and numerical shorthand, to push the speed barriers of conventional computing.
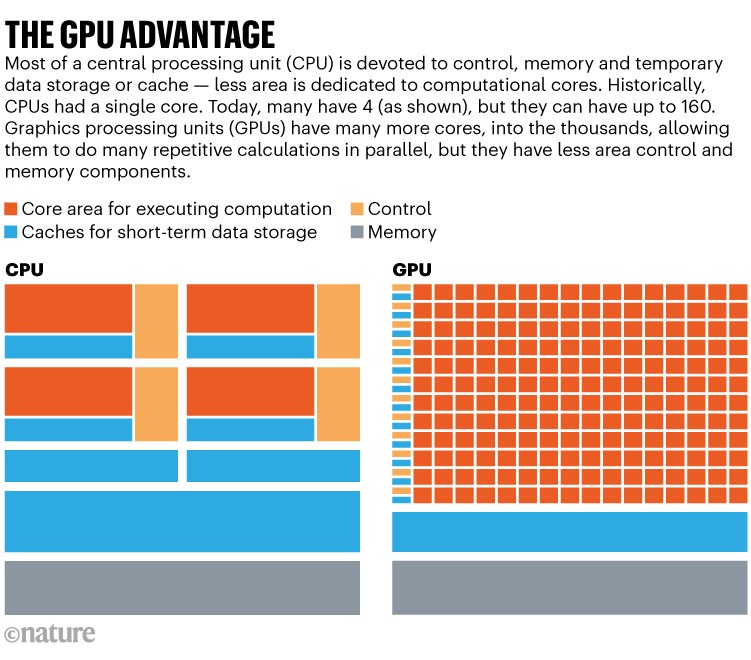
Source: Cornell University