Videos: Robots With Knives, Powerline Drones, Exoskeletons
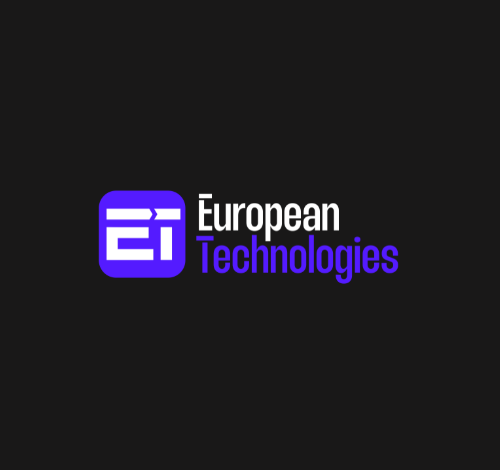
Greetings from the
IEEE International Conference on Robotics and Automation (ICRA) in Yokohama, Japan! We hope you’ve been enjoying our short videos on TikTok, YouTube, and Instagram. They are just a preview of our in-depth ICRA coverage, and over the next several weeks we’ll have lots of articles and videos for you. In today’s edition of Video Friday, we bring you a dozen of the most interesting projects presented at the conference.
Enjoy today’s videos, and stay tuned for more ICRA posts!
Upcoming robotics events for the next few months:
RoboCup 2024: 17–22 July 2024, EINDHOVEN, NETHERLANDS
ICSR 2024: 23–26 October 2024, ODENSE, DENMARK
Cybathlon 2024: 25–27 October 2024, ZURICH, SWITZERLAND
Please
send us your events for inclusion.
The following two videos are part of the “
Cooking Robotics: Perception and Motion Planning” workshop, which explored “the new frontiers of ‘robots in cooking,’ addressing various scientific research questions, including hardware considerations, key challenges in multimodal perception, motion planning and control, experimental methodologies, and benchmarking approaches.” The workshop featured robots handling food items like cookies, burgers, and cereal, and the two robots seen in the videos below used knives to slice cucumbers and cakes. You can watch all workshop videos here.
“SliceIt!: Simulation-Based Reinforcement Learning for Compliant Robotic Food Slicing,” by Cristian C. Beltran-Hernandez, Nicolas Erbetti, and Masashi Hamaya from OMRON SINIC X Corporation, Tokyo, Japan.
Cooking robots can enhance the home experience by reducing the burden of daily chores. However, these robots must perform their tasks dexterously and safely in shared human environments, especially when handling dangerous tools such as kitchen knives. This study focuses on enabling a robot to autonomously and safely learn food-cutting tasks. More specifically, our goal is to enable a collaborative robot or industrial robot arm to perform food-slicing tasks by adapting to varying material properties using compliance control. Our approach involves using Reinforcement Learning (RL) to train a robot to compliantly manipulate a knife, by reducing the contact forces exerted by the food items and by the cutting board. However, training the robot in the real world can be inefficient, and dangerous, and result in a lot of food waste. Therefore, we proposed SliceIt!, a framework for safely and efficiently learning robot food-slicing tasks in simulation. Following a real2sim2real approach, our framework consists of collecting a few real food slicing data, calibrating our dual simulation environment (a high-fidelity cutting simulator and a robotic simulator), learning compliant control policies on the calibrated simulation environment, and finally, deploying the policies on the real robot.
“Cafe Robot: Integrated AI Skillset Based on Large Language Models,” by Jad Tarifi, Nima Asgharbeygi, Shuhei Takamatsu, and Masataka Goto from Integral AI in Tokyo, Japan, and Mountain View, Calif., USA.
The cafe robot engages in natural language inter-action to receive orders and subsequently prepares coffee and cakes. Each action involved in making these items is executed using AI skills developed by Integral, including Integral Liquid Pouring, Integral Powder Scooping, and Integral Cutting. The dialogue for making coffee, as well as the coordination of each action based on the dialogue, is facilitated by the Integral Task Planner.
“Autonomous Overhead Powerline Recharging for Uninterrupted Drone Operations,” by Viet Duong Hoang, Frederik Falk Nyboe, Nicolaj Haarhøj Malle, and Emad Ebeid from University of Southern Denmark, Odense, Denmark.
We present a fully autonomous self-recharging drone system capable of long-duration sustained operations near powerlines. The drone is equipped with a robust onboard perception and navigation system that enables it to locate powerlines and approach them for landing. A passively actuated gripping mechanism grasps the powerline cable during landing after which a control circuit regulates the magnetic field inside a split-core current transformer to provide sufficient holding force as well as battery recharging. The system is evaluated in an active outdoor three-phase powerline environment. We demonstrate multiple contiguous hours of fully autonomous uninterrupted drone operations composed of several cycles of flying, landing, recharging, and takeoff, validating the capability of extended, essentially unlimited, operational endurance.
“Learning Quadrupedal Locomotion With Impaired Joints Using Random Joint Masking,” by Mincheol Kim, Ukcheol Shin, and Jung-Yup Kim from Seoul National University of Science and Technology, Seoul, South Korea, and Robotics Institute, Carnegie Mellon University, Pittsburgh, Pa., USA.
Quadrupedal robots have played a crucial role in various environments, from structured environments to complex harsh terrains, thanks to their agile locomotion ability. However, these robots can easily lose their locomotion functionality if damaged by external accidents or internal malfunctions. In this paper, we propose a novel deep reinforcement learning framework to enable a quadrupedal robot to walk with impaired joints. The proposed framework consists of three components: 1) a random joint masking strategy for simulating impaired joint scenarios, 2) a joint state estimator to predict an implicit status of current joint condition based on past observation history, and 3) progressive curriculum learning to allow a single network to conduct both normal gait and various joint-impaired gaits. We verify that our framework enables the Unitree’s Go1 robot to walk under various impaired joint conditions in real world indoor and outdoor environments.
“Synthesizing Robust Walking Gaits via Discrete-Time Barrier Functions With Application to Multi-Contact Exoskeleton Locomotion,” by Maegan Tucker, Kejun Li, and Aaron D. Ames from Georgia Institute of Technology, Atlanta, Ga., and California Institute of Technology, Pasadena, Calif., USA.
Successfully achieving bipedal locomotion remains challenging due to real-world factors such as model uncertainty, random disturbances, and imperfect state estimation. In this work, we propose a novel metric for locomotive robustness – the estimated size of the hybrid forward invariant set associated with the step-to-step dynamics. Here, the forward invariant set can be loosely interpreted as the region of attraction for the discrete-time dynamics. We illustrate the use of this metric towards synthesizing nominal walking gaits using a simulation in-the-loop learning approach. Further, we leverage discrete time barrier functions and a sampling-based approach to approximate sets that are maximally forward invariant. Lastly, we experimentally demonstrate that this approach results in successful locomotion for both flat-foot walking and multicontact walking on the Atalante lower-body exoskeleton.
“Supernumerary Robotic Limbs to Support Post-Fall Recoveries for Astronauts,” by Erik Ballesteros, Sang-Yoep Lee, Kalind C. Carpenter, and H. Harry Asada from MIT, Cambridge, Mass., USA, and Jet Propulsion Laboratory, California Institute of Technology, Pasadena, Calif., USA.
This paper proposes the utilization of Supernumerary Robotic Limbs (SuperLimbs) for augmenting astronauts during an Extra-Vehicular Activity (EVA) in a partial-gravity environment. We investigate the effectiveness of SuperLimbs in assisting astronauts to their feet following a fall. Based on preliminary observations from a pilot human study, we categorized post-fall recoveries into a sequence of statically stable poses called “waypoints”. The paths between the waypoints can be modeled with a simplified kinetic motion applied about a specific point on the body. Following the characterization of post-fall recoveries, we designed a task-space impedance control with high damping and low stiffness, where the SuperLimbs provide an astronaut with assistance in post-fall recovery while keeping the human in-the-loop scheme. In order to validate this control scheme, a full-scale wearable analog space suit was constructed and tested with a SuperLimbs prototype. Results from the experimentation found that without assistance, astronauts would impulsively exert themselves to perform a post-fall recovery, which resulted in high energy consumption and instabilities maintaining an upright posture, concurring with prior NASA studies. When the SuperLimbs provided assistance, the astronaut’s energy consumption and deviation in their tracking as they performed a post-fall recovery was reduced considerably.
“ArrayBot: Reinforcement Learning for Generalizable Distributed Manipulation through Touch,” by Zhengrong Xue, Han Zhang, Jingwen Cheng, Zhengmao He, Yuanchen Ju, Changyi Lin, Gu Zhang, and Huazhe Xu from Tsinghua Embodied AI Lab, IIIS, Tsinghua University; Shanghai Qi Zhi Institute; Shanghai AI Lab; and Shanghai Jiao Tong University, Shanghai, China.
We present ArrayBot, a distributed manipulation system consisting of a 16 × 16 array of vertically sliding pillars integrated with tactile sensors. Functionally, ArrayBot is designed to simultaneously support, perceive, and manipulate the tabletop objects. Towards generalizable distributed manipulation, we leverage reinforcement learning (RL) algorithms for the automatic discovery of control policies. In the face of the massively redundant actions, we propose to reshape the action space by considering the spatially local action patch and the low-frequency actions in the frequency domain. With this reshaped action space, we train RL agents that can relocate diverse objects through tactile observations only. Intriguingly, we find that the discovered policy can not only generalize to unseen object shapes in the simulator but also have the ability to transfer to the physical robot without any sim-to-real fine tuning. Leveraging the deployed policy, we derive more real world manipulation skills on ArrayBot to further illustrate the distinctive merits of our proposed system.
“SKT-Hang: Hanging Everyday Objects via Object-Agnostic Semantic Keypoint Trajectory Generation,” by Chia-Liang Kuo, Yu-Wei Chao, and Yi-Ting Chen from National Yang Ming Chiao Tung University, in Taipei and Hsinchu, Taiwan, and NVIDIA.
We study the problem of hanging a wide range of grasped objects on diverse supporting items. Hanging objects is a ubiquitous task that is encountered in numerous aspects of our everyday lives. However, both the objects and supporting items can exhibit substantial variations in their shapes and structures, bringing two challenging issues: (1) determining the task-relevant geometric structures across different objects and supporting items, and (2) identifying a robust action sequence to accommodate the shape variations of supporting items. To this end, we propose Semantic Keypoint Trajectory (SKT), an object agnostic representation that is highly versatile and applicable to various everyday objects. We also propose Shape-conditioned Trajectory Deformation Network (SCTDN), a model that learns to generate SKT by deforming a template trajectory based on the task-relevant geometric structure features of the supporting items. We conduct extensive experiments and demonstrate substantial improvements in our framework over existing robot hanging methods in the success rate and inference time. Finally, our simulation-trained framework shows promising hanging results in the real world.
“TEXterity: Tactile Extrinsic deXterity,” by Antonia Bronars, Sangwoon Kim, Parag Patre, and Alberto Rodriguez from MIT and Magna International Inc.
We introduce a novel approach that combines tactile estimation and control for in-hand object manipulation. By integrating measurements from robot kinematics and an image based tactile sensor, our framework estimates and tracks object pose while simultaneously generating motion plans in a receding horizon fashion to control the pose of a grasped object. This approach consists of a discrete pose estimator that tracks the most likely sequence of object poses in a coarsely discretized grid, and a continuous pose estimator-controller to refine the pose estimate and accurately manipulate the pose of the grasped object. Our method is tested on diverse objects and configurations, achieving desired manipulation objectives and outperforming single-shot methods in estimation accuracy. The proposed approach holds potential for tasks requiring precise manipulation and limited intrinsic in-hand dexterity under visual occlusion, laying the foundation for closed loop behavior in applications such as regrasping, insertion, and tool use.
“Out of Sight, Still in Mind: Reasoning and Planning about Unobserved Objects With Video Tracking Enabled Memory Models,” by Yixuan Huang, Jialin Yuan, Chanho Kim, Pupul Pradhan, Bryan Chen, Li Fuxin, and Tucker Hermans from University of Utah, Salt Lake City, Utah, Oregon State University, Corvallis, Ore., and NVIDIA, Seattle, Wash., USA.
Robots need to have a memory of previously observed, but currently occluded objects to work reliably in realistic environments. We investigate the problem of encoding object-oriented memory into a multi-object manipulation reasoning and planning framework. We propose DOOM and LOOM, which leverage transformer relational dynamics to encode the history of trajectories given partial-view point clouds and an object discovery and tracking engine. Our approaches can perform multiple challenging tasks including reasoning with occluded objects, novel objects appearance, and object reappearance. Throughout our extensive simulation and real world experiments, we find that our approaches perform well in terms of different numbers of objects and different numbers
“Open Sourse Underwater Robot: Easys,” by Michikuni Eguchi, Koki Kato, Tatsuya Oshima, and Shunya Hara from University of Tsukuba and Osaka University, Japan.
“Sensorized Soft Skin for Dexterous Robotic Hands,” by Jana Egli, Benedek Forrai, Thomas Buchner, Jiangtao Su, Xiaodong Chen, and Robert K. Katzschmann from ETH Zurich, Switzerland, and Nanyang Technological University, Singapore.
Conventional industrial robots often use two fingered grippers or suction cups to manipulate objects or interact with the world. Because of their simplified design, they are unable to reproduce the dexterity of human hands when manipulating a wide range of objects. While the control of humanoid hands evolved greatly, hardware platforms still lack capabilities, particularly in tactile sensing and providing soft contact surfaces. In this work, we present a method that equips the skeleton of a tendon-driven humanoid hand with a soft and sensorized tactile skin. Multi-material 3D printing allows us to iteratively approach a cast skin design which preserves the robot’s dexterity in terms of range of motion and speed. We demonstrate that a soft skin enables frmer grasps and piezoresistive sensor integration enhances the hand’s tactile sensing capabilities.
From Your Site Articles
Related Articles Around the Web