Voice-Enabled Robot Collaboration in Quality Inspection
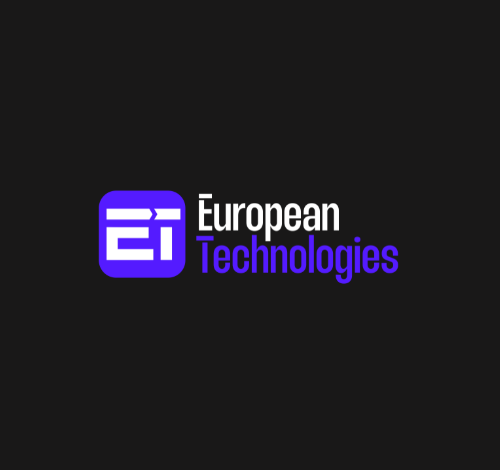
In a recent article published in the journal Applied Sciences, researchers introduced a novel framework facilitating human and robot collaboration via voice commands for quality inspection tasks. This framework, built on robot operating system version 2 (ROS2) architecture, seamlessly integrates speech recognition and computer vision modules. Additionally, the research validated the effectiveness of this method through a case study in the automotive industry, while also exploring its associated benefits and challenges.
Background
Quality inspection stands as a crucial process in modern manufacturing, as it ensures the reliability and customization of products. However, current inspection practices rely heavily on manual expertise, leading to time-consuming and error-prone procedures. Therefore, there is a need for solutions that enhance human-robot collaboration (HRC), enabling operators to interact with robots naturally and intuitively. Voice-based interaction emerges as a promising technique for facilitating HRC and empowering operators to use verbal commands to control robots and receive feedback seamlessly.
About the Research
In this paper, the authors developed a voice-enabled ROS2 framework specifically designed to address limitations in existing HRC systems for quality inspection tasks. The proposed framework aims to bridge the gap by offering a modular and flexible solution for inspecting parts in HRC environments. ROS2 is an open-source platform that provides the foundation for seamless communication and coordination between different software components within the framework. The main components of the framework include:
- Speech/voice recognition module: This component enables operators to communicate with the robot using voice commands such as start, stop, right, left, top, front, and back. It utilizes the Google Cloud Speech application programming interface (API) to convert speech signals into text and matches them with predefined commands. Additionally, it provides online filtering and feedback to the operator.
- Quality inspection module: This element employs OpenCV and TensorFlow for vision-based detection and classification of parts using an industrial camera and a deep learning model. It utilizes you only look once version 4 (YOLOv4) model, which is capable of localizing and classifying multiple objects in an image. Additionally, it provides confidence scores and bounding boxes for each detected part.
- Robot manipulation module: This module plans and executes complex actions of the robotic manipulator, such as moving the camera to different positions and orientations, using the ROS2 framework. It is divided into planning and movement sub-modules responsible for generating and executing motion commands, respectively.
- Visualization module: This component displays information and results, including inspection outcomes, voice commands, and workstation layout, through a graphical user interface (GUI). It assists operators in monitoring and reviewing the inspection process and its outcomes.
Furthermore, the proposed framework leverages the data distribution service (DDS) standard, allowing the configuration of quality-of-service parameters and connectivity beyond TCP protocols. Additionally, it utilizes web sockets and communication backends to enable the integration of different modules.
Research Findings
The study evaluated the performance and usability of the new framework in a case study derived from the automotive industry, focusing on the inspection of a car door panel by a robot arm and a human operator. The operator used voice commands to instruct the robot arm to move to different positions, capture images of the panel, and perform quality inspection tasks. The robot arm responded to the commands, executed the actions, and reported the results using speech synthesis.
The authors measured the accuracy of the speech recognition application, the quality inspection solution, and the overall framework. The outcomes demonstrated the new technique’s high performance, with 97.5% accuracy in speech recognition, 98.6% in object detection, and 95.8% in defect detection. Furthermore, it significantly decreased the cycle time for quality inspection by 37.5% compared to manual methods.
Applications
The framework can be applied to diverse industrial scenarios requiring quality inspection of parts within HRC environments. It offers support to operators and enhances inspection performance by delivering a robust and flexible solution integrating vision-based detection, voice recognition, robot manipulation, and visualization.
Moreover, it can be customized and expanded to address varying parts, defects, and inspection tasks by adjusting module parameters and models. Integration with other HRC frameworks and modules, such as augmented reality, smart devices, and cloud computing, can enable the provision of advanced and comprehensive solutions for HRC quality inspection.
Conclusion
In summary, the novel HRC framework proved effective for quality inspection, facilitating operator interaction with the robot via voice commands and real-time monitoring of inspection results. Moving forward, researchers acknowledged limitations and proposed future directions. They suggested improving the robustness and reliability of the voice recognition module by employing advanced speech recognition models and techniques, addressing noise and accent issues. Furthermore, they recommended developing a more interactive and immersive visualization module with a user-friendly graphical interface and incorporating additional feedback modalities such as sound and haptics.
Journal Reference
Papavasileiou, A.; Nikoladakis, S.; Basamakis, F.P.; Aivaliotis, S.; Michalos, G.; Makris, S. A Voice-Enabled ROS2 Framework for Human–Robot Collaborative Inspection. Appl. Sci. 2024, 14, 4138. https://doi.org/10.3390/app14104138, https://www.mdpi.com/2076-3417/14/10/4138.