Understanding the GenAI ‘Stack’ – Publications
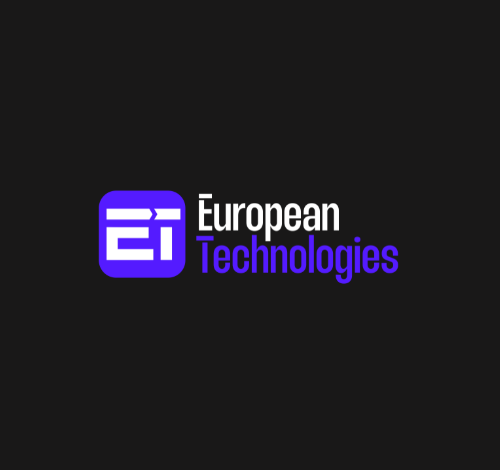
Insight
June 12, 2024
The rapid rise of generative artificial intelligence (GenAI) is poised to revolutionize industries and potentially unleash efficiencies and innovation in the global economy. However, this transformative technology has also garnered significant antitrust scrutiny from commentators and regulators. As GenAI becomes increasingly integrated into sectors such as healthcare, automotive, and finance, among other digital services, competition authorities in the United States (US), United Kingdom (UK), and European Union (EU) are considering each layer of the GenAI stack to help sustain a competitive and innovative marketplace.
The GenAI Stack: A Complex Ecosystem
Many enforcers and industry stakeholders view the structure of the GenAI sector through the computing technology concept known as a “stack.” The GenAI stack, or value chain, comprises several layers and building blocks, each presenting unique antitrust and competition challenges.
While there is no single agreed-upon definition of the GenAI “stack,” the stack generally contains the following core layers:
- Infrastructure Layer. The infrastructure layer consists of the hardware (specialized silicon chips) and cloud services necessary for training and hosting GenAI models. These resources provide the computational power for both model training (building from scratch) and inference (using the model in production).
- Model Layer. The model layer encompasses a spectrum of models, from closed (proprietary, typically accessed via an API) to open (internally accessible to varying degrees). Closed models offer lower development costs but less flexibility, while open models provide greater flexibility but demand higher development costs and technical expertise. Beyond general foundation models, this layer also includes fine-tuned or augmented models tailored for specific tasks or domains.
- Application Layer. The application layer consists of two general deployment options: (1) point solutions built on top of a foundation or fine-tuned model and (2) end-to-end applications where a developer builds a proprietary model from scratch specifically for its own end-user-facing application.
The stack also encompasses three additional building blocks for development:
- Data. Some enforcers have noted that data is the lifeblood of the GenAI stack, providing the raw material that fuels AI models and applications. The data layer is particularly critical, as the quality and quantity of data directly impact the performance and capabilities of GenAI models and services.
- FMOps/Tooling. FMOps, or Foundation Model Operations, encompass the end-to-end lifecycle management of foundation models, from data preparation and model training to deployment, monitoring, and continuous improvement. The tooling layer complements FMOps by providing various software, platforms, and frameworks to support AI development, deployment, and management. FMOps and the tooling layer are interconnected, but while FMOps emphasize the operational aspects of managing large-scale foundation models, the tooling layer provides a broader set of tools that support the development and deployment of AI models more generally.
- Talent. Because this technology is complex and requires a specialized skillset to go from concept to reality, talent and expertise play a pivotal role in the development and deployment of GenAI.
Balancing Innovation with Concerns from Global Competition Authorities
While competition enforcers in the US, UK, and EU deploy differing legal frameworks, they share largely the same substantive concerns across the GenAI landscape. Despite these concerns, regulators also recognize we are in the early stages of a potentially transformative technology and are mindful of the need and benefit of balancing enforcement against fostering innovation and open markets.
The high-level categories of potential competition concerns and countervailing forces include:
- Access. Enforcers have highlighted nondiscriminatory access to data, compute, models, and talent as areas of focus. While data are valuable, they are often not the limiting factor in GenAI development. Moreover, open-source datasets, data-sharing initiatives, the development of smaller models, and the increasing availability of computational resources all work to maintain a relatively level playing field. Additionally, competitive compensation and collaborative environments can effectively attract and nurture the talent necessary for development.
- Conditional Dealing. Enforcers have also raised concerns about various forms of conditional dealing, including exclusive dealing arrangements, bundling and tying practices, and rebates or loyalty discounts. While these practices can sometimes lead to market foreclosure or limit user choice, they can also serve legitimate business purposes, such as incentivizing investment in new technologies, reducing transaction costs, driving product adoption, and offering cost savings to customers.
- Interoperability and Routes to Market. Enforcers will likely encourage open routes to market and interoperability across the GenAI stack. Interoperability is widespread today as companies recognize the value in attracting enterprise clients that require seamless integration between different tools and services. However, it is important to note that defaults and predefined routes to market can also benefit users by reducing transaction costs and enhancing user experience. Users often prefer the convenience of preconfigured options, especially for complex products.
- Open Early, Closed Late. Enforcers are wary of “open early, closed late” strategies, where technology companies initially offer open access to their technology to attract a broad user base only to later restrict access, potentially locking in customers. However, this process is often a natural part of the complex technological lifecycle. Open platforms provide fertile ground for companies to build proprietary systems and features on top of an open-source foundation. The proprietary functionality allows innovators to capture value from their work and incentivizes investment, ultimately fueling the open-source project and further innovative development.
- Transactions and Board Interlocks. Regulators are closely examining transactions and board interlocks in the AI sector. Deal structures of interest include partnerships, traditional horizontal acquisitions, overlapping data acquisitions and licensing, and complementary acquisitions between players across the stack. Given the current stage in the industry lifecycle, consolidation in the AI space is likely. However, such consolidation stands to boost, rather than harm, competition and innovation. Many promising AI companies lack the resources, expertise, and infrastructure to bring their ideas to fruition, and acquisitions by larger players often provide early-stage firms with the financial backing, technical knowledge, and access to markets critical for their survival and continued development. This cross-pollination of ideas can lead to breakthroughs that are unlikely to occur if such companies remain independent. Moreover, mergers of complements, where companies combine different but functionally linked parts of the AI value chain, can often be procompetitive, leading to efficiencies, improved product offerings, and accelerated innovation.
Regulators face the delicate task of balancing the need to protect competition with the desire to foster innovation in the rapidly evolving GenAI landscape. This assessment involves carefully considering the potential benefits and drawbacks of different business practices and tailoring enforcement actions accordingly. By adopting a nuanced and balanced approach, enforcers can create a regulatory environment that encourages healthy competition, protects consumer interests, and allows GenAI to flourish as a transformative technology.
Best Practices for Compliance
When traversing the complex GenAI landscape, companies should develop risk mitigation strategies to avoid the concerns identified by global competition authorities. The appropriate measures require consideration of the legal regimes in different jurisdictions and a careful balancing of the pros and cons of different approaches:
- Data Collection and Management. Catalog origins of training data and ensure the collection and use of data meets governing standards (e.g., transparency requirements).
- Strive for Nondiscriminatory Access to Inputs. Monitor exclusivity requirements with respect to data, infrastructure, and models and provide access on nondiscriminatory terms when possible. Where access is limited, document the procompetitive rationale for such limitations, such as user preferences or superior product design.
- Conditional Dealing Flexibility and Documentation. When engaging in bundling practices, consider whether customers should be offered the option to substitute functionality to an alternative service. Regardless of the ability to substitute, document the benefits and procompetitive effects of its promotion practices as well as any customer preferences for the offering.
- Promote Interoperability and Choice. To the extent possible and beneficial to users, adopt interoperability throughout the stack where point solutions can compete with functionality in a suite of offerings. Where GenAI functionality is integrated within an existing product, consider ways to allow users to choose and/or switch between competing solutions. But where such a product design is technically burdensome or suboptimal from a user experience perspective, clearly document such difficulties and/or user preferences for the superior design.
- Develop Antitrust Compliance Program for Board Activity. To reduce risks associated with outside board members, develop antitrust compliance programs that monitor board appointments and implement processes for information sharing and competitive decision-making.
- Commitment to Maintain Terms Post-Transaction. To the extent feasible, firms entering into transactions in the AI space should document commitments to maintain current interoperability and access terms post-transaction. With respect to data-driven transactions and complementary transactions across the stack, documentation of the combined firm’s intent to continue dealing on the same terms post-transaction may mitigate risk associated with foreclosure concerns.
The US, UK, and EU are all closely scrutinizing the GenAI landscape. By understanding the antitrust and competition concerns across the GenAI stack and adhering to best practices, companies can foster innovation in AI development and use while ensuring compliance with global regulatory frameworks. Proactive engagement with legal counsel and competition authorities, along with transparent business practices, will be key to thriving in a complex regulatory landscape.