Top 10 Challenges of Big Data Analytics in Healthcare
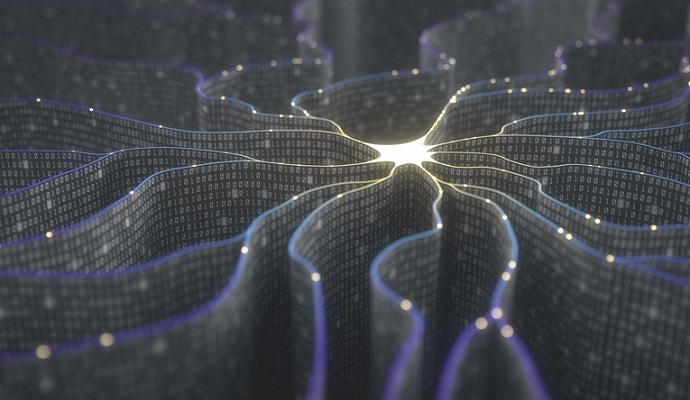
– Big data analytics is a major undertaking for the healthcare industry.
Providers who have barely come to grips with putting data into their electronic health records (EHRs) are now tasked with pulling actionable insights out of them – and applying those learnings to complicated initiatives that directly impact reimbursement.
For healthcare organizations that successfully integrate data-driven insights into their clinical and operational processes, the rewards can be huge. Healthier patients, lower care costs, more visibility into performance, and higher staff and consumer satisfaction rates are among the many benefits of turning data assets into data insights.
However, the road to meaningful healthcare analytics is a rocky one, filled with challenges and problems to solve.
Big data are complex and unwieldy, requiring healthcare organizations to take a close look at their approaches to collecting, storing, analyzing, and presenting their data to staff members, business partners, and patients.
READ MORE: The Healthcare Data Cycle: Generation, Collection, and Processing
What are some of the top challenges organizations typically face when booting up a big data analytics program, and how can they overcome these issues to achieve their data-driven clinical and financial goals?
1. CAPTURE
All data comes from somewhere. Unfortunately, for many healthcare providers, it doesn’t always come from somewhere with impeccable data governance habits. Capturing data that is clean, complete, accurate, and formatted correctly for use in multiple systems is an ongoing battle for organizations, many of which aren’t on the winning side of the conflict.
Having a robust data collection process is key to advancing big data analytics efforts in healthcare in the age of EHRs, artificial intelligence (AI), and machine learning (ML). Proper data capture is one of the first steps organizations can take to build datasets and support projects to improve clinical care.
Poor EHR usability, convoluted workflows, and an incomplete understanding of why big data are important to capture can all contribute to quality issues that will plague data throughout its lifecycle and limit its useability.
Providers can start to improve their data capture routines by prioritizing valuable data types – EHRs, genomic data, population-level information – for their specific projects, enlisting the data governance and integrity expertise of health information management professionals, and developing clinical documentation improvement programs that coach clinicians about how to ensure that data are useful for downstream analytics.
2. CLEANING
READ MORE: Storage, Management, and Analysis in the Health Data Lifecycle
Healthcare providers are intimately familiar with the importance of cleanliness in the clinic and the operating room, but may not be quite as aware of how vital it is to cleanse their data, too.
Dirty data can quickly derail a big data analytics project, especially when bringing together disparate data sources that may record clinical or operational elements in slightly different formats. Data cleaning – also known as cleansing or scrubbing – ensures that datasets are accurate, correct, consistent, relevant, and not corrupted.
The Office of the National Coordinator for Health Information Technology (ONC) recommends conducting data cleaning processes as close to the point of first capture as possible, as doing so minimizes potential duplications of effort or conflicting cleansing activities.
While some data cleaning processes are still performed manually, automated data cleaning tools and frameworks are available to assist healthcare stakeholders with their data integrity efforts. These tools are likely to become increasingly sophisticated and precise as AI and ML techniques continue their rapid advance, reducing the time and expense required to ensure high levels of accuracy and integrity in healthcare data warehouses.
3. STORAGE
Data storage is a critical cost, security, and performance issue for a healthcare information technology (IT) department. As the volume of healthcare data grows exponentially, some providers are no longer able to manage the costs and impacts of on-premise data centers.
READ MORE: Visualizing, Interpreting, and Disposing of Healthcare Analytics Data
On-premise data storage promises control over security, access, and up-time, but an on-site server network can be expensive to scale, difficult to maintain, and prone to producing data silos across different departments.
Cloud storage and other digital health ecosystems are becoming increasingly attractive for providers and payers as costs drop and reliability grows.
The cloud offers nimble disaster recovery, lower up-front costs, and easier expansion – although organizations must be extremely careful about choosing Health Insurance Portability and Accountability Act of 1996 (HIPAA)-compliant cloud storage partners.
Many organizations end up with a hybrid approach to their data storage programs, which may be the most flexible and workable approach for providers with varying data access and storage needs. When developing hybrid infrastructure, however, providers should be careful to ensure that disparate systems are able to communicate and share data with other segments of the organization when necessary.
4. SECURITY
Data security is a major priority for healthcare organizations, especially in the wake of a rapid-fire series of high-profile breaches, hackings, and ransomware episodes. From zero-day attacks to AI-assisted cyberattacks, healthcare data are subject to a nearly infinite array of vulnerabilities.
The HIPAA Security Rule includes a long list of technical safeguards for organizations storing protected health information (PHI), including transmission security, authentication protocols, and controls over access, integrity, and auditing.
In practice, these safeguards translate into common-sense security procedures such as using up-to-date anti-virus software, encrypting sensitive data, and using multi-factor authentication.
But even the most tightly secured data center can be taken down by the fallibility of human staff members, who may not be well-versed in good cybersecurity practices.
Healthcare organizations must frequently communicate the critical nature of data security protocols across the enterprise, prioritize employee cybersecurity training and healthcare-specific cybersecurity performance goals, and consistently review who has access to high-value data assets to prevent malicious parties from causing damage.
5. STEWARDSHIP
Healthcare data, especially on the clinical side, has a long shelf life. In addition to keeping patient data accessible for at least six years as required by HIPAA, providers may wish to utilize de-identified datasets for research projects, which makes ongoing stewardship and curation an important concern. Data may also be reused or reexamined for other purposes, such as quality measurement or performance benchmarking.
Understanding when, by whom, and for what purpose the data were created – as well as how those data were used in the past – is important for researchers and data analysts.
Developing complete, accurate, and updated metadata is a key component of a successful data governance plan. Metadata allows analysts to exactly replicate previous queries, which is vital for scientific studies and accurate benchmarking, and prevents the creation of “data dumpsters,” or isolated datasets with limited utility.
Healthcare organizations should assign a data steward to handle the development and curation of meaningful metadata. A data steward can ensure that all elements have standard definitions and formats, are documented appropriately from creation to deletion, and remain useful for the tasks at hand.
6. QUERYING
Robust metadata and strong stewardship protocols also make it easier for organizations to query their data and get the answers that they seek. The ability to query data is foundational for reporting and analytics, but healthcare organizations must typically overcome a number of challenges before they can engage in meaningful analysis of their big data assets.
Firstly, they must overcome data silos and interoperability problems that prevent query tools from accessing the organization’s entire repository of information. If different components of a dataset exist in multiple walled-off systems or in different formats, it may not be possible to generate a complete portrait of an organization’s status or an individual patient’s health.
Even if data live in a common warehouse, standardization and quality can be lacking. In the absence of medical coding systems like the International Classification of Diseases (ICD), SNOMED-CT, or Logical Observation Identifiers Names and Codes (LOINC) that reduce free-form concepts into a shared ontology, it may be difficult to ensure that a query is identifying and returning the correct information to the user.
Many organizations use Structured Query Language (SQL) to dive into large datasets and relational databases, but it is only effective when a user can first trust the accuracy, completeness, and standardization of the data at hand.
7. REPORTING
After providers have nailed down the query process, they must generate a report that is clear, concise, and accessible to the target audience.
Once again, the accuracy and integrity of the data has a critical downstream impact on the accuracy and reliability of the report. Poor data at the outset will produce suspect reports at the end of the process, which can be detrimental for clinicians who are trying to use the information to treat patients.
Providers must also understand the difference between “analysis” and “reporting.” Reporting is often the prerequisite for analysis – the data must be extracted before it can be examined – but reporting can also stand on its own as an end product.
While some reports may be geared toward highlighting a certain trend, coming to a novel conclusion, or convincing the reader to take a specific action, others must be presented in a way that allows the reader to draw their own inferences about what the full spectrum of data means.
Organizations should be very clear about how they plan to use their reports to ensure that database administrators can generate the information they actually need.
A great deal of the reporting in the healthcare industry is external, since regulatory and quality assessment programs frequently demand large volumes of data to feed quality measures and reimbursement models. Providers have a number of options for meeting these various requirements, including qualified registries, reporting tools built into their electronic health records, and web portals hosted by the Centers for Medicare & Medicaid Services (CMS) and other groups.
8. VISUALIZATION
At the point of care, clean and engaging data visualization can make it much easier for a clinician to absorb information and use it appropriately.
Color-coding is a popular data visualization technique that typically produces an immediate response – for example, red, yellow, and green are generally understood to mean stop, caution, and go.
Organizations must also consider data presentation best practices, such as leveraging charts that use proper proportions to illustrate contrasting figures and correct labeling of information to reduce potential confusion. Convoluted flowcharts, cramped or overlapping text, and low-quality graphics can frustrate and annoy recipients, leading them to ignore or misinterpret data.
Common healthcare data visualization approaches include pivot tables, charts, and dashboards, all of which have their own specific uses to illustrate concepts and information.
9. UPDATING
Healthcare data are dynamic, and most elements will require relatively frequent updates in order to remain current and relevant. For some datasets, like patient vital signs, these updates may occur every few seconds. Other information, such as home address or marital status, might only change a few times during an individual’s entire lifetime.
Understanding the volatility of big data, or how often and to what degree it changes, can be a challenge for organizations that do not consistently monitor their data assets.
Providers must have a clear idea of which datasets need manual updating, which can be automated, how to complete this process without downtime for end-users, and how to ensure that updates can be conducted without damaging the quality or integrity of the dataset.
Organizations should also ensure that they are not creating unnecessary duplicate records when attempting an update to a single element, which may make it difficult for clinicians to access necessary information for patient decision-making.
10. SHARING
Providers don’t operate in a vacuum, and few patients receive all of their care at a single location. This means that sharing data with external partners is essential, especially as the industry moves toward population health management and value-based care.
Data interoperability is a perennial concern for organizations of all types, sizes, and positions along the data maturity spectrum.
Fundamental differences in the design and implementation of health information systems can severely curtail a user’s ability to move data between disparate organizations, often leaving clinicians without information they need to make key decisions, follow up with patients, and develop strategies to improve overall outcomes.
The industry is currently working hard to improve the sharing of data across technical and organizational barriers. Emerging tools and strategies such as the Fast Healthcare Interoperability Resource (FHIR) and application programming interfaces (APIs) are making it easier for organizations to share data easily and securely.
But adoption of these methodologies varies, leaving many organizations cut off from the possibilities inherent in the seamless sharing of patient data.
In order to develop a big data exchange ecosystem that connects all members of the care continuum with trustworthy, timely, and meaningful information, providers will need to overcome every challenge on this list. Doing so will take time, commitment, funding, and communication – but success will ease the burdens of all those concerns.